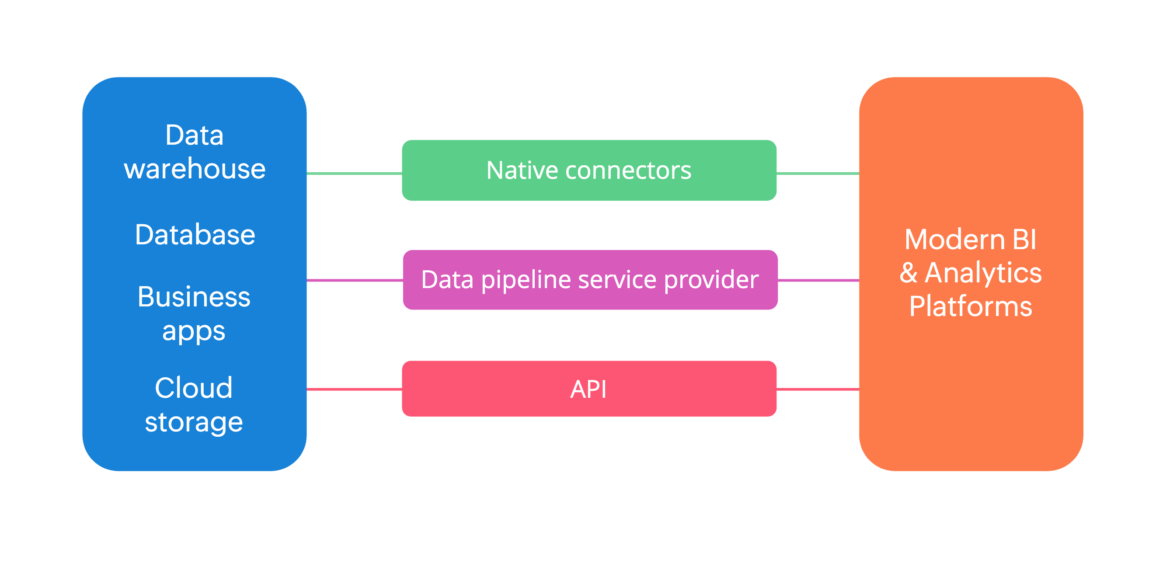
The Rise of Automated Data Analytics Platforms
The Rise of Automated Data Analytics Platforms
By 2027, the global market for automated data analytics platforms is projected to exceed $20 billion, driven by an insatiable demand for faster and more reliable insights in business decision-making.
Traditional data analytics processes often rely on manual data preparation, siloed systems, and resource-heavy operations. These limitations lead to delays in actionable insights, errors in analysis, and missed opportunities for organizations to stay ahead in a competitive landscape.
Automated data analytics platforms are revolutionizing the way businesses approach data. By leveraging cutting-edge technologies like artificial intelligence and machine learning, these platforms enable faster, more accurate insights at scale, empowering organizations to make smarter decisions and drive innovation.
The Need for Automation in Data Analytics
Challenge Overview:
Traditional data analytics processes are fraught with inefficiencies and limitations that hinder organizations from fully harnessing the power of their data:
- Time-Consuming Data Preparation:
Cleaning, organizing, and preparing data for analysis can consume up to 80% of a data analyst’s time. This slows down the delivery of actionable insights, making it difficult for businesses to respond to market changes swiftly. - Human Error in Analysis:
Manual processes often lead to inaccuracies, from mislabeling data to overlooking key patterns. These errors compromise the reliability of insights and can result in misguided business decisions. - The Growing Complexity of Datasets:
As businesses generate and integrate data from multiple sources—social media, IoT devices, CRM systems, and more—the sheer volume and diversity of datasets can overwhelm traditional analytics tools and teams.
Business Impact:
These challenges directly impact an organization’s ability to remain competitive in a data-driven economy:
- Delayed Decision-Making: Slow analytics processes mean missed opportunities, particularly in fast-moving industries like retail or finance.
- Increased Costs: Inefficient workflows and the need for large data teams drive up operational costs.
- Loss of Competitive Edge: Organizations unable to extract timely and accurate insights struggle to adapt to market trends, losing out to competitors leveraging automation for agility.
Addressing these pain points has made automation not just a convenience but a necessity in modern data analytics, paving the way for platforms designed to streamline and enhance every step of the data lifecycle.
What Are Automated Data Analytics Platforms?
Definition:
Automated data analytics platforms are software solutions designed to streamline the entire data analysis process by automating data ingestion, preparation, analysis, and visualization. These platforms leverage advanced technologies to remove manual intervention and enable organizations to extract valuable insights in real-time, faster and more accurately. Key features of automated data analytics platforms include:
- Automated Data Ingestion and Preparation:
These platforms can automatically collect data from multiple sources, cleanse it, and transform it into a structured format for analysis. This removes the time-consuming and error-prone process of manual data wrangling. - AI-Powered Analytics and Visualization:
Using machine learning (ML) algorithms, these platforms can identify patterns, trends, and anomalies in large datasets. Advanced visualization tools then present insights in easy-to-understand formats, allowing decision-makers to quickly grasp key findings and take action. - Predictive Insights and Decision Support:
By leveraging predictive analytics, these platforms can forecast future trends, helping businesses anticipate opportunities or risks. Decision support systems within the platform guide users in making data-driven decisions based on real-time insights.
