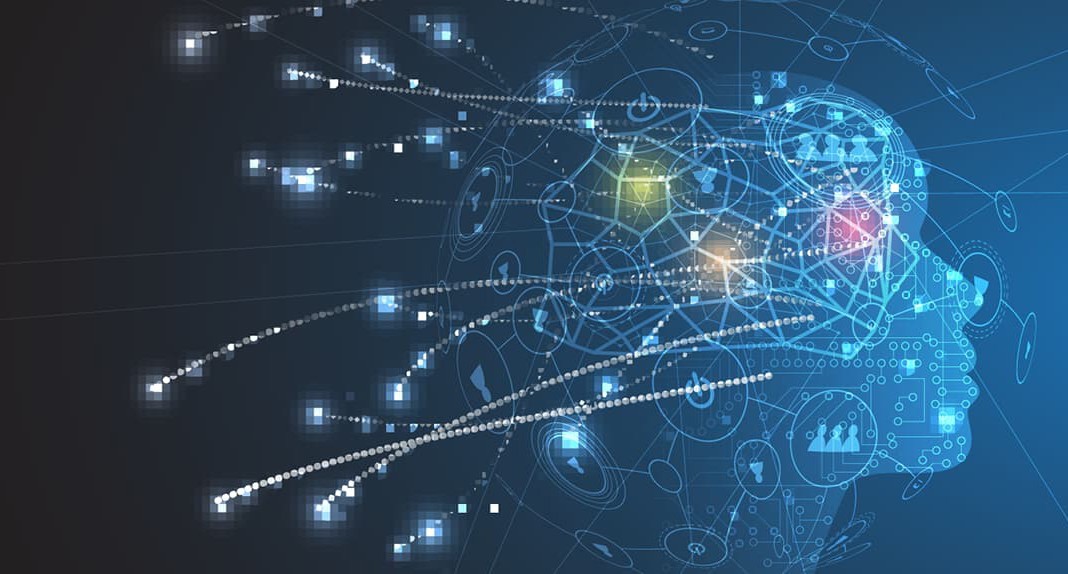
Integrating AI with Data Warehousing: Transforming Data Management in 2025
Integrating AI with Data Warehousing: Transforming Data Management in 2025
The evolution of data warehousing has reached a turning point with the integration of Artificial Intelligence (AI). Traditional data warehouses, once limited by manual processes and static reporting, are now transforming into dynamic, intelligent ecosystems that enable businesses to extract real-time insights, automate data management, and scale seamlessly.
AI’s Role in Revolutionizing Data Warehousing
AI is reshaping data warehousing by introducing advanced capabilities such as automated data ingestion, predictive analytics, and self-optimizing storage solutions. By leveraging AI, organizations can process vast amounts of structured and unstructured data faster than ever before, eliminating the bottlenecks associated with conventional ETL (Extract, Transform, Load) processes. Additionally, machine learning models can detect anomalies, optimize queries, and enhance data governance, ensuring accuracy and compliance across all operations.
The Growing Demand for Real-Time Insights, Scalability, and Automation
As businesses generate and rely on more data, the demand for real-time analytics has surged. Organizations need insights on customer behavior, market trends, and operational efficiencies within seconds—not hours or days. AI-driven data warehouses meet this need by leveraging in-memory computing, automated indexing, and intelligent caching techniques. Additionally, AI enhances scalability by dynamically allocating resources based on demand, ensuring performance remains optimal without excessive infrastructure costs.
Why AI-Driven Data Warehouses Are Essential for Competitiveness in 2025
In 2025, businesses that fail to adopt AI-driven data warehouses risk falling behind competitors that leverage AI for faster decision-making, improved data accuracy, and cost efficiency. AI-powered automation reduces reliance on manual data engineering tasks, allowing organizations to focus on innovation and strategic initiatives. Additionally, AI-driven data quality management ensures that insights are based on reliable and accurate information, ultimately leading to better business outcomes.
As we move further into the AI-driven era, businesses must embrace AI-enabled data warehousing to stay agile, competitive, and future-ready.
The Evolution of Data Warehousing
Data warehousing has undergone significant transformations over the past few decades, driven by the increasing volume, velocity, and variety of data. Traditional data warehouses, once designed for batch processing and historical analysis, are no longer sufficient for businesses that require real-time insights and agile decision-making. The emergence of AI and cloud technologies has paved the way for next-generation data warehousing solutions that are more efficient, scalable, and intelligent.
