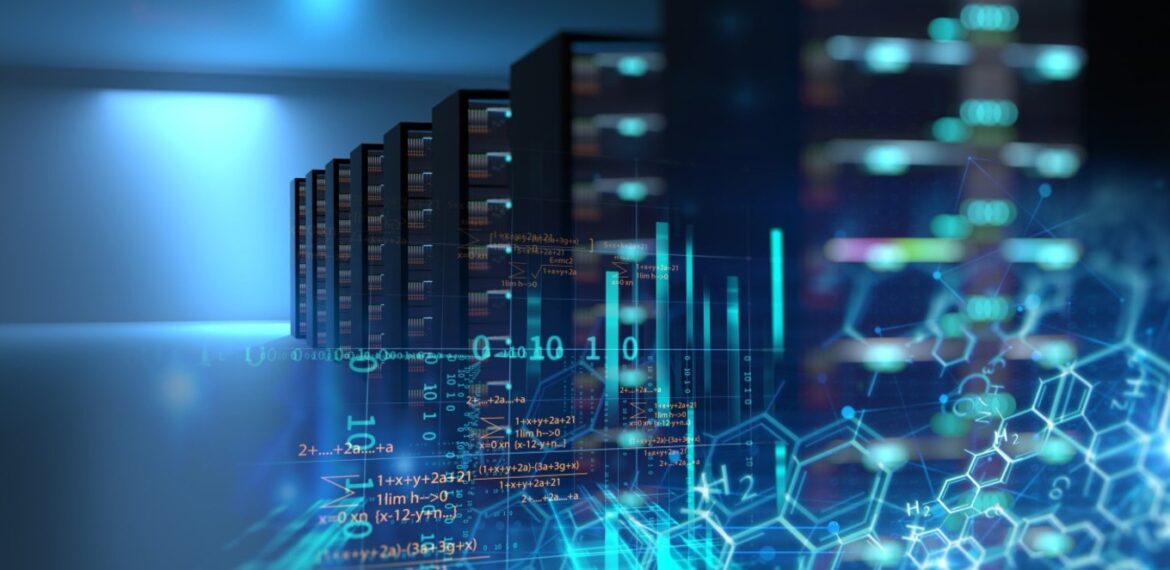
Demystifying the Modern Data Warehouse: Evolution and Essentials
Demystifying the Modern Data Warehouse: Evolution and Essentials
In today’s data-centric world, the concept of modern data warehousing has emerged as a pivotal force in shaping how organizations manage, analyze, and derive insights from their data assets. As businesses grapple with vast amounts of information generated at unprecedented speeds, the adoption of modern data warehousing has become a strategic imperative for staying competitive and agile in the ever-evolving landscape.
At its core, modern data warehousing represents a paradigm shift from the traditional methods of data storage and analysis. It leverages cutting-edge technologies and cloud-based architectures to transform how data is collected, processed, and turned into actionable insights. This transition from traditional to modern approaches reflects the pressing need for organizations to embrace scalable, real-time, and agile solutions that can keep pace with the demands of today’s fast-paced business environment.
Traditional vs. Modern Data Warehousing:
The evolution from traditional data warehousing to the modern approach marks a fundamental shift in how organizations manage and leverage their data assets. Let’s delve into the distinctions between these two paradigms and uncover the limitations of traditional methods that have driven the adoption of modern data warehousing.
Traditional Data Warehousing:
Traditional data warehousing, born in the era of on-premises computing, involved centralized repositories for storing historical data. It was characterized by:
Batch Processing: Data was collected over time and processed in periodic batches, leading to delayed insights that were often outdated by the time they were analyzed.
Scalability Challenges: As data volumes grew, traditional warehouses struggled to scale efficiently, often requiring significant investments in hardware and infrastructure.
Limited Flexibility: Adapting to changing data formats and types was complex and time-consuming, making it challenging to handle the diverse data sources of today’s digital landscape.
High Costs: The expenses associated with hardware, maintenance, and physical storage made traditional warehousing cost-prohibitive for many businesses.
Modern Data Warehousing:
The modern data warehousing approach addresses these limitations head-on, offering a range of benefits that align with the demands of the digital age:
Real-Time Analytics: Modern data warehousing enables real-time data processing and analysis, allowing businesses to make informed decisions based on the freshest insights available.
Scalability and Elasticity: Cloud-based architectures provide scalable resources on demand, allowing organizations to expand or contract their data infrastructure as needed.
Flexibility and Agility: Modern data warehousing supports diverse data types and formats, accommodating structured, semi-structured, and unstructured data sources seamlessly.
Cost-Efficiency: By eliminating the need for extensive on-premises infrastructure, modern data warehousing offers a more cost-effective solution with pay-as-you-go pricing models.
Global Accessibility: Cloud-based modern data warehouses provide accessibility from anywhere, facilitating collaboration and analysis across distributed teams.
Advanced Analytics: The modern approach integrates advanced analytics techniques, such as machine learning and AI, to uncover deeper insights from data.
By adopting modern data warehousing, organizations position themselves to tackle data challenges head-on, unlocking the potential of their information assets to drive innovation and growth.
Key Components of Modern Data Warehousing:
A modern data warehouse is a dynamic ecosystem built on a foundation of essential components that work in concert to enable efficient data storage, processing, and analysis. Let’s explore these key components that define the modern data warehousing landscape.
1. Cloud Infrastructure:
Cloud computing forms the bedrock of modern data warehousing. Cloud platforms offer scalable and elastic resources that accommodate varying data volumes and workloads. This eliminates the need for upfront hardware investments and allows businesses to adapt their resources as demands change.
