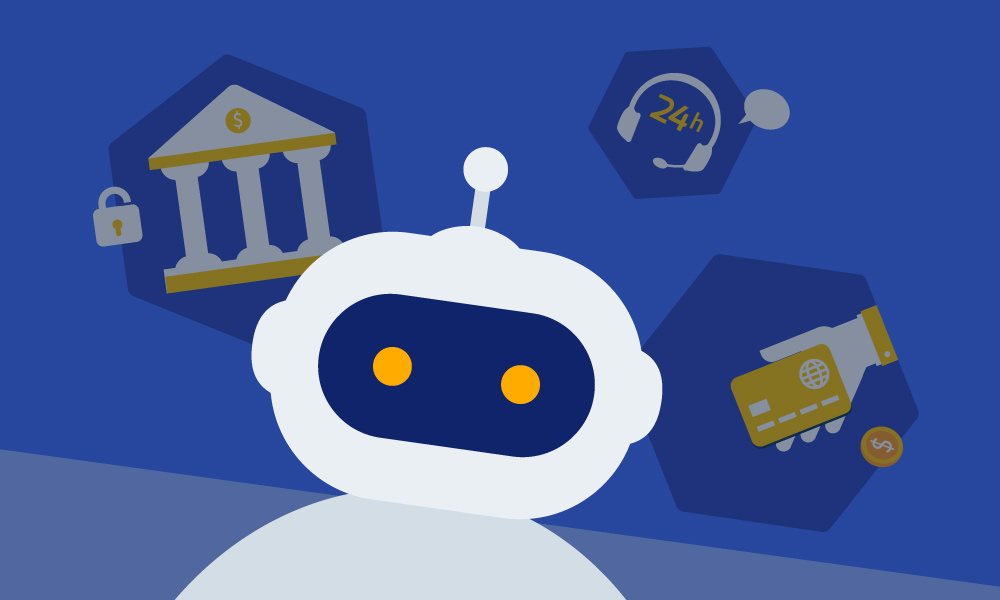
AI and Banking: Predicting Market Trends and Optimizing Investments
AI and Banking: Predicting Market Trends and Optimizing Investments
Artificial Intelligence (AI) is transforming the banking sector, offering new levels of precision, efficiency, and insight, particularly in predicting market trends and optimizing investments. In an increasingly complex and volatile financial landscape, banks and financial institutions are turning to AI to stay competitive, leveraging its advanced data processing capabilities to make faster, more informed decisions.
With vast amounts of data generated every day, traditional methods of analysis can no longer keep pace with the speed and accuracy required for optimal market forecasting. AI’s ability to analyze large datasets, identify patterns, and adapt to changing conditions in real time is providing a significant edge in predicting market movements. As the financial industry becomes more reliant on data-driven decision-making, the need for smarter, more strategic investment approaches is more crucial than ever. Banks that embrace AI technologies are well-positioned to not only improve their investment performance but also mitigate risks in an unpredictable market.
The Role of AI in Modern Banking
Transformation of Traditional Banking Practices
Artificial Intelligence is fundamentally reshaping traditional banking practices by automating processes and enhancing the efficiency of operations across various functions. One of the most visible applications of AI is in customer service, where AI-powered chatbots handle routine inquiries, resolve common issues, and provide round-the-clock assistance. This not only improves customer satisfaction but also frees up human resources for more complex tasks.
Beyond customer-facing roles, AI is revolutionizing back-office operations by automating labor-intensive processes such as data entry, compliance checks, and document verification. By doing so, banks can significantly reduce operational costs and improve the speed of transactions and services.
AI’s ability to handle vast amounts of data in real time is one of its most transformative capabilities. Traditional banking systems, often bogged down by manual processes and limited computing power, struggle with analyzing large datasets quickly enough to capitalize on market opportunities. AI, on the other hand, processes data efficiently, performs complex calculations, and makes real-time predictions with far greater accuracy. This is helping banks streamline everything from loan approvals to risk assessments, allowing for more informed decision-making and precise financial forecasting.
AI’s Integration into Financial Services
AI’s integration into core financial services has been swift and impactful, particularly in areas like fraud detection, credit scoring, and customer service. AI-powered algorithms can detect unusual patterns in transactions, flagging potentially fraudulent activity before it escalates. This ability to identify and mitigate risks in real time is essential for maintaining security in today’s digital banking environment.
