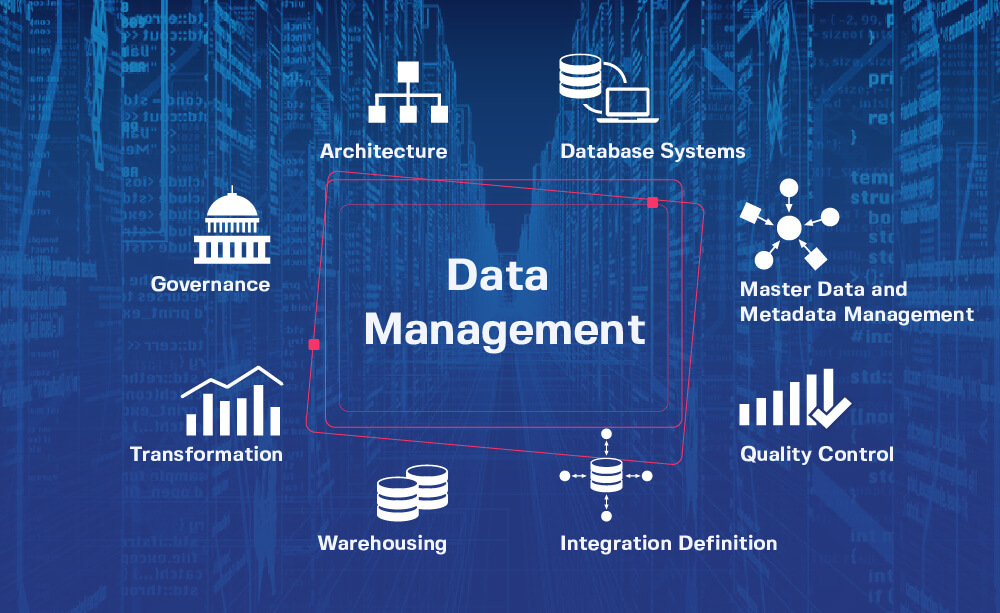
The Impact of Data Management on Business Intelligence and Analytics
The Impact of Data Management on Business Intelligence and Analytics
Businesses generate approximately 2.5 quintillion bytes of data each day, yet a staggering 60% of this data remains underutilized due to poor data management practices. This statistic highlights a critical challenge: despite having vast amounts of data, many organizations struggle to leverage it effectively.
Effective data management is not just a technical necessity but a strategic asset that profoundly influences business intelligence (BI) and analytics. By ensuring data quality, integration, and governance, organizations can unlock actionable insights, drive informed decision-making, and gain a competitive edge. This blog will explore how robust data management practices directly impact BI and analytics, offering a roadmap for businesses to enhance their data strategies and achieve success.
Understanding Data Management
Data Management refers to the process of acquiring, validating, storing, protecting, and processing data to ensure its accessibility, reliability, and timeliness for its users. It encompasses a range of practices and technologies aimed at managing an organization’s data resources effectively.
Key Components of Data Management include:
- Data Storage: Methods and systems for storing data securely and efficiently, such as databases and data warehouses.
- Data Integration: Combining data from different sources to provide a unified view, often using data integration tools and techniques.
- Data Quality: Ensuring that data is accurate, consistent, and reliable.
- Data Governance: Establishing policies, procedures, and standards to manage data effectively and ensure compliance with regulations.
- Data Security: Protecting data from unauthorized access, breaches, and other security threats.
- Data Analytics: Utilizing data for analysis and decision-making, including reporting and advanced analytics.
Importance
Data management is crucial for businesses for several reasons:
- Informed Decision-Making: Accurate and well-managed data provides the foundation for making informed decisions. Without reliable data, decision-making can be based on incomplete or incorrect information, leading to suboptimal outcomes.
- Operational Efficiency: Proper data management streamlines data processes, reduces redundancy, and improves efficiency, allowing organizations to operate more smoothly and effectively.
- Regulatory Compliance: Businesses must comply with various regulations and standards related to data privacy and protection. Effective data management ensures that data practices adhere to legal requirements, reducing the risk of non-compliance and associated penalties.
- Enhanced Data Security: Proper management includes implementing robust security measures to protect sensitive information from breaches and unauthorized access, safeguarding both the organization and its customers.
