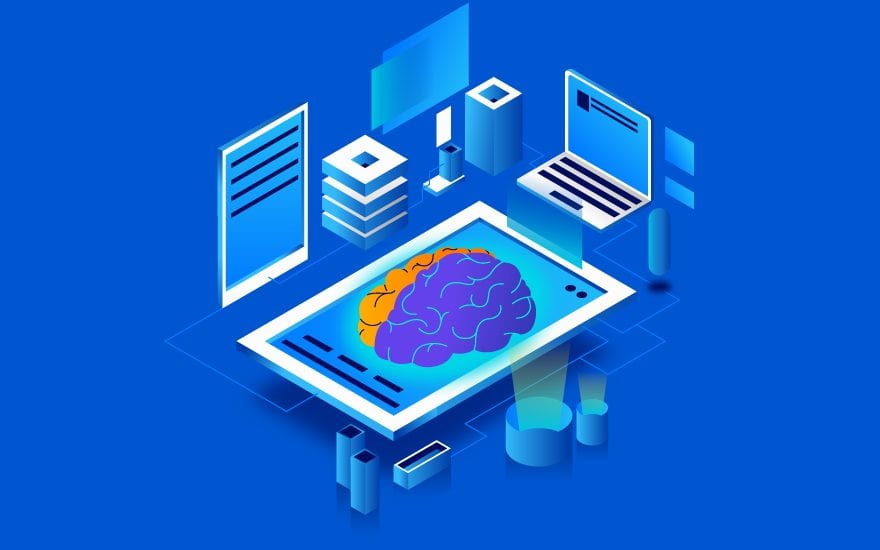
AI-powered Analytics: From Sci-Fi to Reality
AI-powered Analytics: From Sci-Fi to Reality
Imagine a world where businesses can predict market trends with pinpoint accuracy, doctors can diagnose diseases with unprecedented speed, and retailers can anticipate customer needs before they even arise. This isn’t a scene from a sci-fi movie; it’s the reality brought about by AI-powered analytics.
AI-powered analytics is the culmination of years of innovation in artificial intelligence (AI) and data science. Once confined to the realms of science fiction, AI’s capabilities in data analysis have now become integral to various industries, revolutionizing how we interpret and utilize data.
The Evolution of Analytics
Historical Context: Analytics has always been about deriving meaningful insights from data. In the pre-digital era, this involved manual calculations and simple statistical methods. Businesses relied on basic tools like spreadsheets and databases to analyze historical data and make informed decisions. Early data analytics focused on descriptive statistics—summarizing past data to understand what happened.
Emergence of AI: The development of AI dates back to the mid-20th century, with early pioneers like Alan Turing and John McCarthy laying the groundwork. Initially, AI was more of an academic pursuit, focusing on creating machines that could mimic human intelligence. The real shift came with the advent of machine learning (ML) and neural networks, which enabled computers to learn from data and improve over time.
These advancements were driven by increased computational power and the availability of large datasets.
From Theory to Practice: As computational power increased and data storage became cheaper, AI moved from theoretical concepts to practical applications. The integration of AI into analytics began in earnest in the early 2000s, with companies starting to leverage machine learning algorithms to uncover patterns and trends in vast datasets.
The introduction of big data technologies allowed organizations to process and analyze data at an unprecedented scale, paving the way for more sophisticated AI applications in analytics.
How AI is Transforming Analytics Today
Enhanced Data Processing: Traditional data analysis methods struggled with the sheer volume and complexity of modern datasets. AI, particularly machine learning, excels at processing large amounts of data quickly and accurately. Techniques like deep learning allow for the analysis of complex data types, including images and speech.
AI algorithms can handle both structured data (e.g., numerical data, databases) and unstructured data (e.g., text, images), making them versatile tools for modern analytics.
Predictive Analytics: One of the most powerful applications of AI in analytics is its ability to predict future outcomes based on historical data. Predictive models can forecast everything from consumer behavior to equipment failures, enabling proactive decision-making. For instance, in finance, AI models can predict stock price movements, while in healthcare, they can anticipate disease outbreaks.
Predictive analytics leverages techniques such as regression analysis, time series analysis, and machine learning to identify patterns and make accurate predictions.
Natural Language Processing (NLP): NLP is a branch of AI that focuses on the interaction between computers and human language. It allows for the analysis of unstructured data, such as emails, social media posts, and customer reviews. Businesses use NLP to gain insights into customer sentiment, automate customer service, and even generate reports from data.
NLP techniques like sentiment analysis, entity recognition, and language translation are transforming how organizations interact with and understand their customers.
Real-time Analytics: The need for real-time data analysis has grown with the rise of the Internet of Things (IoT) and connected devices. AI enables real-time analytics, allowing organizations to make immediate, data-driven decisions. For example, in retail, AI-powered systems can adjust pricing and inventory in real time based on current demand and supply.
Real-time analytics is critical for industries where timely decision-making is essential, such as finance, healthcare, and manufacturing.
Key Applications of AI-powered Analytics
Business Intelligence: AI enhances traditional business intelligence (BI) tools by automating data analysis and generating deeper insights. Companies can use AI to identify trends, anomalies, and opportunities more efficiently. For example, AI-driven dashboards can provide executives with real-time insights into key performance indicators (KPIs), enabling more agile and informed decision-making.
AI can also perform advanced analytics, such as predictive and prescriptive analytics, which go beyond descriptive analytics to provide actionable recommendations.
