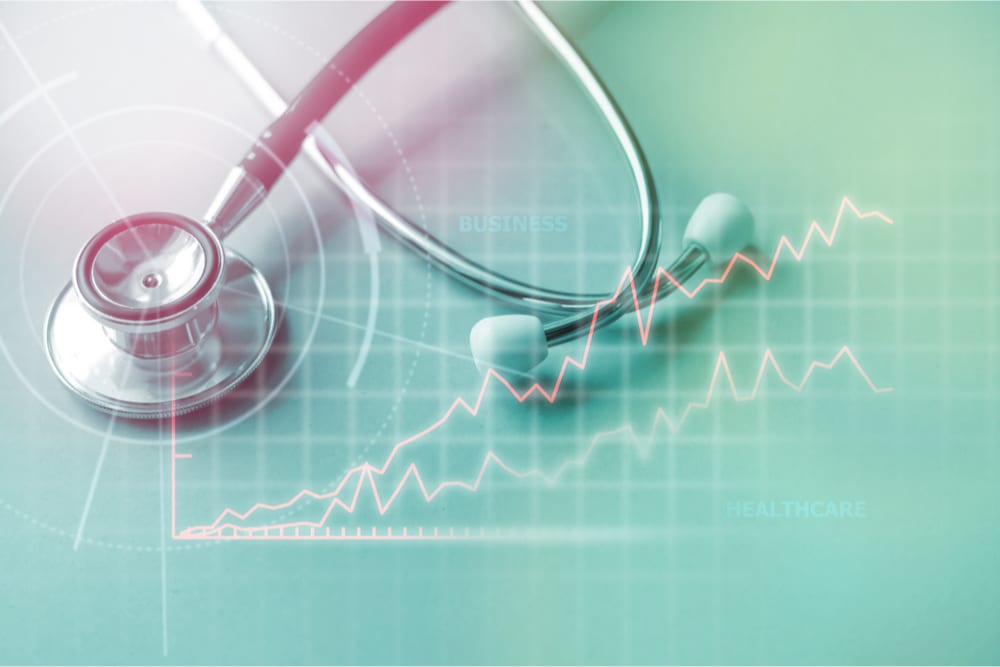
Rising Healthcare Costs: How Data Analytics Can Optimize Resource Allocation and Reduce Expenses
Rising Healthcare Costs: How Data Analytics Can Optimize Resource Allocation and Reduce Expenses
Healthcare costs have been on a relentless rise, driven by a combination of factors including technological advancements, an aging population, and the increasing prevalence of chronic conditions. This trend poses a significant challenge for healthcare systems worldwide, as they grapple with the need to deliver high-quality care while managing ever-expanding budgets. Amidst these challenges, data analytics emerges as a powerful tool to address the issue of rising healthcare costs. By harnessing the power of data, healthcare organizations can optimize resource allocation, improve operational efficiency, and ultimately reduce expenses.
Understanding the Problem
The increase in healthcare costs can be attributed to several key factors:
- Aging Population: As people live longer, there is a higher demand for medical care, often for chronic and complex conditions. This demographic shift places a strain on healthcare resources and drives up costs.
- Technological Advancements: While new technologies and treatments can enhance patient care, they often come with a high price tag. The cost of implementing and maintaining advanced medical equipment and treatments contributes to the overall rise in expenses.
- Chronic Diseases: The prevalence of chronic conditions such as diabetes, heart disease, and obesity requires ongoing management and intervention. This not only increases the frequency of healthcare visits but also necessitates expensive medications and treatments.
- Administrative Inefficiencies: The healthcare system often suffers from administrative inefficiencies, including billing errors, redundant processes, and poor resource management. These inefficiencies contribute to higher operational costs and affect the overall cost of care.
