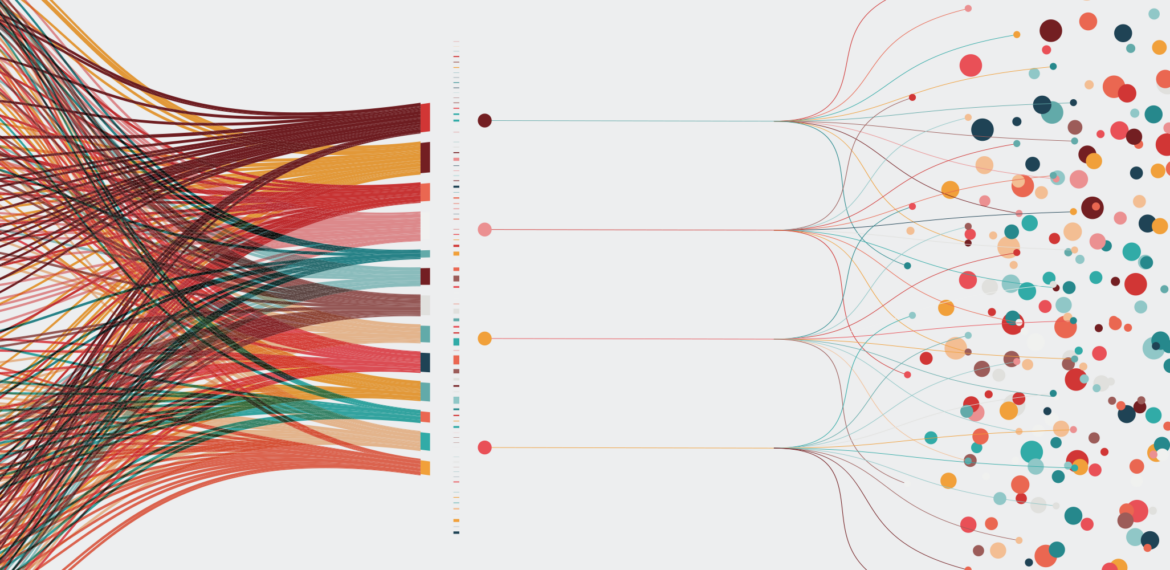
Unstructured Data: Unlocking Insights with AI
Unstructured Data: Unlocking Insights with AI
In today’s data-driven world, organizations are generating information at unprecedented rates. Yet, beneath the structured spreadsheets and relational databases lies a vast and often untapped resource: unstructured data. From customer emails and social media comments to scanned documents, images, videos, and audio recordings—this type of data makes up more than 80% of all enterprise information.
Unlike structured data, which fits neatly into rows and columns, unstructured data lacks a predefined format, making it difficult to store, search, and analyze using traditional methods. For decades, businesses have struggled to extract meaningful insights from this growing digital chaos.
But that’s changing rapidly.
Thanks to advancements in Artificial Intelligence (AI)—particularly in areas like Natural Language Processing (NLP), Computer Vision, and Machine Learning—organizations are now equipped to unlock the hidden potential of unstructured data. These technologies can read, interpret, and derive insights from human language, visuals, sounds, and other non-tabular formats, transforming raw inputs into strategic intelligence.
This blog explores how AI is transforming the way we engage with unstructured data. We’ll examine industry-specific use cases, key technologies, implementation strategies, and the future of AI-driven analytics.
Because the next big opportunity for your business might not be in a dashboard—but buried in a PDF, a customer tweet, or a product image.
The Rise of Unstructured Data in the Digital Age
As businesses digitize at breakneck speed, the volume of data being produced has exploded—not just in quantity, but in variety. Every customer interaction, social media post, voice command, security camera feed, or scanned invoice generates a stream of unstructured data. And this surge shows no signs of slowing down.
Why the Growth?
-
Proliferation of Devices: Smartphones, IoT sensors, wearables, and smart appliances continuously produce audio, video, and textual data.
-
Digital Customer Engagement: Businesses now rely on omnichannel communication—emails, chats, reviews, voice calls—creating vast trails of customer sentiment and behavioral data.
-
Content-First World: From marketing assets to internal documents, digital content is increasingly multimedia and format-diverse.