Similarly, AI has revolutionized the credit scoring process by analyzing non-traditional data points such as social media activity and spending behaviors, offering a more nuanced and accurate picture of a customer’s creditworthiness. Automated customer service solutions powered by AI have also streamlined service delivery, responding to customer inquiries instantly and resolving issues without the need for human intervention.
However, where AI’s true potential lies—and where it is fast becoming a game-changer—is in market prediction and investment optimization. By analyzing historical market data, identifying trends, and factoring in economic indicators, AI systems can predict market movements with remarkable accuracy. This level of predictive capability allows banks and financial institutions to optimize their investment strategies, manage risk more effectively, and even anticipate future market conditions, providing a significant advantage in the fast-moving world of finance.
How AI Predicts Market Trends
Analyzing Big Data
AI-powered algorithms are revolutionizing the way financial markets are analyzed by processing vast amounts of historical data, market indicators, and economic variables. Traditional methods of analyzing market trends often struggle with the complexity and sheer volume of available data, but AI algorithms can swiftly sift through years of financial records, global economic reports, and industry-specific metrics to identify relevant patterns.
Techniques like machine learning (ML) and deep learning enable AI to detect both subtle and obvious trends in financial markets. Machine learning models are trained to recognize patterns within large datasets and continuously improve their predictions based on new data. Deep learning, which uses artificial neural networks to simulate human brain activity, allows AI systems to discover hidden relationships between seemingly unrelated variables, making it possible to predict market fluctuations with greater precision. These advanced techniques help AI spot trends and anomalies that human analysts may miss, giving financial institutions a more detailed and accurate understanding of market dynamics.
Real-time Analysis and Adaptation
One of the standout advantages of AI in market prediction is its ability to process and analyze real-time data, delivering up-to-the-minute forecasts. Financial markets are highly dynamic, where conditions can change within seconds, making timely adjustments crucial for success. AI can monitor and analyze a continuous stream of live data—such as stock prices, trading volumes, and currency fluctuations—allowing investors and banks to adapt their strategies instantaneously.
By evaluating real-time events, AI models can detect market shifts as they happen, flagging emerging trends and potential risks before they become fully apparent. This capacity for real-time adaptation means that financial institutions can recalibrate their strategies on the fly, executing trades, reallocating assets, or adjusting portfolios to capitalize on new opportunities or avoid potential losses.
AI-Powered Sentiment Analysis
In addition to quantitative data, AI can assess qualitative data through sentiment analysis. Using natural language processing (NLP), AI systems can evaluate the tone and sentiment of news articles, social media posts, company reports, and public statements to gauge public perception of the market.
This kind of sentiment analysis helps predict how events like political developments, corporate scandals, or economic news will influence market behavior. For example, a sudden shift in social media sentiment regarding a particular company can signal changes in its stock performance, allowing investors to make proactive adjustments. By continuously analyzing the sentiment across various sources, AI can incorporate public perception into its predictive models, providing a more comprehensive view of the factors that drive market trends.
Predictive Models
AI utilizes a range of predictive models to forecast market movements and identify potential investment opportunities. These models include:
- Regression Analysis: A statistical method used to model the relationship between a dependent variable (such as stock price) and one or more independent variables (such as economic growth rates or inflation). AI enhances traditional regression analysis by accounting for more variables and identifying nonlinear relationships that are difficult for human analysts to detect.
- Decision Trees: AI decision trees break down data into branches to make sequential decisions based on various market conditions. These trees help identify paths leading to different financial outcomes, enabling investors to predict the likelihood of market movements under different scenarios.
- Neural Networks: AI-driven neural networks, inspired by the human brain, process complex data inputs to identify patterns that traditional models might overlook. These networks can be trained to predict price movements or forecast economic cycles by learning from past data and adjusting their predictions as new data becomes available.
By combining these predictive models, AI delivers a robust, multi-faceted approach to forecasting market trends, helping investors make more informed and strategic decisions in an ever-evolving financial landscape.
Optimizing Investment Strategies with AI
AI-Driven Portfolio Management
AI algorithms are revolutionizing portfolio management by designing and managing diversified portfolios that aim to balance risk and return. Traditional portfolio management relies heavily on human analysts to monitor market trends and adjust asset allocations, but AI takes this a step further by continuously analyzing market data and financial indicators at a scale and speed beyond human capability. AI-powered systems can evaluate a vast array of variables—such as stock performance, interest rates, market volatility, and economic trends—to identify optimal investment strategies.