The Role of Data Analytics in Healthcare
Data analytics refers to the process of examining large volumes of data to uncover patterns, correlations, and insights that can inform decision-making. In the context of healthcare, data analytics can play a transformative role in addressing rising costs by providing actionable insights that drive more efficient and effective resource allocation. Here are several key areas where data analytics can make a significant impact:
1. Predictive Analytics for Patient Care
Predictive analytics involves using historical data and statistical algorithms to forecast future events. In healthcare, predictive analytics can be used to anticipate patient needs and optimize resource allocation. For instance:
- Early Intervention: By analyzing data on patient demographics, medical history, and lifestyle factors, healthcare providers can identify individuals at high risk for certain conditions. Early intervention can then be targeted to prevent or manage these conditions before they require costly treatments.
- Hospital Readmission Prevention: Predictive models can identify patients at risk of readmission based on their previous hospital stays, treatment plans, and other factors. By proactively addressing these risks, hospitals can reduce readmission rates and associated costs.
2. Optimization of Resource Utilization
Healthcare facilities often face challenges related to resource management, including staff allocation, equipment usage, and bed availability. Data analytics can help optimize these resources by:
- Demand Forecasting: Analyzing historical data on patient volumes, seasonal trends, and appointment patterns allows healthcare facilities to better predict and manage demand for services. This helps in staffing decisions and ensures that resources are available when needed.
- Efficiency Improvement: Data analytics can identify inefficiencies in workflows and processes, such as unnecessary tests or redundant procedures. By streamlining operations and eliminating waste, healthcare organizations can reduce costs and improve patient care.
3. Cost Management and Reduction
Effective cost management is crucial for controlling healthcare expenses. Data analytics can assist in:
- Cost Analysis: By examining cost data across different departments, procedures, and patient groups, healthcare organizations can identify areas where costs are disproportionately high. This insight allows for targeted cost-saving measures and budget adjustments.
- Contract Optimization: Analyzing claims data and payer contracts can help healthcare organizations negotiate better terms with insurers and vendors. Understanding cost drivers and utilization patterns enables more informed negotiations and contract management.
4. Population Health Management
Population health management focuses on improving the health outcomes of specific groups of patients, often with an emphasis on prevention and chronic disease management. Data analytics can support population health initiatives by:
- Identifying High-Risk Populations: Analyzing data on social determinants of health, health behaviors, and medical history helps identify high-risk populations who may benefit from targeted interventions.
- Coordinating Care: Data integration across different care settings allows for better coordination of care for patients with chronic conditions. This can lead to more effective management of their health and reduced overall costs.
5. Enhanced Decision-Making
Healthcare decisions, from clinical to administrative, are often based on incomplete or subjective information. Data analytics provides a more objective basis for decision-making by:
- Evidence-Based Decisions: Analyzing clinical outcomes and treatment effectiveness helps healthcare providers make evidence-based decisions, leading to better patient outcomes and more efficient use of resources.
- Benchmarking and Performance Improvement: Data analytics allows healthcare organizations to benchmark their performance against industry standards and identify areas for improvement. This drives continuous quality improvement and cost reduction.
Practical Applications and Case Studies
To illustrate how data analytics can effectively address rising healthcare costs, let’s explore some real-world applications and case studies where data-driven strategies have been successfully implemented.
1. Reducing Readmission Rates: The Case of Mount Sinai Health System
Mount Sinai Health System, a major healthcare provider in New York City, has made significant strides in reducing readmission rates through the use of data analytics. By employing predictive analytics models, they identified patients at high risk of readmission based on factors such as previous hospitalizations, medical conditions, and social determinants of health.
Implementation:
- Predictive Models: Mount Sinai developed predictive models that analyzed electronic health records (EHRs) and other patient data to estimate the likelihood of readmission.
- Targeted Interventions: Patients identified as high-risk were given targeted interventions, including follow-up care, personalized health plans, and support services.
Results:
- Readmission Reduction: The targeted interventions led to a significant decrease in readmission rates, resulting in cost savings for both the healthcare system and patients.
- Improved Outcomes: Patients received more focused care, improving their overall health outcomes and reducing the need for costly emergency interventions.
