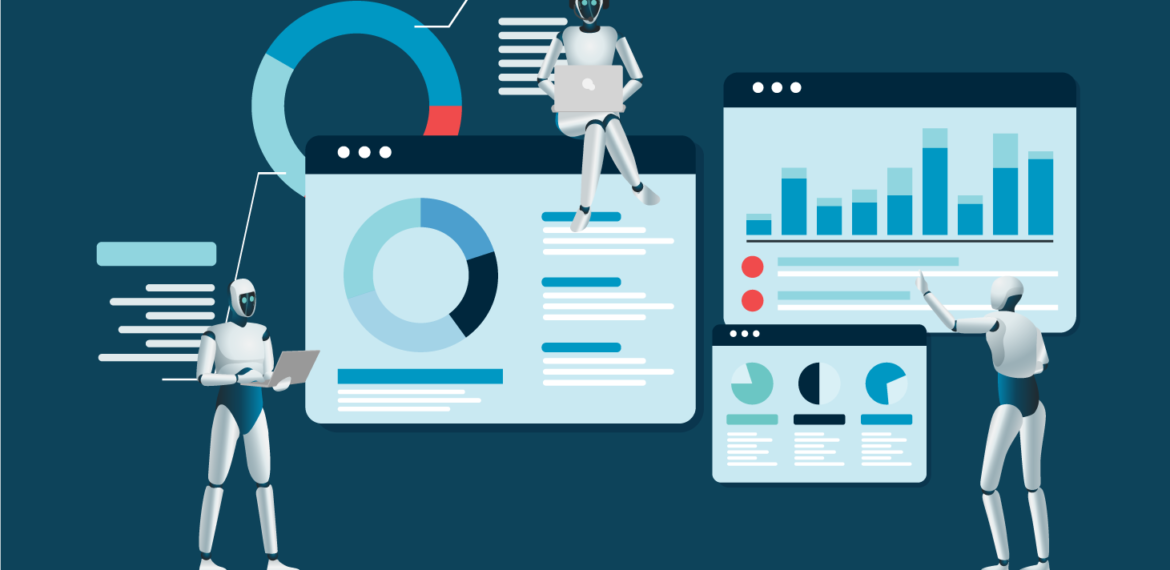
Generative AI and Data Analytics: Enhancing Data Analysis, Automation, and Insights Generation
Generative AI and Data Analytics: Enhancing Data Analysis, Automation, and Insights Generation
In the rapidly evolving world of data analytics, the integration of Generative AI technologies has begun to reshape how organizations approach data analysis, automation, and insights generation. Generative AI models, like ChatGPT, are increasingly becoming indispensable tools in the data analytics toolkit, offering transformative capabilities that extend far beyond traditional methods. This blog explores how these AI models are revolutionizing the field and the practical implications for businesses and analysts.
The Rise of Generative AI
Generative AI refers to a subset of artificial intelligence designed to generate new content, predictions, or responses based on existing data. Unlike traditional AI systems that primarily focus on recognizing patterns or making predictions, generative models create novel outputs that can closely mimic human-like creativity and reasoning. This capability is powered by advanced neural network architectures and large-scale training datasets, enabling models like ChatGPT to understand context, generate text, and even simulate complex scenarios.
Enhancing Data Analysis with Generative AI
- Natural Language Processing for Data Interpretation
One of the most impactful applications of generative AI in data analytics is its ability to interpret and communicate complex data insights through natural language. Traditional data analysis often involves translating statistical outputs into actionable insights, a task that can be cumbersome and prone to misinterpretation. Generative AI models can bridge this gap by automatically generating comprehensible summaries and explanations of data trends, patterns, and anomalies.
For instance, ChatGPT can analyze a dataset and produce a narrative report that highlights key findings, correlations, and recommendations in plain language. This capability not only streamlines the reporting process but also democratizes data access, making insights more accessible to non-technical stakeholders.
- Automated Data Cleaning and Preparation
Data cleaning and preparation are critical yet often time-consuming steps in the data analysis pipeline. Generative AI can significantly accelerate these processes by automating data wrangling tasks. For example, AI models can identify and rectify inconsistencies, fill in missing values, and even suggest transformations to enhance data quality.