Key Technologies:
Automated data analytics platforms rely on a variety of cutting-edge technologies to drive their capabilities, including:
- Artificial Intelligence (AI):
AI models enable the automation of complex analytical tasks, such as natural language processing (NLP) for understanding text data, image recognition for visual data, and AI-based recommendation engines. - Machine Learning (ML):
ML algorithms power the predictive capabilities of these platforms, learning from historical data to make accurate forecasts and optimize processes. - Natural Language Processing (NLP):
NLP allows platforms to analyze unstructured data, like customer reviews or social media posts, by understanding human language, making it easier to extract sentiment and context from large volumes of text.
Together, these technologies make automated data analytics platforms not only more efficient but also more intelligent, enabling businesses to make data-driven decisions at the speed of thought.
Key Benefits of Automated Data Analytics Platforms
- Faster Time-to-Insight:
Automation accelerates the data analysis process by eliminating time-consuming manual tasks like data cleaning, integration, and transformation. With automated workflows, organizations can obtain insights in real-time or within minutes, rather than days or weeks. This speed enables businesses to make quick decisions and respond to market trends with agility. - Improved Accuracy:
Human errors are inevitable in manual data processing, from data entry mistakes to inconsistencies in analysis. Automated platforms, powered by machine learning algorithms, minimize these errors by applying consistent, repeatable processes. Additionally, AI models can enhance the accuracy of insights by identifying hidden patterns and anomalies in complex datasets, ensuring that decisions are based on high-quality data. - Scalability:
As businesses grow, so does their data. Automated data analytics platforms are designed to scale effortlessly, capable of handling vast volumes of structured and unstructured data from diverse sources. Whether data grows horizontally (increased sources) or vertically (increased data points), these platforms ensure performance remains steady, enabling businesses to maintain efficiency even as their analytics needs expand. - Cost-Effectiveness:
Automation significantly reduces the reliance on large, specialized in-house teams. By streamlining processes like data preparation, analysis, and reporting, businesses can achieve the same (or better) results with fewer resources. This leads to cost savings in both labor and infrastructure, making automated data analytics platforms a more affordable option compared to traditional methods. Additionally, businesses can repurpose resources for strategic initiatives rather than spending them on repetitive tasks.
Together, these benefits make automated data analytics platforms not just a technological upgrade but a strategic investment that drives business growth, improves operational efficiency, and reduces costs.
Challenges and Considerations
- Data Privacy and Security:
As businesses increasingly rely on automated data analytics platforms, one of the most pressing concerns is data privacy and security. These platforms handle vast amounts of sensitive data, making them potential targets for cyberattacks. Organizations must ensure that their platforms comply with data protection regulations (such as GDPR or CCPA) and implement robust security measures like encryption, secure access controls, and regular audits. Failure to address these concerns could lead to data breaches, legal repercussions, and damage to a company’s reputation. - Integration Issues:
While automated platforms promise efficiency, integrating them with existing data systems and infrastructure can be challenging. Businesses often operate with legacy systems that house critical data, and seamless integration is necessary for smooth data flow between platforms. Disjointed systems can lead to data silos, inconsistencies, and inefficiencies, undermining the value of automation. To overcome these challenges, organizations need to invest in platforms that offer compatibility with their current IT environment or work with experts to ensure proper integration and data synchronization. - Skill Requirements:
While automation handles much of the heavy lifting in data analysis, the need for skilled personnel remains crucial. Automated platforms provide insights, but it takes experienced professionals to interpret these insights effectively and apply them to business strategies. Organizations need employees with expertise in data analytics, data science, and business intelligence to ensure that the automated insights are aligned with organizational goals. Without these skills, there is a risk that automated insights could be misunderstood or misapplied, reducing the overall impact of automation.
Addressing these challenges requires a balanced approach that includes robust data governance, careful platform selection, and investment in talent development to fully capitalize on the benefits of automated data analytics platforms.