Industry-Specific Examples
Let’s break it down by industry to see how unstructured data is created daily:
-
Healthcare
-
Clinical notes, diagnostic reports, radiology images, prescriptions
-
EMRs often store large amounts of text-based and image-based records
-
-
Retail & eCommerce
-
Product reviews, social media comments, customer support chat logs
-
User-generated content and visual searches are driving sales strategies
-
-
Banking & Finance
-
Call center recordings, chat transcripts, PDF contracts
-
Important for fraud detection, risk management, and compliance
-
-
Manufacturing
-
Machine sensor logs, maintenance reports, quality control images
-
Valuable for predictive maintenance and operational insights
-
-
Government & Public Sector
-
CCTV footage, citizen feedback forms, scanned documents
-
Supports urban planning, public safety, and policy evaluation
-
The Problem with Traditional Systems
Structured databases and conventional analytics tools are simply not designed to accommodate this diversity. Traditional SQL-based systems rely on defined schemas, making them ill-equipped to handle the variability, volume, and velocity of unstructured inputs.
As a result, valuable information often sits unused, forming what’s known as a “data graveyard”—available, but inaccessible.
The Wake-Up Call
With data growth far outpacing human ability to manually process and interpret it, companies are now turning to AI. AI doesn’t just scale up human analysis—it redefines what’s possible.
Up next: how exactly AI is breaking through the unstructured data barrier.
Challenges of Unstructured Data
Unstructured data holds enormous potential—but unlocking that potential isn’t straightforward. Organizations often encounter serious roadblocks when attempting to extract insights or derive value from it. Understanding these challenges is crucial before diving into AI-driven solutions.
1. No Defined Structure
Unlike structured data, unstructured data lacks a fixed schema or predefined fields. This makes it difficult to:
-
Query with traditional tools
-
Store in relational databases
-
Organize for reporting or analysis
For example, a paragraph in a customer complaint email can contain sentiment, product references, and urgency—but no column or label explicitly tells you where to look.
2. Format Variety
Unstructured data comes in many forms:
-
Text (emails, PDFs, chats, logs)
-
Images (JPEG, PNG, scans)
-
Video (MP4, surveillance footage)
-
Audio (calls, voice notes)
-
Mixed content (social media posts, web pages)
This diversity means that a one-size-fits-all tool or model won’t suffice. Each data type requires specialized techniques for processing and analysis.
3. Volume and Velocity
Massive volumes of unstructured data are generated continuously:
-
Petabytes of video from security systems
-
Millions of tweets per minute
-
Thousands of customer service calls per day
Without scalable infrastructure and automated processing, it’s impossible to keep up.
4. Quality and Noise
Unstructured data is often messy:
-
Incomplete or ambiguous language in documents
-
Poor audio quality or background noise in recordings
-
Blurry images or low-resolution scans
AI systems need to be trained to recognize and handle this “noise” to avoid garbage-in, garbage-out scenarios.
5. Search and Retrieval Difficulty
Structured databases allow precise queries (e.g., “show all orders over $500”). With unstructured data, that’s much harder:
-
How do you search millions of PDFs for a specific clause?
-
How do you find all customer messages mentioning a hidden defect?
AI-powered semantic search is emerging to tackle this—but it’s still complex and evolving.
6. Security, Governance, and Compliance
Unstructured data can contain:
-
Personal identifiable information (PII)
-
Confidential business information
-
Sensitive health or financial data
Managing access, usage rights, retention policies, and regulatory compliance (GDPR, HIPAA, etc.) adds another layer of complexity.
7. Integration into Existing Workflows
Even if insights are extracted, they need to be delivered where they matter—CRM systems, dashboards, or operational tools. Integrating unstructured data processing into BI pipelines and business processes remains a technical and organizational hurdle.
How AI Unlocks Unstructured Data
Artificial Intelligence has revolutionized how we process and analyze data—especially the messy, diverse, and complex world of unstructured information. Unlike traditional tools that require fixed schemas and predefined rules, AI excels at pattern recognition, contextual understanding, and adaptive learning—making it the perfect ally for unlocking the value hidden in unstructured data.
Let’s break down how AI works across different unstructured formats:
1. Natural Language Processing (NLP) – Understanding Text
NLP enables machines to read, understand, and derive meaning from human language. This is critical for analyzing unstructured text like emails, support tickets, reports, reviews, or contracts.
Key AI Capabilities:
-
Entity Recognition: Extracting names, locations, dates, products, etc.
-
Sentiment Analysis: Detecting emotions in customer feedback
-
Topic Modeling: Grouping large volumes of text into themes
-
Summarization: Turning lengthy documents into digestible overviews
-
Translation & Transcription: Converting speech or text across languages
Use Case Example:
A telecom company uses NLP to process customer complaints from chat logs and categorize them by urgency and topic—dramatically reducing resolution time.