By leveraging generative models, organizations can reduce the manual effort required for data preparation and improve the accuracy and reliability of their datasets. This allows analysts to focus on higher-level tasks, such as interpreting results and deriving actionable strategies.
- Predictive Analytics and Scenario Simulation
Generative AI excels in creating simulations and predictive models that can forecast future trends based on historical data. This capability is particularly valuable for scenario analysis, where businesses need to evaluate the potential outcomes of different strategies or decisions.
For example, ChatGPT can generate hypothetical scenarios based on varying inputs and simulate how different variables might impact future outcomes. This enables data analysts to explore a broader range of possibilities and make more informed decisions by considering various “what-if” scenarios.
- Customized Data Insights and Recommendations
Every business has unique data needs and challenges, and generative AI can tailor its insights and recommendations accordingly. By analyzing specific datasets and understanding the context in which they are used, AI models can provide personalized suggestions that align with organizational goals.
ChatGPT, for instance, can generate customized recommendations for optimizing business processes, improving customer experiences, or enhancing operational efficiency. These insights are grounded in data-driven analysis and can be adjusted to reflect changing conditions or new information.
Revolutionizing Automation in Data Analytics
- Automated Reporting and Dashboard Generation
Generative AI can automate the creation of reports and dashboards, transforming raw data into visually appealing and informative presentations. This automation not only speeds up the reporting process but also ensures consistency and accuracy in the generated outputs.
AI models like ChatGPT can design dashboards that highlight key metrics and trends, generate periodic reports, and even provide real-time updates on data changes. This level of automation reduces the manual effort required for reporting and allows organizations to focus on interpreting and acting upon the insights provided.
- Enhanced Data Querying and Interaction
Interacting with data through traditional query languages can be complex and requires specialized knowledge. Generative AI simplifies this process by enabling natural language querying, allowing users to ask questions about their data in plain language.
For example, instead of writing complex SQL queries, users can simply ask ChatGPT, “What were the sales figures for the last quarter?” The AI model will then interpret the query, retrieve the relevant data, and present the results in an understandable format. This ease of interaction makes data querying more accessible and user-friendly.
- Intelligent Data Augmentation
Generative AI can also assist in data augmentation by creating synthetic data that complements existing datasets. This is particularly useful in scenarios where real data is scarce or incomplete. By generating synthetic data that mimics real-world patterns, organizations can enhance their datasets and improve the robustness of their analyses.
ChatGPT can contribute to this process by generating additional data points based on existing trends and distributions. This augmented data can be used for training machine learning models, conducting simulations, or validating analytical results.
Insights Generation: From Data to Decision-Making
- Actionable Insights and Strategy Formulation
The ultimate goal of data analytics is to derive actionable insights that drive strategic decision-making. Generative AI models can play a crucial role in this process by synthesizing data findings and providing strategic recommendations.
For instance, ChatGPT can analyze market trends, customer feedback, and operational data to generate insights that inform business strategies. These insights can include recommendations for product development, marketing strategies, or operational improvements, all grounded in comprehensive data analysis.
- Continuous Learning and Adaptation
Generative AI models are not static; they continuously learn and adapt based on new data and user interactions. This dynamic capability allows AI models like ChatGPT to stay current with evolving trends and provide up-to-date insights.
As businesses accumulate more data and refine their strategies, generative AI models can adjust their analyses and recommendations accordingly. This ensures that insights remain relevant and actionable in a constantly changing environment.
Challenges and Considerations in Integrating Generative AI
While generative AI holds great promise for enhancing data analytics, its integration into existing systems and processes comes with its own set of challenges and considerations. Addressing these challenges is crucial for maximizing the benefits of AI technologies while ensuring their responsible and effective use.
- Data Privacy and Security
The use of generative AI in data analytics raises significant concerns about data privacy and security. AI models, especially those trained on large datasets, can inadvertently expose sensitive information if not properly managed. Ensuring that AI systems adhere to strict data protection regulations and implementing robust security measures are essential for safeguarding confidential data.
Organizations must also be vigilant about the sources of data used to train AI models, ensuring that they do not inadvertently incorporate personal or sensitive information. Privacy-preserving techniques, such as data anonymization and encryption, can help mitigate these risks.