The Future of Automated Data Analytics
- Emerging Trends:
As automated data analytics platforms continue to evolve, several key advancements are shaping the future of the industry:- Real-Time Analytics:
The demand for real-time insights is growing as businesses seek to make decisions faster and more accurately. Future platforms will increasingly focus on real-time data processing, enabling businesses to act on insights as they emerge. Whether in retail, finance, or manufacturing, real-time analytics will help organizations stay agile, responding instantly to market changes, customer behavior, or operational issues. - Conversational AI:
Conversational AI, including chatbots and virtual assistants, is set to play a bigger role in data analytics. By allowing users to interact with data platforms via natural language, businesses can democratize data access. Users—from executives to frontline employees—will be able to ask questions in plain language and receive automated insights, removing the need for specialized knowledge in data analysis. - Deeper AI Integration:
AI will continue to evolve, with more sophisticated algorithms that not only automate basic tasks but also provide more advanced insights. In the future, AI will be able to anticipate trends, generate actionable recommendations, and offer predictive analytics with even greater accuracy. This deeper integration will make platforms more proactive, allowing businesses to make decisions before issues arise or opportunities are missed.
- Real-Time Analytics:
- Market Growth:
The market for automated data analytics platforms is experiencing rapid growth. According to recent industry reports, the global market is projected to grow at a compound annual growth rate (CAGR) of over 30% from 2023 to 2027, driven by increasing demand for data-driven decision-making and the need for automation in organizations of all sizes.- Increased Adoption Across Industries:
Initially, larger enterprises were the primary adopters of automated data analytics, but smaller businesses are now catching up as the platforms become more affordable and user-friendly. Sectors such as retail, healthcare, finance, and manufacturing are leading the charge, but even industries like agriculture, energy, and government are beginning to leverage automation for improved efficiency and insights. - Vendor Expansion:
As the market expands, new vendors are entering the space, offering specialized solutions tailored to different industry needs. This growing ecosystem is driving innovation, with businesses having more options than ever when selecting the right platform for their requirements.
- Increased Adoption Across Industries:
The future of automated data analytics is bright, with continuous innovation poised to enhance decision-making, drive efficiency, and unlock new business opportunities. As these technologies become more advanced and accessible, organizations across the globe will increasingly rely on automation to stay competitive in an increasingly data-driven world.
Choosing the Right Technology Partner for Implementing Automated Data Analytics
Selecting the right technology partner is a crucial step in successfully implementing automated data analytics platforms. The right partner can not only provide the tools you need but also offer the expertise and guidance necessary to integrate and scale your solution effectively. Here are key factors to consider when choosing a technology partner:
- Experience and Expertise:
Look for a partner with a proven track record in implementing automated data analytics solutions across various industries. A partner with deep expertise in data science, machine learning, and AI will be able to help you navigate the complexities of advanced analytics and customize solutions that align with your business goals. - Customization and Flexibility:
Every business is unique, and so are its data needs. A technology partner should be able to provide a solution that is customizable and scalable to your organization’s specific requirements. Whether you’re dealing with complex datasets or need integration with existing systems, the partner should offer flexibility to accommodate your evolving needs. - Integration Capabilities:
Ensure the partner’s platform can seamlessly integrate with your existing infrastructure, including data sources, ERP systems, CRMs, and other software tools you currently use. An ideal technology partner will help ensure a smooth transition and ensure that data flows freely across all systems, eliminating silos and inefficiencies. - Support and Training:
Beyond just providing the technology, your partner should offer comprehensive support and training. This ensures that your team is well-equipped to use the platform effectively, extract insights, and take full advantage of automation. Look for partners who offer ongoing maintenance and technical support to address any issues that arise post-implementation. - Security and Compliance:
Data privacy and security should be a top priority when selecting a technology partner. Make sure they follow industry best practices for data protection and comply with relevant regulations such as GDPR or CCPA. A partner with robust security protocols ensures that your sensitive data remains protected as you scale your analytics capabilities. - Cost-Effectiveness:
The right partner should provide a solution that aligns with your budget while delivering long-term value. While cutting-edge solutions may come with higher upfront costs, a good partner will help you understand the return on investment (ROI) and identify areas for cost optimization. - Innovation and Future-Proofing:
Choose a partner who is committed to innovation and staying ahead of the curve with the latest advancements in automated data analytics. This will ensure your platform remains relevant as new technologies, such as AI and machine learning, evolve and can be seamlessly integrated into your systems over time.
At DataHub Analytics, we pride ourselves on being the trusted partner for businesses looking to leverage automated data analytics to drive growth and innovation. Our team of experts will work with you every step of the way, from initial consultation to implementation and ongoing support, ensuring that your data analytics journey is smooth, scalable, and future-proof.
Conclusion
The rise of automated data analytics platforms marks a significant shift in how businesses approach data-driven decision-making. By reducing manual efforts, enhancing accuracy, and scaling efficiently, these platforms empower organizations to unlock the full potential of their data in ways that were once unimaginable. As technology advances, real-time analytics, conversational AI, and deeper AI integration will further transform the landscape, offering unprecedented opportunities for businesses to stay competitive and make smarter, faster decisions.
At DataHub Analytics, we are at the forefront of helping businesses harness the power of automated data analytics. Whether you’re looking to streamline your data processes, gain deeper insights, or integrate advanced technologies into your operations, our team is ready to guide you on the journey to data-driven success.