2. Computer Vision – Understanding Images and Video
Computer Vision (CV) enables machines to interpret and analyze visual data. It’s essential for industries like manufacturing, healthcare, retail, and security.
Key AI Capabilities:
-
Image Classification: Labeling content in images (e.g., product defects)
-
Object Detection: Locating specific items in photos or video
-
Facial Recognition: Identifying individuals in a crowd
-
OCR (Optical Character Recognition): Extracting text from scanned documents
Use Case Example:
A manufacturing firm uses CV to automatically detect cracks or deformities in parts during the quality assurance process—reducing human error and improving speed.
3. Speech Recognition & Audio Analysis
Voice recordings, podcasts, customer service calls, and video audio are goldmines of insight—if you can understand them.
Key AI Capabilities:
-
Speech-to-Text: Transcribing audio to searchable text
-
Speaker Diarization: Identifying who is speaking in a conversation
-
Emotion Detection: Understanding tone, stress, and sentiment
-
Voice Command Recognition: Powering voice assistants and IVR systems
Use Case Example:
A bank analyzes thousands of daily call center interactions using AI to flag calls with signs of dissatisfaction or compliance violations.
4. Generative AI – Synthesizing New Knowledge
Generative AI models like ChatGPT and Claude go a step further by synthesizing new insights from raw inputs.
Key AI Capabilities:
-
Document Understanding: Summarizing reports, extracting action items
-
Question Answering: Responding to queries using large document sets
-
Text Generation: Creating structured outputs (emails, summaries, analyses) from unstructured prompts
-
Multimodal Processing: Combining text, image, and audio understanding in a single model
Use Case Example:
A legal firm uses a large language model to automatically extract key clauses from lengthy contracts, saving hours of manual review time per document.
Why It Works
AI models are trained on massive datasets to recognize language, image patterns, and audio signals across contexts. With fine-tuning and domain-specific training, they can adapt to your organization’s unique data landscape.
Bonus: These tools scale effortlessly. Whether you’re analyzing 100 or 10 million records, AI doesn’t tire, slow down, or lose accuracy (when implemented right).
AI Tools and Technologies for Unstructured Data
Transforming unstructured data into actionable intelligence requires more than just algorithms—it demands the right combination of AI models, processing pipelines, infrastructure, and integration platforms. Fortunately, the AI ecosystem has matured to offer a broad toolkit that organizations can tailor to their needs.
Let’s explore the key technologies that make unstructured data processing possible:
1. AI & Machine Learning Frameworks
These foundational tools allow data scientists and engineers to build, train, and deploy AI models tailored to specific unstructured data types.
-
TensorFlow / PyTorch: Popular deep learning libraries for custom NLP and Computer Vision models.
-
HuggingFace Transformers: A rich repository of pre-trained NLP models (BERT, RoBERTa, GPT) for tasks like summarization, Q&A, and sentiment analysis.
-
OpenCV: Widely used for image and video processing tasks in computer vision.
Use Case: A healthcare company uses PyTorch with medical image datasets to train a deep learning model that identifies tumors in radiology scans.
2. Cloud-Based AI Services
Cloud providers offer pre-built AI APIs that simplify the integration of unstructured data capabilities—no training required.
-
AWS: Comprehend (text analysis), Rekognition (image/video), Transcribe (speech-to-text)
-
Azure Cognitive Services: Text Analytics, Form Recognizer, Face API, Speech Services
-
Google Cloud AI: Vision AI, Natural Language AI, Video Intelligence
Benefit: Ideal for rapid prototyping, scalable deployments, and pay-as-you-go pricing models.
3. Data Storage & Retrieval
Unstructured data requires flexible, scalable, and cost-efficient storage. These technologies help store, manage, and retrieve large volumes of heterogeneous data.
-
Data Lakes (e.g., Amazon S3, Azure Data Lake, Google Cloud Storage)
Store raw data in its native format (text, audio, image) for later analysis -
NoSQL Databases (e.g., MongoDB, Couchbase)
Suitable for document-oriented, semi-structured, and dynamic data -
Vector Databases (e.g., Pinecone, Weaviate, FAISS)
Enable fast semantic search and similarity comparison—essential for NLP and image AI
Use Case: A retail company uses a vector database to power a product recommendation engine that compares customer reviews and visual tags.