- Bias and Fairness
Generative AI models can perpetuate or even amplify existing biases present in the training data. Bias in data can lead to skewed insights and reinforce unfair practices or decisions. It is crucial to implement strategies for identifying and addressing biases in AI models to ensure fairness and equity in the generated insights.
Regular audits of AI systems, diverse training datasets, and transparent model evaluation processes are essential for mitigating bias and ensuring that AI-generated recommendations are fair and unbiased.
- Accuracy and Reliability
While generative AI models like ChatGPT are powerful, they are not infallible. The accuracy and reliability of AI-generated insights can vary depending on the quality of the input data and the model’s training. Ensuring that AI systems are rigorously tested and validated before deployment is critical for maintaining the integrity of the analytical results.
Additionally, human oversight is important to verify and interpret AI-generated insights, especially when making high-stakes decisions. Combining AI capabilities with expert analysis can enhance the reliability of the results.
- Integration with Existing Systems
Integrating generative AI into existing data analytics workflows and systems can be complex. Organizations may face challenges related to compatibility, data interoperability, and the need for additional infrastructure. It is essential to carefully plan and execute the integration process to minimize disruptions and ensure seamless operation.
Collaborating with AI experts and investing in robust integration solutions can facilitate the smooth adoption of generative AI technologies.
- Ethical Considerations
The deployment of generative AI raises ethical questions about the role of AI in decision-making processes. Organizations must consider the ethical implications of using AI-generated insights and ensure that these technologies are used responsibly and transparently.
Developing and adhering to ethical guidelines for AI use, engaging stakeholders in discussions about AI ethics, and fostering a culture of accountability are important steps in addressing these concerns.
Future Directions and Emerging Trends
As generative AI continues to advance, several emerging trends and future directions are likely to shape its role in data analytics. These trends will influence how organizations leverage AI technologies and adapt to new opportunities and challenges.
- Advanced AI Models and Techniques
The development of more sophisticated AI models and techniques will enhance the capabilities of generative AI in data analytics. Future models are expected to offer improved accuracy, context understanding, and customization options, making them even more effective in generating actionable insights.
Innovations such as transfer learning, few-shot learning, and hybrid AI approaches will further expand the possibilities of generative AI, allowing for more nuanced and adaptive analyses.
- Integration with Other Emerging Technologies
Generative AI is likely to be increasingly integrated with other emerging technologies, such as the Internet of Things (IoT), edge computing, and blockchain. These integrations will create new opportunities for data collection, analysis, and security, further enhancing the capabilities of AI-driven analytics.
For example, combining AI with IoT data can provide real-time insights into operational performance, while blockchain technology can ensure the integrity and security of data used for AI analysis.
- Enhanced Human-AI Collaboration
The future of data analytics will involve greater collaboration between humans and AI systems. AI technologies will complement human expertise, enabling analysts to leverage AI-generated insights while applying their domain knowledge and critical thinking skills.
Training and upskilling programs for data professionals will focus on developing skills to effectively collaborate with AI systems and interpret their outputs. This collaborative approach will enhance decision-making and drive innovation.
- Personalized AI Solutions
As AI models become more advanced, the ability to provide personalized solutions tailored to specific business needs will improve. Organizations will be able to customize AI systems to address their unique data challenges and goals, resulting in more relevant and actionable insights.
Personalized AI solutions will enable businesses to optimize their data analytics processes, enhance customer experiences, and achieve strategic objectives more effectively.
- Ethical AI and Regulation
The ethical use of AI and the establishment of regulatory frameworks will be crucial for the responsible deployment of generative AI technologies. Governments, organizations, and industry bodies will work together to develop standards and guidelines that ensure the ethical and transparent use of AI in data analytics.
Ongoing discussions about AI ethics, fairness, and accountability will shape the future of AI deployment, promoting trust and ensuring that AI technologies are used for the benefit of society.
Conclusion
Generative AI models like ChatGPT are transforming the landscape of data analytics by enhancing data interpretation, automating processes, and generating valuable insights. While the integration of these technologies presents challenges related to privacy, bias, accuracy, and ethics, the potential benefits are substantial.
As AI technologies continue to advance, organizations that embrace generative AI will be well-positioned to harness its capabilities for improved decision-making, strategic planning, and operational efficiency. By addressing the challenges and staying abreast of emerging trends, businesses can effectively leverage generative AI to drive innovation and achieve their data-driven goals.