Challenges of Traditional Data Warehousing
Early data warehousing solutions were built on rigid architectures that struggled to keep up with modern business demands. Some key limitations included:
- Slow ETL Processes – Traditional Extract, Transform, Load (ETL) pipelines often required hours or even days to process and load data, delaying insights and decision-making.
- Lack of Real-Time Data Processing – Legacy systems primarily supported batch processing, making it difficult to deliver up-to-the-minute analytics.
- Scalability Issues – On-premise data warehouses required extensive hardware investments and manual scaling efforts, limiting flexibility and responsiveness to changing data demands.
- High Maintenance Costs – Maintaining traditional data warehouses involved significant infrastructure, software, and personnel costs.
- Data Silos and Integration Challenges – Legacy systems struggled to integrate diverse data sources, leading to fragmented insights and inefficiencies.
The Shift to Cloud-Based Data Warehouses
To overcome these challenges, businesses have transitioned from on-premise data warehouses to cloud-based solutions. Cloud data warehouses, such as Snowflake, Google BigQuery, and Amazon Redshift, offer:
- Elastic Scalability – Compute and storage resources scale on demand, eliminating the need for expensive on-premise hardware.
- Faster Data Processing – Cloud warehouses support massively parallel processing (MPP) and serverless architectures, significantly reducing query times.
- Cost Efficiency – Pay-as-you-go models optimize costs by charging only for the resources used.
- Seamless Integration – Cloud platforms easily integrate with various data sources, APIs, and AI-driven analytics tools.
- Enhanced Security and Compliance – Leading cloud providers offer robust security frameworks, including encryption, access controls, and regulatory compliance support.
The Role of AI in Modernizing Data Warehousing
AI is revolutionizing data warehousing by automating complex processes, enhancing performance, and enabling predictive analytics. Key AI-driven advancements include:
- Automated Data Ingestion and Transformation – AI optimizes ETL/ELT workflows, reducing manual intervention and improving data freshness.
- Intelligent Query Optimization – Machine learning algorithms enhance query performance by predicting optimal execution paths and indexing strategies.
- Real-Time Data Processing – AI enables continuous data streaming, ensuring instant access to critical business insights.
- Enhanced Data Quality and Governance – AI-driven anomaly detection and data cleansing ensure accuracy and compliance.
- Predictive and Prescriptive Analytics – AI enables businesses to move beyond historical reporting to advanced forecasting and decision-making models.
As AI continues to advance, data warehousing will become even more autonomous, adaptive, and intelligent. Organizations that embrace AI-driven data warehousing strategies will be better equipped to handle the complexities of modern data ecosystems and maintain a competitive edge in 2025 and beyond.
AI-Powered Data Warehousing: Key Capabilities
As data volumes grow and business intelligence demands become more complex, AI-powered data warehousing has emerged as a game-changer. By integrating AI-driven automation, optimization, and intelligence, modern data warehouses are transforming into high-performance ecosystems capable of delivering real-time insights, predictive analytics, and enhanced data governance.
Automated Data Ingestion & Processing
One of the most significant advantages of AI in data warehousing is the automation of data ingestion and processing. AI-driven ETL (Extract, Transform, Load) and ELT (Extract, Load, Transform) pipelines streamline data integration by eliminating manual bottlenecks and optimizing workflows.
- Faster Data Integration – AI automates data mapping, schema detection, and transformation processes, significantly reducing the time required to onboard new data sources.
- Seamless Handling of Structured and Unstructured Data – Traditional data warehouses struggle with unstructured data (e.g., images, text, logs). AI-powered solutions leverage Natural Language Processing (NLP) and machine learning to classify, process, and extract insights from diverse data types.
- Self-Optimizing Pipelines – Machine learning models monitor ingestion patterns and dynamically optimize performance, ensuring efficient resource utilization.
Real-Time Analytics & Predictive Insights
Businesses require real-time insights to make informed decisions, and AI plays a crucial role in enhancing analytical capabilities. AI models enhance query performance, analytics processing, and predictive intelligence to deliver faster, more accurate insights.
- AI-Driven Query Acceleration – By analyzing query history and user behavior, AI optimizes execution paths and caching strategies for rapid responses.
- Streaming Analytics for Real-Time Decision-Making – AI-powered systems process and analyze live data streams, allowing businesses to react instantly to market changes, customer behavior, and operational anomalies.
- Predictive Analytics for Strategic Planning – AI leverages historical data and real-time inputs to predict trends, optimize inventory, detect fraud, and drive data-driven decision-making.