4. ETL/ELT Pipelines for Unstructured Data
Extract, transform, and load (ETL) tools are evolving to support unstructured data ingestion, enrichment, and transformation.
-
Apache NiFi: Flow-based automation for ingesting and processing diverse data formats
-
Airbyte / Fivetran: ELT pipelines that support structured and unstructured connectors
-
Talend: Offers prebuilt components for text processing, OCR, and more
Pro Tip: Combine these with AI inference pipelines to process data at ingestion time.
5. Foundation Models & Large Language Models (LLMs)
LLMs represent a breakthrough in working with unstructured text and mixed media content.
-
OpenAI’s GPT (ChatGPT): Excellent for document summarization, content generation, semantic search
-
Anthropic’s Claude: Known for document analysis, compliance use cases
-
Meta’s LLaMA / Google Gemini / Mistral: Open-source and commercial models for fine-tuning
Emerging Capability: Multimodal LLMs can understand and connect information across images, text, and speech—enabling unified analysis across different data formats.
6. MLOps and Deployment Tools
Once models are trained or integrated, they need to be managed and scaled in production.
-
MLflow / Weights & Biases: For experiment tracking and model management
-
Kubeflow / Vertex AI: For deploying AI pipelines at scale
-
Docker / Kubernetes: For containerized deployment of AI services
Use Case: A logistics firm uses Kubeflow to deploy and monitor a CV model that inspects package quality from real-time camera feeds.
7. Integration with Business Intelligence (BI) & CRM Tools
The insights from unstructured data become truly valuable when integrated into decision-making tools.
-
Power BI, Tableau, Qlik: Can now ingest AI-enriched unstructured data
-
Salesforce, ServiceNow, Dynamics 365: Enhanced with AI insights from customer interactions, documents, and service logs
Putting It All Together
Modern AI stacks allow organizations to:
-
Ingest unstructured data in real-time
-
Process it with domain-specific models
-
Store and index it for search
-
Serve it via APIs, dashboards, and applications
The result? Faster decisions, smarter automation, and deeper insight.
Real-World Use Cases Across Industries
AI-powered unstructured data analytics is no longer a futuristic concept—it’s already transforming operations, decision-making, and customer experiences across industries. By applying NLP, computer vision, audio analysis, and generative AI, organizations are uncovering insights that were previously locked away in emails, images, videos, and voice recordings.
Let’s look at how different sectors are benefiting from these innovations:
A. Healthcare
Healthcare is one of the most data-rich yet data-siloed industries. AI helps bridge the gap between raw clinical data and actionable medical insight.
Use Cases:
-
Radiology Imaging: Computer vision models detect anomalies (tumors, fractures) in X-rays, MRIs, and CT scans.
-
Clinical Notes Analysis: NLP extracts diagnoses, symptoms, and medication history from unstructured physician notes in EMRs.
-
Patient Sentiment Monitoring: Analyzing feedback from patient surveys and call center transcripts to improve care quality.
Impact: Faster diagnoses, reduced clinician burnout, and improved patient outcomes.
B. Finance & Banking
Financial institutions manage vast amounts of textual, audio, and document-based data—from loan applications to call recordings.
Use Cases:
-
Fraud Detection: AI scans transaction memos and audio logs to flag suspicious behavior.
-
Contract Analysis: NLP automates clause extraction and risk detection in legal agreements.
-
Customer Experience: Transcription and analysis of call center conversations to identify service gaps or churn indicators.
Impact: Enhanced compliance, reduced risk exposure, better customer service.
C. Retail & eCommerce
In retail, customer sentiment is gold. Most of that sentiment comes through unstructured channels like reviews, social media, and chat logs.
Use Cases:
-
Review Mining: NLP models extract key themes (pricing, quality, shipping) from product reviews at scale.
-
Visual Search: Customers upload images and AI matches them with similar products.
-
Social Listening: AI tracks brand mentions and sentiment across social platforms in real-time.
Impact: Smarter product design, optimized inventory, and more personalized customer journeys.
D. Manufacturing
Manufacturers are increasingly deploying AI to monitor equipment, enhance quality control, and streamline maintenance.
Use Cases:
-
Defect Detection: Computer vision identifies cracks, misalignments, or color mismatches during production.
-
Maintenance Logs Analysis: NLP processes unstructured reports from field technicians to predict equipment failures.
-
Safety Monitoring: Analyzing CCTV footage for compliance with safety protocols.
Impact: Reduced downtime, higher product quality, and safer work environments.