2. Optimizing Hospital Resource Utilization: The Cleveland Clinic Experience
The Cleveland Clinic, a renowned healthcare provider, utilized data analytics to optimize hospital resource utilization, including staff scheduling, equipment use, and bed management. This effort aimed to enhance operational efficiency and reduce costs associated with underutilized resources.
Implementation:
- Data Integration: The Cleveland Clinic integrated data from various sources, including EHRs, operational systems, and patient flow metrics.
- Resource Forecasting: Advanced analytics were used to forecast demand for resources based on historical trends and real-time data.
Results:
- Increased Efficiency: The clinic achieved better alignment of staff schedules with patient needs, minimizing downtime and improving patient care.
- Cost Savings: Optimized resource utilization led to reduced operational costs and improved overall efficiency within the hospital.
3. Managing Population Health: The Case of Geisinger Health System
Geisinger Health System, a large integrated healthcare provider, implemented a population health management strategy using data analytics to manage chronic conditions and improve health outcomes for its patient population.
Implementation:
- Risk Stratification: Geisinger used data analytics to identify high-risk patients with chronic conditions such as diabetes and cardiovascular disease.
- Care Coordination: A coordinated care approach was adopted, with care teams working together to provide personalized care plans and interventions.
Results:
- Improved Health Outcomes: The approach led to better management of chronic conditions, reducing complications and hospitalizations.
- Cost Reduction: By focusing on preventive care and effective management of chronic diseases, Geisinger achieved significant cost savings and improved patient quality of life.
4. Cost Management Through Claims Analysis: The Blue Cross Blue Shield Experience
Blue Cross Blue Shield (BCBS) undertook a comprehensive analysis of claims data to manage and reduce healthcare costs. This analysis focused on identifying high-cost claims and understanding the drivers behind these expenses.
Implementation:
- Claims Data Analysis: BCBS analyzed claims data to identify patterns and trends in high-cost procedures and treatments.
- Strategic Interventions: The organization used this insight to negotiate better terms with providers, implement cost-control measures, and promote evidence-based care practices.
Results:
- Cost Containment: By addressing high-cost claims and optimizing provider contracts, BCBS achieved significant cost savings.
- Enhanced Care Quality: The focus on evidence-based practices led to improved care quality and better patient outcomes.
Future Trends and Considerations
As healthcare continues to evolve, several trends and considerations will shape the future of data analytics in managing costs:
1. Artificial Intelligence and Machine Learning
The integration of artificial intelligence (AI) and machine learning (ML) in data analytics is expected to enhance predictive models and decision-making capabilities. AI-driven tools can analyze vast amounts of data quickly, uncovering insights that were previously difficult to detect. This advancement will further improve resource allocation, patient care, and cost management.
2. Telemedicine and Remote Monitoring
The rise of telemedicine and remote monitoring technologies provides new opportunities for data collection and analysis. By monitoring patients remotely, healthcare providers can gather real-time data on patient health and treatment adherence, leading to more proactive and cost-effective care.
3. Data Privacy and Security
As healthcare organizations increasingly rely on data analytics, ensuring the privacy and security of patient data becomes paramount. Adhering to data protection regulations and implementing robust security measures will be essential in maintaining patient trust and protecting sensitive information.
4. Integration Across Systems
Seamless integration of data across different healthcare systems and platforms will be crucial for achieving a comprehensive view of patient health and resource utilization. Interoperability between EHRs, billing systems, and other healthcare technologies will enhance the effectiveness of data-driven strategies.
Conclusion
Data analytics holds immense potential for addressing the challenge of rising healthcare costs. By leveraging predictive analytics, optimizing resource utilization, managing population health, and implementing targeted cost-control measures, healthcare organizations can achieve significant cost savings while improving patient care. As technology advances and data analytics continues to evolve, the future promises even greater opportunities for enhancing efficiency and reducing expenses in the healthcare sector. Embracing these data-driven approaches will be key to building a more sustainable and effective healthcare system for the future.