2. Data Integration Platforms:
Data integration tools and platforms play a crucial role in aggregating and unifying data from disparate sources. These platforms enable seamless extraction, transformation, and loading (ETL) processes, ensuring that data is clean, consistent, and ready for analysis.
3. Data Storage:
The modern data warehouse employs a combination of data storage solutions, including data lakes and data warehouses. Data lakes store raw and unstructured data, while data warehouses house structured and processed data that are optimized for querying and analytics.
4. Data Processing Engines:
Data processing engines handle the heavy lifting of querying and analyzing data. They allow for the efficient execution of complex queries across massive datasets, enabling organizations to derive insights quickly and accurately.
5. Analytics and Visualization Layers:
Modern data warehouses offer powerful analytics and visualization tools that empower users to explore data, create reports, and generate insights. These tools facilitate interactive exploration and sharing of findings within the organization.
6. Data Governance and Security:
Robust data governance practices ensure data quality, compliance, and security. Policies and controls are put in place to manage data access, monitor data usage, and maintain regulatory compliance.
7. Real-Time Capabilities:
Real-time data processing and analytics capabilities enable organizations to analyze data as it’s generated, allowing for timely decision-making and proactive responses to emerging trends.
8. Scalability and Elasticity:
Modern data warehouses are designed to scale seamlessly. They can handle both sudden spikes in workload and steady growth in data volumes, ensuring consistent performance even under heavy demand.
9. Automation and Orchestration:
Automation and orchestration tools streamline processes like data transformation, workflow scheduling, and resource management. This enhances efficiency and reduces manual intervention.
10. Machine Learning Integration:
Many modern data warehouses offer integration with machine learning frameworks. This enables organizations to apply predictive and prescriptive analytics, uncover patterns, and make data-driven predictions.
Understanding the synergy between these components is key to harnessing the full potential of modern data warehousing.
Challenges and Considerations:
While modern data warehousing offers an array of benefits, the journey to adopting this approach is not without its challenges and considerations. Organizations need to navigate these potential hurdles to ensure a successful transition to the modern data warehouse paradigm.
1. Data Migration:
Transitioning from traditional data warehousing to a modern solution involves migrating data from legacy systems. Data migration can be complex, requiring careful planning to ensure data integrity, consistency, and accuracy during the transfer.
2. Vendor Selection:
Choosing the right cloud platform and data warehouse vendor is a critical decision. Organizations need to assess factors such as features, performance, scalability, pricing, and vendor reliability before making a choice.
3. Data Quality and Preparation:
Ensuring data quality is essential for accurate analysis. Data from various sources may need cleansing, transformation, and enrichment to ensure consistency and reliability in the modern data warehouse.
4. Cost Management:
While cloud-based solutions offer cost advantages, improper resource allocation and management can lead to unexpected expenses. Organizations must optimize their cloud usage to avoid unnecessary costs.
5. Data Governance and Compliance:
Data governance practices must be established to maintain data quality, security, and regulatory compliance in the cloud environment. Compliance with data protection regulations becomes paramount when dealing with sensitive data.
6. Skill Set and Training:
Transitioning to modern data warehousing may require new skills from IT teams. Training or hiring personnel familiar with cloud technologies, data integration, and analytics is crucial for successful implementation.
7. Cultural Shift:
Adapting to a new approach may require a cultural shift within the organization. Stakeholders need to embrace the change and understand the benefits of modern data warehousing.
8. Performance Monitoring and Optimization:
Modern data warehouses require continuous monitoring to ensure optimal performance. Organizations should be prepared to troubleshoot performance issues and optimize resources as needed.
9. Integration Challenges:
Integrating with existing systems and applications can be challenging. Ensuring seamless connectivity between the modern data warehouse and other tools is essential for data flow and analytics.
10. Change Management:
Change management is crucial to ensure a smooth transition. Organizations need to communicate the benefits of modern data warehousing and provide adequate training to users.
By addressing these challenges proactively and strategically, organizations can minimize risks and maximize the benefits of modern data warehousing.
Conclusion:
In the dynamic landscape of data management and analytics, modern data warehousing stands as a beacon of innovation and transformation. Let’s recap the key takeaways and reinforce the profound impact of modern data warehousing on data-driven decision-making.
Embracing Scalability and Real-Time Insights:
Modern data warehousing offers organizations the ability to scale resources as data volumes grow and evolve. The real-time analytics capabilities of modern data warehouses empower businesses to harness insights from data as it’s generated, fostering agility and prompt decision-making.
Efficiency Through Cloud Infrastructure:
The cloud-based foundation of modern data warehousing provides organizations with the flexibility, scalability, and cost-efficiency needed to adapt to changing data demands and business needs.
Holistic Data Ecosystem:
Modern data warehousing goes beyond traditional data storage, encompassing data integration, transformation, analytics, and visualization within a single ecosystem. This holistic approach enables seamless data flow and comprehensive insights.
Navigating Challenges Strategically:
While modern data warehousing offers transformative advantages, it’s important to acknowledge and address challenges such as data migration, vendor selection, and data governance. A strategic approach to overcoming these challenges is vital for a successful transition.
Empowering Data-Driven Decision-Making:
Modern data warehousing plays a pivotal role in transforming raw data into actionable insights that inform strategic decisions. By enabling organizations to analyze data rapidly, accurately, and in real-time, modern data warehousing empowers a new era of data-driven decision-making.
In an era where data is a driving force behind innovation and competitiveness, the adoption of modern data warehousing marks a fundamental shift in how organizations leverage their information assets. As you continue your data journey, remember that modern data warehousing isn’t just about technology; it’s about embracing a culture of data-driven excellence that guides organizations toward smarter, more informed decisions.
By harnessing the power of modern data warehousing, you’re positioned to thrive in an increasingly data-driven world, where insights are the currency of success.