E. Telecom
Telecom companies generate petabytes of unstructured data from service logs, call recordings, support chats, and network devices.
Use Cases:
-
Churn Prediction: AI analyzes customer complaints and call sentiment to flag at-risk users.
-
Network Incident Reports: NLP extracts patterns from technician logs to predict outages or performance degradation.
-
Smart Assistants: AI bots handle tier-1 queries based on historical chat transcripts and FAQs.
Impact: Improved retention, faster issue resolution, and higher operational efficiency.
F. Legal & Compliance
The legal sector deals with massive volumes of contracts, case files, and regulatory documents.
Use Cases:
-
Contract Review Automation: AI highlights risky clauses and recommends revisions.
-
eDiscovery: NLP identifies relevant documents in litigation quickly and accurately.
-
Regulatory Mapping: AI aligns internal policies with changing external regulations using unstructured data.
Impact: Reduced legal review time, improved compliance, and lowered costs.
G. Government & Public Sector
Governments handle multi-format citizen data—feedback, forms, video feeds, and scanned documents.
Use Cases:
-
Public Safety Monitoring: Analyzing CCTV and body cam footage for criminal activity or traffic violations.
-
Citizen Sentiment Analysis: Mining unstructured survey responses and social media feedback to guide policy.
-
Document Digitization: Using OCR and NLP to transform paper archives into searchable databases.
Impact: Better governance, safer cities, and more responsive public services.
Across all sectors, one pattern is clear: AI turns previously unusable data into a strategic advantage. The key is knowing how to combine the right tools, models, and industry expertise.
Next up: We’ll look at how to build a strategy to operationalize unstructured data analytics in your organization.
Building a Strategy to Leverage Unstructured Data
Unstructured data projects don’t succeed by accident. They require a clear strategy, cross-functional collaboration, and the right mix of technology and governance. Whether you’re just starting out or looking to scale, a structured approach to unstructured data is essential for unlocking its full potential.
Here’s a step-by-step roadmap to help your organization build a sustainable and scalable strategy:
Step 1: Inventory Your Unstructured Data Sources
Begin by identifying where your unstructured data lives. Typical sources include:
-
Emails and customer service logs
-
Product reviews and social media
-
Scanned contracts and PDFs
-
Video surveillance and audio recordings
-
Medical images, field notes, sensor logs
Tip: Involve both IT and business teams to discover data assets across departments.
Step 2: Define Business Objectives
Don’t collect data just for the sake of it. Tie your unstructured data initiative to specific, measurable goals:
-
Improve customer satisfaction scores?
-
Reduce compliance risk?
-
Automate repetitive document reviews?
-
Predict machine failure before it happens?
Outcome: A focused objective will guide tool selection, resource planning, and KPI tracking.
Step 3: Choose the Right Infrastructure
Unstructured data is large, diverse, and fast-moving. Invest in infrastructure that can scale with your needs:
-
Storage: Cloud-based data lakes (e.g., Amazon S3, Azure Data Lake)
-
Processing: Distributed systems (Spark, Kubernetes), GPU-accelerated compute
-
Search & Retrieval: Vector databases for semantic search
Pro Tip: Use hybrid architectures for sensitive data—combine cloud agility with on-prem security.
Step 4: Select or Train AI Models
Choose pre-trained models when you need fast results, and fine-tune custom models when domain knowledge is critical.
-
Use foundation models like GPT, BERT, or Vision Transformers for general tasks
-
Train domain-specific models for specialized applications (e.g., legal clause extraction, medical imaging)
Tools: HuggingFace, PyTorch, TensorFlow, OpenAI APIs, Azure Cognitive Services
Step 5: Build Your AI Pipeline
Develop an automated pipeline to:
-
Ingest raw data
-
Preprocess (cleaning, OCR, noise reduction)
-
Apply AI models
-
Store enriched insights for use in dashboards or workflows
Tip: Leverage orchestration tools like Airflow or Kubeflow for scaling and monitoring.
Step 6: Integrate Insights into Business Workflows
Insights are only valuable if they’re accessible and actionable. Integrate outputs from your AI models into:
-
BI dashboards (Power BI, Tableau)
-
CRM or ERP systems (Salesforce, ServiceNow)
-
Alerting systems or operational dashboards
Example: NLP results from customer complaints fed directly into a product improvement backlog.