One of AI’s key advantages in portfolio management is its ability to automatically rebalance portfolios based on real-time market conditions. When market trends shift or an asset’s risk profile changes, AI algorithms can adjust the portfolio by buying or selling assets to maintain a predetermined balance between risk and reward. This automated rebalancing ensures that portfolios remain aligned with investment goals, maximizing returns while minimizing potential losses. With the continuous adjustments made by AI, investors can avoid the delays and human errors that often arise with manual portfolio management.
Risk Management
Managing risk is a critical component of investment strategy, and AI is transforming how risks are identified and mitigated. AI’s ability to process real-time data allows it to detect early warning signs of market instability, enabling investors to take preemptive action. By analyzing past performance data, economic indicators, and market sentiment, AI systems can identify investment risks that may be overlooked by traditional risk assessment methods.
AI enhances risk management through predictive risk assessment, a forward-looking approach that uses AI to anticipate potential risks before they fully materialize. By simulating different market conditions and stress-testing portfolios, AI can forecast how investments might perform in various scenarios—such as economic recessions, interest rate changes, or political instability. Based on these predictions, AI can recommend adjustments to minimize exposure to high-risk assets and prevent significant losses during market downturns. This real-time, predictive approach allows investors to stay ahead of risks and optimize their strategies for long-term stability.
AI-Powered Robo-Advisors
Robo-advisors, powered by AI, have emerged as a cost-effective and efficient solution for personalized investment management. These AI-based platforms use algorithms to analyze an individual’s financial situation, risk tolerance, and investment goals to create customized portfolios. Robo-advisors automatically monitor and adjust these portfolios, ensuring they remain aligned with the investor’s objectives.
For individuals and institutions alike, robo-advisors offer several advantages. They lower the barrier to entry for investing by providing affordable financial advice, eliminating the need for costly human advisors. Additionally, robo-advisors operate 24/7, constantly analyzing market conditions and making adjustments to portfolios in real time. This hands-off, automated approach is especially appealing to investors who want to benefit from sophisticated AI-driven investment strategies without the complexities of active management.
Algorithmic Trading
One of the most advanced applications of AI in investment optimization is algorithmic trading, particularly in high-frequency trading (HFT) strategies. AI optimizes these strategies by executing trades at lightning-fast speeds, often within milliseconds, based on pre-programmed algorithms that respond to real-time market data.
AI-driven algorithmic trading systems scan multiple data sources, such as stock prices, market sentiment, and trading volumes, to make instantaneous decisions about when to buy or sell assets. These algorithms are designed to capitalize on fleeting market opportunities that human traders could easily miss. By reacting to market changes in real time, AI ensures that trades are executed at optimal moments, potentially generating significant profits.
AI’s ability to process vast amounts of data in real time also enables it to detect and respond to micro-trends within the market—small but rapid price fluctuations that may only last for a fraction of a second. By leveraging these micro-trends, AI-driven trading systems can execute thousands of trades in a single day, optimizing returns while minimizing risks. This level of precision and speed makes AI-powered algorithmic trading a powerful tool for both individual and institutional investors looking to maximize their investment strategies.
Benefits of AI in Market Prediction and Investment Optimization
Increased Accuracy and Efficiency
AI significantly enhances the accuracy of market predictions by analyzing large volumes of data with a level of detail that human analysts often cannot match. AI-powered algorithms are capable of identifying intricate patterns, trends, and correlations within historical and real-time data that would otherwise go unnoticed. This improved precision enables financial institutions to make better-informed predictions about market movements, enhancing the effectiveness of their investment strategies.
Another key benefit is the reduction of human error in decision-making. AI systems operate based on data and programmed logic, removing biases, emotional influence, or subjective judgment from investment and portfolio management decisions. This helps banks and investors avoid costly mistakes that are often caused by human oversight, making investment strategies more reliable and consistent over time.
Faster Decision-Making
One of the most transformative advantages AI brings to market prediction and investment optimization is speed. AI processes and analyzes data much faster than traditional methods, enabling quicker decision-making in response to market fluctuations. In today’s fast-moving financial markets, being able to react in real time is critical to capturing short-term opportunities and avoiding losses.
AI systems are continuously monitoring and evaluating data from various sources, making it possible for financial institutions to make rapid adjustments to their strategies. Whether it’s rebalancing portfolios, executing trades, or responding to shifts in market sentiment, AI allows banks and investors to act quickly and efficiently, providing a competitive edge in an environment where timing is often everything.