- Improved Customer Experience: Accurate and well-organized data enables businesses to understand and meet customer needs better, leading to improved customer satisfaction and loyalty.
- Competitive Advantage: Organizations that manage their data effectively can leverage it to gain insights, innovate, and make strategic decisions that provide a competitive edge in the market.
Key Principles
Data Quality
- Definition: Data quality refers to the accuracy, completeness, consistency, and reliability of data.
- Importance: High-quality data is essential for making accurate analyses and decisions. Poor data quality can lead to erroneous conclusions and affect business outcomes.
- Practices: Regular data cleansing, validation, and auditing processes help maintain data quality.
Data Governance
- Definition: Data governance involves the creation and enforcement of policies, procedures, and standards for managing data assets.
- Importance: Effective governance ensures that data is managed consistently, complies with regulations, and meets organizational standards.
- Practices: Establishing data stewardship roles, defining data ownership, and implementing data management policies are key practices in data governance.
Data Integration
- Definition: Data integration is the process of combining data from different sources to provide a unified view.
- Importance: Integration allows organizations to consolidate data from disparate systems, improving accessibility and enabling comprehensive analysis.
- Practices: Utilizing data integration tools and techniques, such as ETL (Extract, Transform, Load) processes and data pipelines, facilitates seamless data integration.
Understanding these core components and principles of data management helps organizations build a strong foundation for their data strategies, ultimately enhancing their ability to leverage data effectively for business intelligence and analytics.
Business Intelligence (BI) and Analytics Overview
Business Intelligence (BI) refers to the technologies, processes, and practices used to collect, analyze, and present business data. The primary purpose of BI is to support decision-making by providing actionable insights from historical and current data. BI systems help organizations understand past performance, monitor key metrics, and generate reports that guide strategic planning.
Analytics, on the other hand, involves the systematic computational analysis of data to uncover patterns, correlations, and trends. The purpose of analytics is to provide deeper insights that can predict future outcomes and optimize business processes. Analytics often uses advanced statistical methods and machine learning techniques to derive insights from data.
Roles in Decision-Making:
- BI: Helps organizations make informed decisions by providing historical and real-time data visualizations, dashboards, and reports. It answers questions about what happened in the past and what is happening now.
- Analytics: Goes beyond reporting to answer complex questions about why something happened and what might happen in the future. It supports predictive and prescriptive decision-making, helping organizations anticipate trends and make proactive adjustments.
BI vs. Analytics
Differences:
- Scope: BI focuses on descriptive analytics, which explains past data and provides a summary of historical performance. Analytics, particularly advanced analytics, encompasses predictive and prescriptive analytics, which look at future possibilities and recommend actions.
- Methods: BI primarily uses data aggregation, visualization, and reporting tools to provide insights. Analytics employs statistical analysis, machine learning algorithms, and data mining techniques to uncover deeper insights and patterns.
- Usage: BI is typically used for operational and tactical decision-making, providing regular updates on business performance. Analytics is used for strategic decision-making, offering deeper insights that drive innovation and long-term planning.
Complementarity:
- BI and Analytics Together: BI and analytics complement each other by providing a comprehensive view of data. BI delivers the “what” and “how” of business performance, while analytics explores the “why” and “what next.” Together, they enable organizations to not only understand their current status but also anticipate future trends and make data-driven decisions.
Emerging Technologies:
- Artificial Intelligence (AI): Enhances both BI and analytics by automating data analysis and providing advanced insights through machine learning algorithms.
- Big Data Technologies: Tools like Apache Hadoop and Apache Spark enable the processing and analysis of large-scale data sets, supporting both BI and advanced analytics.
- Cloud-Based Analytics: Solutions like AWS QuickSight and Google BigQuery offer scalable and flexible analytics capabilities in the cloud, providing real-time insights and reducing the need for on-premises infrastructure.
By leveraging these tools and technologies, organizations can effectively harness the power of BI and analytics to drive informed decision-making and achieve strategic objectives.
The Relationship Between Data Management and BI/Analytics
Data Quality
How High-Quality Data Enhances BI and Analytics Efforts:
High-quality data is the backbone of effective business intelligence and analytics. It ensures that the insights derived are accurate, reliable, and actionable. Here’s how high-quality data contributes:
- Accuracy: High-quality data is accurate and reflects the true state of the business, leading to precise analytics and reliable BI reports.