Data Governance & Quality Management
Maintaining data quality, integrity, and compliance is a critical challenge for organizations. AI automates governance processes by detecting anomalies, ensuring consistency, and enforcing security policies.
- Automated Data Cleansing & Consistency Checks – AI algorithms identify missing, duplicate, or inconsistent data points and rectify errors to maintain data accuracy.
- Anomaly Detection for Data Integrity – Machine learning models continuously monitor data streams, flagging irregularities that may indicate errors, fraud, or cyber threats.
- Regulatory Compliance Enforcement – AI ensures adherence to data privacy regulations (e.g., GDPR, CCPA) by automating access controls, encryption, and audit trails.
Smart Query Optimization
AI-driven query optimization techniques enhance performance while reducing computational costs. By leveraging intelligent indexing, caching, and execution planning, AI ensures faster and more cost-effective analytics.
- AI-Based Indexing & Query Acceleration – AI predicts query patterns and dynamically adjusts indexing structures to improve retrieval speeds.
- Adaptive Resource Allocation – Machine learning models allocate compute resources based on workload demands, optimizing cost efficiency.
- Reduced Computational Overhead – AI minimizes redundant queries, applies intelligent caching, and prioritizes critical workloads to improve response times.
The Business Benefits of AI-Integrated Data Warehousing
AI-driven data warehousing is not just about technological advancements; it delivers tangible business benefits that drive growth, efficiency, and security. Organizations leveraging AI-powered data warehouses gain a competitive edge by making faster decisions, optimizing operations, and ensuring data integrity at scale.
Improved Decision-Making: Faster Access to Insights and Trends
In today’s fast-paced business environment, real-time decision-making is a necessity. AI enhances data analytics by:
- Delivering Instant Insights – AI-powered data warehouses process and analyze data in real time, allowing businesses to respond quickly to market changes, customer demands, and operational shifts.
- Identifying Patterns & Trends – Machine learning algorithms detect patterns in vast datasets, helping organizations predict demand, optimize pricing strategies, and uncover growth opportunities.
- Enhancing Data Visualization & Reporting – AI automates reporting, generates dynamic dashboards, and provides actionable recommendations, making data-driven decision-making easier and more efficient.
Operational Efficiency: Reduced Data Processing Times and Lower Infrastructure Costs
AI-driven automation significantly improves the speed and efficiency of data warehousing operations:
- Optimized ETL/ELT Processes – AI eliminates bottlenecks in data extraction, transformation, and loading, reducing the time needed to process massive datasets.
- Lower Infrastructure Costs – AI-powered optimization techniques, such as intelligent caching and dynamic workload management, minimize computational overhead and storage costs.
- Automated Maintenance & Performance Tuning – AI continuously monitors database health, optimizes queries, and automatically scales resources, reducing IT workload and operational costs.
Enhanced Security: AI-Driven Threat Detection and Access Control
As cyber threats become more sophisticated, AI-driven security features in modern data warehouses provide robust protection:
- Anomaly Detection & Fraud Prevention – AI continuously analyzes data access patterns to detect anomalies, unauthorized access, and potential security breaches in real time.
- Automated Access Controls & Compliance Enforcement – AI enhances identity and access management (IAM) by automating permissions, encryption, and audit trails to ensure compliance with regulations like GDPR, CCPA, and SOC 2.
- Proactive Threat Mitigation – AI-powered cybersecurity tools predict and neutralize potential threats before they can compromise data integrity.
Scalability & Flexibility: AI Adapting to Dynamic Business Needs and Growing Datasets
With data volumes expanding rapidly, AI ensures seamless scalability and adaptability:
- Elastic Scaling on Demand – AI dynamically adjusts storage and compute resources based on data workloads, ensuring optimal performance without unnecessary costs.
- Support for Multi-Cloud & Hybrid Environments – AI-powered data warehousing solutions integrate seamlessly across on-premise, cloud, and hybrid infrastructures, offering flexibility for enterprises with diverse IT landscapes.
- Future-Proofing Against Growth & Complexity – AI continuously learns and evolves, optimizing data pipelines, query execution, and workload distribution to accommodate future business needs.
Key Technologies Enabling AI in Data Warehousing
The integration of AI into data warehousing is powered by a combination of cloud computing, machine learning frameworks, and automation tools that enhance data processing, analytics, and governance. These technologies ensure scalability, efficiency, and seamless AI integration into modern data warehouses.