Cost-Effectiveness
AI-powered investment tools offer significant cost savings by automating many of the processes that were traditionally handled by financial analysts or advisory firms. These tools can manage data analysis, portfolio rebalancing, and even client advisory services without the need for a large, expensive team of human advisors.
For example, robo-advisors provide personalized investment advice at a fraction of the cost of hiring a financial advisor, making high-quality financial management accessible to a wider range of clients. Additionally, AI-driven systems reduce the costs associated with manual data analysis, freeing up resources for financial institutions to allocate elsewhere. In the long term, AI’s efficiency and automation reduce operational expenses while improving the overall quality of financial services.
Scalability
AI’s scalability is another major benefit, allowing it to handle growing datasets and increasingly complex markets as financial institutions expand. Traditional financial models may struggle to cope with large volumes of data or the intricacies of global markets, but AI systems are designed to thrive in these conditions. Whether a bank is managing millions of transactions or monitoring global economic indicators, AI can scale its operations without a drop in performance.
This scalability makes AI suitable for banks and financial institutions of all sizes, from small investment firms to large multinational banks. As data sources and financial markets grow in complexity, AI can continue to adapt, process more data, and deliver increasingly accurate insights, ensuring that investment strategies remain optimized at every level of scale.
Challenges of Implementing AI in Banking
Data Privacy and Security
One of the primary concerns in implementing AI in banking is ensuring the secure handling of sensitive financial data. Banks deal with vast amounts of highly confidential information, from personal client details to transaction records. AI systems, by their nature, process and analyze this data to deliver insights, but this opens up potential vulnerabilities. AI systems can become targets for cyberattacks, where malicious actors attempt to breach systems to access or manipulate sensitive financial information.
Additionally, data privacy regulations, such as the General Data Protection Regulation (GDPR) in Europe, require financial institutions to follow strict protocols when collecting, storing, and processing personal data. Ensuring that AI systems meet these regulations while maintaining high levels of security is a significant challenge for banks. Implementing robust cybersecurity measures and encryption technologies is critical to safeguarding AI-powered systems from breaches and protecting client data.
Regulatory Compliance
Another challenge in integrating AI into banking operations is ensuring compliance with complex financial regulations. AI systems must adhere to various rules, such as anti-money laundering (AML), know-your-customer (KYC) requirements, and transaction monitoring laws. These regulations require financial institutions to conduct due diligence, verify identities, and monitor transactions to prevent fraudulent activities.
AI must be able to interpret and apply these regulations effectively, ensuring that its decision-making processes align with legal requirements. Given the complexity of global financial regulations, designing AI algorithms that consistently comply with these rules can be difficult. Furthermore, regulators are often concerned about the opacity of AI systems—sometimes referred to as the “black box” problem—where the decision-making process is not easily explainable, raising questions about accountability and transparency.
Ethical Considerations
The ethical implications of AI in banking, particularly regarding biases in decision-making, pose another significant challenge. AI algorithms are trained on historical data, and if this data contains biases, such biases can be perpetuated in the AI’s decision-making processes. For example, AI systems used for loan approvals or investment risk assessments may unintentionally favor or disadvantage certain demographic groups based on past trends.
Addressing algorithmic bias is crucial, as biased decisions can lead to unfair outcomes for customers and damage a bank’s reputation. Ethical concerns also extend to transparency—customers may not understand how AI systems arrive at decisions, leading to confusion or mistrust. Financial institutions must ensure that their AI systems are free from discrimination and maintain transparency by providing clear explanations for decisions, especially in areas like loan approvals and credit scoring.
Integration with Legacy Systems
One of the most practical challenges of implementing AI in banking is integrating it with legacy systems. Many banks still rely on outdated infrastructure that was not designed to handle the advanced computing needs of AI. These traditional systems may struggle to support AI algorithms, leading to inefficiencies or technical issues during implementation.
Upgrading legacy systems to seamlessly incorporate AI can be a costly and time-consuming process. Banks must invest in modernizing their infrastructure, ensuring that AI tools are compatible with existing databases, software, and security protocols. Additionally, ensuring that employees are trained to work with AI-powered systems is an important factor in achieving successful integration. Financial institutions must balance the costs of upgrading their systems with the long-term benefits AI can deliver.
Future Trends in AI for Banking and Investment Optimization
Advancements in AI Technology
As AI technology continues to evolve, its potential to enhance market predictions and optimize investment strategies will grow exponentially. In the coming years, AI algorithms will become even more sophisticated, capable of processing larger datasets and generating more accurate forecasts. We can expect advancements in machine learning and deep learning that will allow AI systems to identify patterns, trends, and anomalies in real-time with greater precision.