- Consistency: Data that is consistent across various sources prevents discrepancies and ensures uniformity in analysis.
- Completeness: Complete data provides a full picture, allowing for thorough analysis and comprehensive insights.
- Timeliness: Up-to-date data ensures that analytics and BI efforts reflect the most current information, enabling timely decision-making.
Impact on Decision-Making:
- Strategic Planning: Accurate sales data can help forecast future revenue and market trends, allowing companies to make informed strategic decisions.
- Operational Efficiency: Consistent and reliable operational data helps optimize processes and improve efficiency by identifying bottlenecks and areas for improvement.
- Customer Insights: High-quality customer data enables personalized marketing strategies and better customer service by providing a clear understanding of customer preferences and behaviors.
Case Study: Suppose a company operates in the retail sector and uses BI tools to analyze sales performance. Due to poor data quality, the company faced inaccuracies in its sales reports, leading to misguided inventory decisions. The company can improve its data accuracy by implementing a data quality management program, including regular data cleansing and validation processes. As a result, it can optimize inventory levels, reduce stockouts, and increase sales through better-informed decisions.
Data Integration
Importance of Integrating Data from Various Sources:
Integrating data from diverse sources provides a unified view, enabling more comprehensive analysis and richer insights. This is essential for:
- Holistic Analysis: Combining data from various systems (e.g., sales, marketing, finance) offers a complete view of business performance and facilitates cross-functional analysis.
- Enhanced Reporting: Integrated data allows for more accurate and comprehensive reporting, providing a single source of truth for business metrics.
- Improved Decision-Making: A unified data view supports better decision-making by providing a more accurate and complete picture of the business environment.
Challenges and Solutions:
- Data Silos: Data often resides in separate systems, making integration challenging. Solution: Implement data integration platforms and tools that support seamless data consolidation.
- Inconsistent Data Formats: Different sources may use varying data formats. Solution: Use data transformation tools to standardize data formats during integration.
- Data Quality Issues: Integrating data from multiple sources can exacerbate existing quality issues. Solution: Establish data quality management practices to ensure data accuracy before integration.
Case Study: Suppose a company operates in the healthcare industry and integrates patient data from electronic health records (EHR), laboratory systems, and billing systems. Initially, data from these sources was fragmented, leading to incomplete patient profiles and inefficient care. By implementing a robust data integration solution, the company can achieve a unified patient record system. This integration improves patient care coordination, reduces administrative overhead, and enhances overall operational efficiency.
Data Governance
Role of Data Governance in Ensuring Data Consistency and Reliability:
Data governance involves creating policies, procedures, and standards for managing data. It ensures that data is consistent, reliable, and secure, which is crucial for effective BI and analytics.
- Consistency: Data governance establishes standards for data management, ensuring consistency across different data sources and systems.
- Reliability: By defining data ownership and stewardship roles, data governance ensures that data is maintained and managed correctly, enhancing its reliability.
- Security: Data governance includes measures for data protection, ensuring that sensitive information is secure and compliant with regulations.
Impact on Compliance and Reporting:
- Regulatory Compliance: Data governance ensures that data management practices adhere to legal and regulatory requirements, reducing the risk of non-compliance.
- Accurate Reporting: With established governance practices, organizations can produce accurate and reliable reports, meeting internal and external reporting standards.
Case Study: Suppose a company is a financial services firm that needs to comply with stringent regulations for data privacy and reporting. Implementing a comprehensive data governance framework, including data classification, access controls, and audit trails, can help the company ensure compliance with regulations such as GDPR and SOX. This not only reduces the risk of legal penalties but also improves the accuracy and reliability of its financial reports.
By understanding the interplay between data management, BI, and analytics, organizations can better leverage their data to drive insightful decision-making, operational efficiency, and strategic advantage.
Best Practices for Optimizing Data Management for BI/Analytics
Data Management Strategies
- Establish Clear Objectives: Define the goals and objectives for your data management strategy. Align these goals with your business objectives and BI/analytics needs to ensure that data management efforts support organizational priorities.
- Implement a Data Management Framework: Develop a comprehensive framework that includes data governance, data quality, and data integration. This framework should outline roles, responsibilities, processes, and standards for managing data effectively.