Healthcare: In healthcare, AI-powered analytics is transforming patient care and research. AI algorithms can analyze medical images, detect anomalies, and assist in diagnosing conditions like cancer and cardiovascular diseases. Additionally, AI is used to personalize treatment plans based on individual patient data and predict disease progression, improving outcomes and reducing costs.
AI-driven analytics also support clinical decision-making, drug discovery, and population health management.
Finance: The financial sector benefits greatly from AI analytics. AI systems can detect fraudulent transactions in real time, manage risk by analyzing market data, and optimize investment strategies. Robo-advisors, which use AI to provide personalized investment advice, are becoming increasingly popular among retail investors.
AI-powered analytics also enhance customer service through chatbots and automate regulatory compliance processes, reducing operational costs and improving efficiency.
Retail: Retailers use AI to enhance customer experiences and optimize operations. AI analyzes customer behavior data to provide personalized product recommendations, manage inventory by predicting demand, and improve supply chain efficiency. AI-driven chatbots and virtual assistants also enhance customer service by providing instant support.
In addition, AI-powered analytics help retailers optimize pricing strategies, design targeted marketing campaigns, and improve store layouts based on customer movement patterns.
Other Industries: AI-powered analytics has applications across various other industries. In manufacturing, AI optimizes production processes and predicts equipment failures to reduce downtime. In logistics, AI improves route planning and delivery efficiency. Even in agriculture, AI helps in crop monitoring and yield prediction, contributing to sustainable farming practices.
AI analytics also play a crucial role in energy management, public safety, and environmental monitoring, addressing some of the most pressing global challenges.
Challenges and Considerations
Data Quality: The effectiveness of AI analytics depends on the quality of the data. Poor-quality data can lead to inaccurate models and misleading insights. Organizations must invest in data cleaning, validation, and management to ensure their AI systems operate effectively. Ensuring data quality involves addressing issues such as missing data, outliers, and inconsistencies, as well as maintaining data integrity and security.
Ethical Concerns: The use of AI in analytics raises several ethical issues. Privacy concerns arise when personal data is analyzed without consent. Additionally, biases in AI algorithms can lead to unfair or discriminatory outcomes. Organizations must address these ethical concerns by implementing robust data governance policies and ensuring transparency in AI decision-making.
Ethical AI practices include bias detection and mitigation, fairness auditing, and adhering to privacy regulations such as GDPR and CCPA.
Integration Issues: Integrating AI systems with existing infrastructure can be challenging. Legacy systems may not be compatible with modern AI technologies, requiring significant upgrades or replacements. Organizations need a clear strategy for integrating AI into their existing workflows and ensuring seamless interoperability.
This may involve adopting cloud-based AI solutions, building data integration platforms, and fostering collaboration between IT and business teams.
Cost and Resource Requirements: Implementing AI-powered analytics requires substantial investment in technology, talent, and training. AI systems can be expensive to develop and maintain, and there is a growing demand for skilled professionals who can design, implement, and manage these systems. Organizations must weigh the costs against the potential benefits and plan their investments accordingly.
Building a successful AI analytics program involves acquiring the right tools and technologies, hiring data scientists and AI specialists, and providing ongoing training and support.
The Future of AI-powered Analytics
Emerging Trends: The future of AI-powered analytics looks promising, with several emerging trends poised to shape the landscape. Explainable AI (XAI) aims to make AI models more transparent and understandable. Edge AI brings data processing closer to the source, enabling faster decision-making. Additionally, AI-powered analytics will become more accessible to small and medium-sized enterprises (SMEs) as the technology becomes more affordable.
Other emerging trends include the integration of AI with blockchain for secure data sharing, the use of AI in augmented and virtual reality applications, and the development of AI-driven autonomous systems.
Long-term Impact: The long-term impact of AI on various industries will be profound. AI will continue to drive efficiency, innovation, and competitiveness. Businesses that embrace AI-powered analytics will gain a significant advantage, while those that lag behind may struggle to keep up. AI’s influence will extend beyond business, transforming healthcare, education, public services, and more.
In the long run, AI-powered analytics will contribute to solving global challenges such as climate change, resource scarcity, and public health crises, creating a more sustainable and equitable world.
Innovative Applications: The potential for AI-powered analytics is limitless. Future applications could include personalized education programs that adapt to individual learning styles, smart cities that optimize resources and services based on real-time data, and advanced environmental monitoring systems that predict and mitigate the impacts of climate change. As AI technology evolves, we can expect to see even more groundbreaking applications that enhance our lives and solve complex global challenges.
Innovations such as AI-driven healthcare diagnostics, predictive maintenance in critical infrastructure, and AI-powered disaster response systems will shape the future of our society.
Conclusion
Embracing AI analytics is crucial for organizations and individuals seeking to thrive in the data-driven world of the future. By leveraging the power of AI, businesses can uncover hidden insights, anticipate market shifts, and drive innovation. However, the journey toward full integration of AI in analytics is not without its hurdles, and it requires a strategic approach to overcome challenges related to data quality, ethical considerations, integration issues, and resource requirements.
Stay informed about the latest developments in AI and analytics. Consider how AI-powered analytics can benefit your organization or field of interest. Explore educational resources, attend webinars, and participate in discussions to deepen your understanding of this transformative technology. By being proactive and open to learning, you can position yourself and your organization at the forefront of the AI revolution.
Harness the Power of AI-Powered Analytics with Datahub Analytics
We believe that AI-powered analytics is not just the future—it’s the present. We specialize in helping businesses transform their data into actionable insights, driving innovation, efficiency, and growth. Our cutting-edge AI solutions are designed to meet the unique needs of various industries, from healthcare and finance to retail and manufacturing.