One significant area of future development is explainable AI (XAI). Currently, one of the main challenges with AI in banking is the “black box” problem, where decision-making processes are not easily understood by human users. XAI aims to provide transparency in AI decision-making, helping banks and investors understand why certain predictions or recommendations are made. This improvement in transparency will increase trust in AI systems, making it easier for financial institutions to implement them at a broader scale. With XAI, regulators, auditors, and customers will have more confidence in AI-powered financial services.
AI and Blockchain Integration
Another future trend that is gaining traction is the integration of AI and blockchain technology. Blockchain, known for its secure and transparent distributed ledger system, can work in tandem with AI to enhance financial transactions and investment processes. AI can analyze transaction patterns within the blockchain to detect fraud, predict market movements, or optimize investment strategies, while blockchain ensures that these transactions are secure and tamper-proof.
For example, combining AI’s predictive capabilities with blockchain’s transparency could lead to more secure and efficient asset trading or investment processes. In areas like smart contracts, AI could automate decision-making processes based on real-time data, ensuring that contracts are executed only when specific conditions are met, reducing the need for intermediaries. This integration will likely lead to more efficient and reliable financial systems, providing enhanced security, faster transaction speeds, and cost savings for banks and investors.
Increased Adoption of AI-Powered Investment Platforms
As AI technology continues to mature, more financial institutions are expected to adopt AI-powered investment platforms. These platforms, which leverage AI for decision-making, portfolio management, and customer service, offer a more efficient, data-driven approach to investment strategies. We will likely see a growing number of robo-advisors that provide personalized investment recommendations at scale, using AI to monitor financial markets and adjust portfolios based on real-time data.
Additionally, AI-based systems will continue to drive advancements in algorithmic trading, improving high-frequency trading (HFT) strategies and automating investment processes for institutions. AI’s ability to analyze large datasets and respond quickly to market changes will make it an indispensable tool for hedge funds, asset managers, and banks. As more financial institutions embrace AI, they will benefit from lower costs, faster decision-making, and improved portfolio performance.
AI in Sustainable Investment
A growing area of interest in the financial world is sustainable investment, where investors focus on ethical or socially responsible companies and initiatives. AI will play a pivotal role in this trend by helping investors identify opportunities in green technologies or environmentally friendly companies. Through natural language processing (NLP) and machine learning algorithms, AI can analyze vast amounts of data, from corporate sustainability reports to news articles and social media, to assess the environmental, social, and governance (ESG) performance of companies.
AI will also enhance predictive modeling for sustainable investments, helping investors forecast the long-term financial performance of companies that are focused on green technologies or ethical business practices. With growing global emphasis on sustainability, AI’s ability to identify investment opportunities in companies that align with these values will drive the future of responsible investing. As a result, banks and investment firms will increasingly rely on AI to offer ethical investment products, creating portfolios that not only generate returns but also contribute to positive social and environmental impact.
Conclusion
Artificial Intelligence (AI) is transforming the banking sector and revolutionizing the way financial institutions predict market trends and optimize investment strategies. By leveraging AI’s ability to analyze massive datasets, process real-time information, and make more accurate predictions, banks can stay competitive in an increasingly data-driven financial landscape. The benefits are clear—AI-driven solutions not only improve accuracy and efficiency but also enhance decision-making, reduce costs, and scale to meet the needs of institutions of all sizes. However, challenges like data privacy, regulatory compliance, and ethical concerns must be carefully managed as AI becomes more integrated into banking systems.
As AI continues to advance, its role in portfolio management, risk mitigation, and sustainable investment will only grow, opening up new opportunities for banks and investors alike. Financial institutions that embrace AI-driven technologies will be better positioned to navigate market volatility, optimize their investments, and deliver enhanced value to their clients.
At Datahub Analytics, we specialize in helping financial institutions harness the power of AI to optimize their investment strategies and streamline market predictions. Our team of AI experts and data analysts work closely with banks to develop custom AI solutions that deliver measurable results. Whether you’re looking to enhance your portfolio management, mitigate risks, or explore opportunities in sustainable investment, Datahub Analytics has the expertise to guide you through the journey.
Ready to unlock the potential of AI for your financial institution? Contact us today to discover how our AI-driven solutions can help you stay ahead in an increasingly competitive market.