- Invest in Data Infrastructure: Build a robust data infrastructure that includes scalable storage solutions, high-performance data processing systems, and reliable backup mechanisms. Ensure that your infrastructure can handle current and future data volumes.
- Promote Data Literacy: Educate employees on data management best practices, data governance policies, and the importance of data quality. Foster a culture of data-driven decision-making across the organization.
- Regularly Review and Update Data Management Practices: Continuously assess and improve data management practices to adapt to changing business needs, technological advancements, and evolving data regulations.
Data Quality Management
- Implement Data Quality Standards: Define clear standards for data accuracy, completeness, consistency, and timeliness. Establish criteria for evaluating and maintaining data quality.
- Conduct Regular Data Quality Audits: Perform periodic data quality audits to identify and address data issues. Use automated tools to monitor data quality and generate reports on data health.
- Data Cleansing: Regularly cleanse data to correct errors, remove duplicates, and fill in missing values. Implement data validation rules to prevent incorrect data entry.
- Data Enrichment: Enhance data by adding relevant external information or by correcting inaccuracies. Use data enrichment services to improve the quality and value of your data.
- Establish Data Stewardship Roles: Designate data stewards who are responsible for managing data quality within their domains. These individuals should oversee data entry, validation, and maintenance processes.
Integration Techniques
- Use ETL (Extract, Transform, Load) Processes: Implement ETL processes to extract data from various sources, transform it into a consistent format, and load it into a central data repository for analysis.
- Adopt Data Integration Tools: Utilize data integration tools and platforms to automate data consolidation and synchronization across different systems. Look for tools that support real-time data integration and data warehousing.
- Implement Data Virtualization: Use data virtualization techniques to create a unified view of data from disparate sources without physically moving it. This approach allows for real-time data access and reduces integration complexity.
- Standardize Data Formats: Ensure that data from different sources is standardized to avoid inconsistencies. Use data mapping and transformation techniques to align data formats.
- Monitor Data Integration Processes: Regularly monitor data integration processes to ensure they are functioning correctly and efficiently. Address any issues promptly to prevent data discrepancies.
Governance Frameworks
- Define Data Governance Policies: Develop and document policies for data management, including data ownership, data access controls, and data privacy. Ensure that these policies align with regulatory requirements and industry best practices.
- Establish Data Governance Roles: Assign roles such as data stewards, data custodians, and data governance committees to oversee data management practices and ensure compliance with governance policies.
- Implement Data Quality Management: Integrate data quality management practices into your governance framework to ensure data accuracy and reliability. Define procedures for handling data quality issues.
- Ensure Data Security and Privacy: Include measures for protecting data security and privacy within your governance framework. Implement access controls, encryption, and audit trails to safeguard sensitive information.
- Foster a Data-Driven Culture: Promote a culture of data governance across the organization. Encourage adherence to data policies and provide training on data governance principles and practices.
By following these best practices and leveraging the right tools and technologies, organizations can optimize their data management efforts, enhancing their BI and analytics capabilities and driving better business outcomes.
Future Trends and Innovations
Emerging Technologies
Artificial Intelligence (AI) and Machine Learning (ML)
- AI-Driven Data Management: AI and ML are increasingly being used to automate and enhance data management tasks such as data quality monitoring, anomaly detection, and predictive analytics. AI algorithms can learn from historical data to identify patterns and make real-time recommendations, improving decision-making and operational efficiency.
- Predictive Analytics: ML models can analyze historical data to forecast future trends and outcomes, enabling businesses to anticipate market changes and make proactive decisions.
Big Data Technologies
- Real-Time Data Processing: Technologies like Apache Kafka and Apache Flink are enabling real-time data processing and streaming analytics. These tools allow businesses to analyze data as it is generated, providing timely insights and supporting agile decision-making.
- Data Lakes: Data lakes are evolving to support the storage and analysis of large volumes of unstructured and semi-structured data. They provide a flexible and scalable solution for managing diverse data types and integrating them for comprehensive analysis.
Cloud-Based Data Management
- Serverless Computing: Serverless computing platforms, such as AWS Lambda and Google Cloud Functions, are simplifying data management by eliminating the need for server infrastructure management. This approach allows organizations to focus on developing and deploying data solutions with greater scalability and cost efficiency.