Step 7: Ensure Data Governance and Compliance
Unstructured data often contains sensitive or regulated content. Establish strong governance:
-
Data classification policies
-
Access controls and encryption
-
Retention rules and audit logs
-
Regular model validation and monitoring
Frameworks: GDPR, HIPAA, NIST, DAMA-DMBOK
Step 8: Build a Cross-Functional Team
You’ll need a blend of skills:
-
Data scientists to develop models
-
Engineers to build and deploy pipelines
-
Domain experts to interpret results
-
Compliance and legal to oversee risk
Optional: Engage external experts or managed service providers to accelerate delivery.
Step 9: Measure, Iterate, and Scale
Start small, measure impact, and iterate. Key metrics might include:
-
Model accuracy (e.g., F1 score, precision/recall)
-
Time saved on manual tasks
-
Number of insights generated
-
ROI from unstructured data use cases
Once validated, scale your solution across new departments, use cases, or geographies.
Key Metrics for Success
To turn unstructured data into strategic advantage, it’s essential to measure the impact at every step—from extraction accuracy to business value. These metrics not only help you track progress but also validate investments and uncover opportunities for optimization.
Here are the key categories and metrics you should consider:
1. Data Quality & Extraction Accuracy
Before analysis begins, ensure the unstructured data you’re working with is usable and accurate.
-
OCR Accuracy (%): Measures the correctness of text extracted from scanned documents or images.
-
Entity Extraction Accuracy (NLP): Precision and recall when identifying entities like names, dates, products, or issues.
-
Noise Reduction Effectiveness: For audio and image preprocessing, measure how well the system cleans input data for better model interpretation.
Example: A legal department may track how many key clauses were missed or misidentified in AI-driven contract analysis.
2. AI Model Performance
AI models are the engine driving insight extraction. Track their performance with standard machine learning metrics:
-
Precision & Recall: How accurate is the model at identifying the correct patterns?
-
F1 Score: The harmonic mean of precision and recall—especially useful when balancing false positives/negatives.
-
BLEU/ROUGE Scores: For evaluating AI-generated text summaries or translations.
-
Image Classification Accuracy: For vision models detecting defects, signs, or labels.
Benchmark: Set domain-specific performance thresholds. A 95% accuracy may be acceptable for product tagging, but not for medical imaging.
3. Operational Efficiency Gains
One of the biggest benefits of AI for unstructured data is automating time-consuming tasks.
-
Time Saved per Task: Compare manual vs. automated document reviews or transcription.
-
Process Automation Rate (%): Percentage of repetitive workflows now handled by AI.
-
Support Ticket Resolution Time: Reduced through faster insight from chat and email logs.
Example: A call center uses AI transcription to cut down agent after-call work by 40%.
4. Decision-Making Speed and Effectiveness
Insights from unstructured data often feed into dashboards, alerts, or recommendations. Measure how they improve decision-making.
-
Time-to-Insight: How quickly data can be transformed into a usable decision point.
-
Insight Adoption Rate: Percentage of insights actually acted upon by business users.
-
Accuracy of Predictions/Recommendations: Validated against real-world outcomes.
5. Business Impact Metrics
Ultimately, AI projects should contribute to business KPIs. Unstructured data initiatives can impact:
-
Customer Satisfaction (CSAT/NPS): Through improved personalization or faster service
-
Revenue Lift or Cost Savings: From better product recommendations, fraud detection, or process automation
-
Risk Reduction: Through earlier detection of compliance issues or operational failures
-
Churn Rate Reduction: Through proactive insights from customer complaints and behavior
Example: An eCommerce platform using AI to analyze reviews sees a 15% uplift in conversion rates on optimized product pages.
6. Governance & Compliance Metrics
When working with sensitive unstructured data, don’t overlook metrics that ensure trust and accountability:
-
Data Anonymization Coverage: % of data that is de-identified before processing
-
Auditability: Are model decisions traceable and explainable?
-
Model Drift Tracking: Detect if model performance degrades over time
7. Scalability and System Performance
As your initiative grows, monitor how well the infrastructure supports increasing loads.
-
Throughput (records processed/hour)
-
Latency (seconds to process a single item)
-
Cost per Insight: Cloud, compute, and personnel costs vs. insights generated
Common Pitfalls to Avoid
While the benefits of using AI to unlock unstructured data are compelling, many organizations run into roadblocks that limit their success—or worse, cause initiatives to stall entirely. Avoiding these common pitfalls can make the difference between a proof of concept and a production-ready, scalable solution.
1. Starting Without a Clear Business Case
Too many organizations begin by experimenting with cool AI tools instead of identifying a specific problem to solve.