- Data-as-a-Service (DaaS): DaaS solutions offer on-demand access to data and analytics services via the cloud, providing businesses with flexible and scalable data management capabilities. This model reduces the need for on-premises infrastructure and supports remote access and collaboration.
Data Privacy and Security Innovations
- Zero Trust Security: The zero-trust security model assumes that threats could be internal or external, requiring strict verification for all access requests. Innovations in this space are improving data security by implementing rigorous access controls and continuous monitoring.
- Privacy-Enhancing Technologies (PETs): PETs, such as differential privacy and homomorphic encryption, are being developed to protect sensitive data while allowing for meaningful analysis. These technologies address privacy concerns and support compliance with data protection regulations.
Data Fabric and Data Mesh
- Data Fabric: A data fabric architecture integrates data across disparate sources and systems, providing a unified view and enabling seamless data management. It leverages metadata and AI to automate data integration and governance tasks.
- Data Mesh: The data mesh concept promotes a decentralized approach to data management, where data is treated as a product and managed by cross-functional teams. This approach aims to improve data scalability and accessibility across the organization.
Predictions
Increased Integration of AI and Automation
- Predictive and Prescriptive Analytics: AI and ML will increasingly drive predictive and prescriptive analytics, allowing businesses to anticipate trends, optimize operations, and make data-driven recommendations with higher accuracy.
- Automation of Data Management Tasks: Routine data management tasks, such as data cleansing, integration, and governance, will become more automated, reducing manual effort and increasing efficiency.
Growth of Real-Time Data Analytics
- Enhanced Real-Time Capabilities: As technologies for real-time data processing continue to advance, businesses will be able to analyze and act on data as it is generated. This will support more agile and responsive decision-making, particularly in fast-paced industries like finance and e-commerce.
Expansion of Cloud-Based Data Solutions
- Cloud-Native Data Platforms: Cloud-native data platforms will become more prevalent, offering scalable and flexible solutions for managing and analyzing data. Organizations will increasingly adopt cloud-based data lakes, data warehouses, and analytics tools to support their data needs.
Evolution of Data Governance and Privacy Practices
- Enhanced Data Governance Frameworks: Data governance practices will evolve to address the growing complexity of data environments and regulatory requirements. Organizations will implement more sophisticated governance frameworks that integrate AI and automation to ensure data quality, security, and compliance.
- Stronger Focus on Privacy: As data privacy concerns continue to rise, there will be a greater emphasis on implementing privacy-enhancing technologies and ensuring compliance with evolving data protection regulations.
Rise of Decentralized Data Architectures
- Adoption of Data Mesh: The concept of data mesh will gain traction, promoting a decentralized approach to data management that empowers teams to manage and utilize data as a product. This will enhance data accessibility and collaboration across the organization.
Integration of Advanced Analytics with IoT
- IoT-Driven Insights: The integration of IoT devices with advanced analytics will enable businesses to gather and analyze data from connected devices, providing valuable insights into operational performance, customer behavior, and market trends.
As data management technologies and practices continue to evolve, businesses that stay ahead of these trends and innovations will be better positioned to leverage their data for strategic advantage and drive informed decision-making.
Conclusion
In this blog, we’ve explored the critical role of data management in enhancing business intelligence (BI) and analytics. We started by defining data management and its importance, delving into its key principles such as data quality, integration, and governance. We then examined how effective data management directly impacts BI and analytics, highlighting the significance of high-quality data, seamless integration, and robust governance frameworks.
We also looked at the latest trends and innovations shaping the future of data management, including the integration of AI and machine learning, advancements in real-time data processing, the rise of cloud-based solutions, and evolving data privacy practices. These developments are set to transform how organizations manage and leverage their data, providing opportunities for more accurate insights and better decision-making.
We encourage you to assess your current data management practices and identify areas for improvement. Consider how optimizing your data management can enhance your BI and analytics capabilities, leading to more informed decisions and greater business success.
If you’re interested in exploring how our data management services can help you achieve these goals, or if you have any questions about improving your BI and analytics capabilities, we invite you to contact us for a consultation. Our team is here to provide expert guidance and support tailored to your specific needs. Explore our services or reach out today to start optimizing your data management strategy.