Don’t Do: “Let’s see what we can find in our emails.”
Do: “Can we reduce customer churn by analyzing complaint emails more effectively?”
Tip: Tie every initiative to a business KPI—whether it’s time saved, cost reduced, or customer satisfaction improved.
2. Ignoring Data Readiness
AI is only as good as the data it learns from. Poor-quality, inconsistent, or unannotated data will lead to underwhelming results.
Common Mistakes:
-
Using PDFs with unreadable fonts for OCR
-
Feeding unbalanced training data into models (e.g., too few examples of rare events)
-
Skipping preprocessing for audio or image files
Solution: Invest time in data cleansing, labeling, and normalization.
3. Underestimating Model Training Efforts
Pre-trained models are powerful—but not always accurate in specialized domains like law, medicine, or manufacturing.
Risk: A generic model may misinterpret domain-specific terminology, leading to false positives or misleading results.
Fix: Fine-tune models using your own labeled data, or choose domain-specific alternatives.
4. Overlooking Edge Cases and Bias
Unstructured data is often messy and unpredictable. Models may struggle with:
-
Sarcasm in text
-
Poor lighting in images
-
Accents in voice recordings
Problem: AI might perform well on the majority of cases, but fail spectacularly on the long tail.
Strategy: Test thoroughly with diverse data samples, monitor model performance continuously, and retrain when needed.
5. Failing to Involve Domain Experts
Data scientists and engineers can build powerful models—but interpreting unstructured data often requires deep subject matter expertise.
Example: A sentiment analysis model may flag “sick performance” as negative—unless a product manager explains it’s slang for praise.
Solution: Bring business users into model development and validation cycles.
6. Not Planning for Governance and Compliance
Unstructured data can contain sensitive or regulated content. Mishandling it can lead to fines, legal exposure, or reputational damage.
Watch Out For:
-
Storing PII without encryption
-
Sharing data across departments without proper access controls
-
Processing data across jurisdictions without compliance checks (e.g., GDPR, HIPAA)
Fix: Implement strict governance policies, role-based access, and automated redaction tools.
7. Building Without Thinking About Integration
Extracting insights is only half the job. If those insights don’t make it into daily workflows, they won’t be used.
Problem: AI flags issues in maintenance logs, but the operations team still uses a manual spreadsheet to track work orders.
Solution: Integrate insights directly into business systems (CRMs, ERPs, dashboards).
8. Focusing Too Much on Tech, Not Enough on Value
Cool AI models won’t generate ROI unless they solve real-world problems. Avoid becoming technology-first instead of outcome-first.
Fix: Design your AI program like a product: What pain does it solve? Who are the users? How do we know it’s working?
9. Scaling Too Soon—or Not at All
Some teams try to industrialize AI after one successful use case without testing robustness. Others stay stuck in pilot mode forever.
Approach:
-
Use agile iterations and quick wins to build momentum
-
Validate ROI before scaling across business units
-
Don’t try to boil the ocean—scale strategically
The Future of Unstructured Data and AI
We’re standing at the edge of a data revolution. As organizations increasingly embrace AI to process unstructured data, the line between what is possible and what was previously unimaginable continues to blur. The future isn’t just about extracting insights—it’s about reimagining how we interact with information entirely.
Let’s explore the key trends shaping the next frontier of unstructured data analytics:
1. Rise of Multimodal AI
The future lies in multimodal models—AI systems that understand and reason across multiple data types simultaneously, such as text, images, audio, and video.
-
Chatbots that analyze a support conversation and a product screenshot
-
Surveillance systems that combine visual feeds with audio detection
-
LLMs that read a slide deck, listen to a recorded meeting, and generate strategic recommendations
Impact: More natural and comprehensive understanding of the full context behind complex business scenarios.
2. Generative AI for Decision Support
Beyond analysis, Generative AI is increasingly being used to:
-
Write reports from meeting transcripts
-
Create structured data from unstructured inputs
-
Draft responses to legal documents or customer complaints
-
Simulate outcomes based on past case data
What’s coming: Autonomous agents that analyze incoming data and take predefined actions—without human input.
3. Democratization of AI through No-Code/Low-Code Platforms
Not every business has an army of data scientists. New platforms are emerging that allow business users to build, test, and deploy AI solutions for unstructured data using drag-and-drop interfaces.
-
Train NLP models on customer feedback
-
Automate contract clause detection
-
Build AI workflows in hours, not weeks
Outcome: Faster time-to-value, reduced reliance on scarce technical talent, and empowered business teams.
4. Real-Time Unstructured Data Processing
What was once a batch job is now becoming real-time. AI pipelines are evolving to handle streaming data from:
-
Social media
-
IoT sensors
-
Call centers
-
Security cameras
This enables instant alerts, faster responses, and continuous learning models that adapt as data flows in.
5. Convergence with Knowledge Graphs and Semantic Search
Combining unstructured data with knowledge graphs will create a web of interconnected insights:
-
Legal documents linked to relevant regulations
-
Customer complaints tied to specific products and historical incidents
-
Research papers automatically mapped to patents and clinical trials
Semantic AI will enable systems to understand meaning—not just keywords—making search and discovery far more powerful.
6. Stronger Governance and Ethical AI
As AI touches more sensitive data (health records, surveillance, legal contracts), ethical AI practices and explainability will become non-negotiable.
-
Transparent decision-making processes
-
Explainable AI (XAI) models
-
Federated learning and privacy-preserving techniques
Expect increased regulation and a push toward trustworthy, accountable AI systems.
7. Unstructured Data as a Strategic Asset
Forward-thinking organizations will no longer treat unstructured data as a byproduct—they’ll treat it as core IP.
-
Historical call logs as training data for AI-powered sales reps
-
Maintenance notes used to train self-diagnosing machinery
-
Customer feedback guiding product roadmaps
Key mindset shift: Stop asking “what’s in the data?” and start asking “how can we learn from it—continuously?”
8. Industry-Specific AI Co-Pilots
AI models are becoming verticalized—trained specifically on the language and logic of industries like:
-
Healthcare (clinical terminology, diagnostics)
-
Legal (contracts, case law)
-
Retail (inventory, promotions)
-
Manufacturing (equipment, processes)
These “AI co-pilots” will work alongside employees, suggesting actions, flagging issues, and optimizing operations in real time.
How Datahub Analytics Can Help
At Datahub Analytics, we understand that unstructured data isn’t just a byproduct of digital transformation—it’s a strategic resource waiting to be tapped. Whether you’re trying to extract insights from customer feedback, automate document workflows, or power AI-driven business intelligence, we offer the end-to-end expertise to turn your unstructured data into a competitive advantage.
Here’s how we can help:
🔍 AI-Powered Unstructured Data Solutions
We design and implement AI workflows tailored to your industry and data types, including:
-
Natural Language Processing (NLP) for text mining, sentiment analysis, and summarization
-
Computer Vision for defect detection, image classification, and OCR
-
Speech and Audio Analysis for transcription, compliance, and voice-based insights
-
Generative AI applications for document understanding and knowledge extraction
🏗️ Scalable Data Infrastructure
We help you build the foundation needed to manage, store, and process large-scale unstructured data:
-
Cloud-based Data Lakes and hybrid storage architectures
-
Integration of vector databases for advanced semantic search
-
Real-time data pipelines using tools like Apache NiFi, Airflow, or Databricks
🤖 Intelligent Automation & RPA
Accelerate workflows and reduce manual effort with AI-infused automation:
-
Automated document classification, routing, and extraction
-
Intelligent bots that respond to emails, analyze forms, and flag anomalies
-
Seamless integration into your CRM, ERP, or service platforms
🔐 Governance, Security & Compliance
We implement robust controls to ensure your unstructured data initiatives are compliant and secure:
-
Data anonymization and redaction
-
Role-based access and encryption
-
Support for GDPR, HIPAA, and industry-specific regulations
🧠 Domain-Specific Expertise
Our team brings deep knowledge across sectors like:
-
Healthcare: EMRs, medical imaging, diagnostics
-
Finance: Call logs, regulatory documents, contracts
-
Retail: Customer feedback, visual merchandising, social data
-
Manufacturing: Quality control imagery, technician logs
📈 Managed Services & Staff Augmentation
Whether you need to augment your internal team or outsource your analytics function, we offer:
-
AI/ML engineers and data scientists on-demand
-
Managed analytics platforms and support
-
Ongoing optimization and model lifecycle management
🎯 End-to-End Delivery
From strategy to deployment, we guide you through:
-
Use case identification and ROI modeling
-
Proof of concept and model validation
-
Scalable production rollout
-
Post-deployment monitoring and support
📩 Ready to unlock hidden insights from your unstructured data?
Let’s talk. Contact Datahub Analytics to schedule a discovery session.