1. Cloud-Based Platforms: The Foundation of AI-Driven Data Warehousing
Cloud-based data warehouses provide the infrastructure needed to support AI-driven analytics, real-time processing, and scalability. Leading platforms include:
- Snowflake – Offers automatic scaling, multi-cloud compatibility, and built-in AI-driven query optimization.
- Google BigQuery – A serverless, AI-enhanced data warehouse with integrated machine learning (BigQuery ML) for predictive analytics.
- Amazon Redshift – Leverages machine learning-based query acceleration and integrates seamlessly with AWS AI/ML services.
Cloud platforms enable organizations to ingest, process, and analyze vast amounts of structured and unstructured data efficiently, ensuring AI models have access to high-quality, real-time data.
2. AI-Powered Data Processing Tools: Optimizing Data Pipelines
AI-driven data processing and analytics frameworks improve the efficiency and speed of data ingestion, transformation, and querying. Key tools include:
- Databricks – Built on Apache Spark, Databricks offers AI-driven data engineering, analytics, and ML model training in a unified environment.
- Apache Spark with MLlib – A distributed data processing framework that includes built-in machine learning capabilities for AI-enhanced ETL and analytics.
- Presto and Trino – AI-optimized distributed SQL engines that accelerate data retrieval across large datasets.
These tools enhance data pipeline automation, improve query execution speeds, and enable AI-driven transformations, making them essential for modern AI-powered data warehousing.
3. Advanced AI Frameworks: Driving Data Science Integration
AI-powered data warehouses seamlessly integrate with machine learning and deep learning frameworks for predictive analytics, anomaly detection, and automated decision-making. Key frameworks include:
- TensorFlow – A deep learning library that enables AI models to process large-scale data for advanced analytics and automation.
- PyTorch – A flexible and efficient AI framework widely used for real-time ML model development and AI-driven data science applications.
- H2O.ai – An AI-driven machine learning platform that enhances predictive analytics and automated model training.
By incorporating these frameworks, businesses can train, deploy, and scale AI models directly within their data warehouses, enhancing forecasting, fraud detection, and intelligent automation.
4. The Role of DataOps and MLOps in Modern AI-Driven Warehouses
As AI adoption grows, DataOps and MLOps ensure efficient management and deployment of AI models within data warehousing environments:
- DataOps – Focuses on automating and optimizing data workflows, improving data quality, and enabling seamless integration between AI models and business applications.
- MLOps – Ensures continuous monitoring, deployment, and optimization of AI models within data warehouses, enhancing performance and scalability.
By integrating DataOps and MLOps, organizations can accelerate AI adoption, streamline data science workflows, and ensure models remain accurate and efficient as data landscapes evolve.
Overcoming Challenges in AI-Powered Data Warehousing
While AI-driven data warehousing offers significant advantages, businesses must navigate several challenges to ensure seamless implementation and optimal performance. Key obstacles include data integration complexities, AI model accuracy and bias, and cost considerations. Addressing these challenges is crucial for unlocking the full potential of AI-powered data warehouses.
1. Data Silos & Integration Complexities: How AI Bridges Disparate Data Sources
One of the biggest challenges organizations face is integrating structured and unstructured data from multiple sources while ensuring consistency and accuracy. Traditional data warehouses struggle with fragmented data ecosystems, leading to inefficiencies and incomplete insights. AI helps bridge these gaps by:
- Automating Data Ingestion – AI-driven ETL/ELT processes streamline data extraction, transformation, and loading, eliminating manual integration bottlenecks.
- Intelligent Data Mapping & Harmonization – AI algorithms analyze schema differences across databases and automatically map data fields, ensuring consistency across disparate sources.
- Data Virtualization & Federated Queries – AI-powered warehouses support federated queries across multiple databases and cloud environments, enabling a unified view of data without costly migrations.
By leveraging AI for data integration, businesses can eliminate silos, enhance data accessibility, and ensure a seamless flow of information across departments.
2. AI Model Accuracy & Bias: Ensuring Transparency and Accountability in AI Predictions
AI models play a critical role in predicting trends, detecting anomalies, and optimizing queries, but they are susceptible to accuracy issues and bias. Ensuring trustworthy AI-driven insights requires:
- Continuous Model Monitoring & Validation – AI-powered data warehouses integrate MLOps frameworks to monitor model performance, detect drift, and retrain models as needed.
- Bias Detection & Explainable AI (XAI) – Machine learning interpretability tools help identify and mitigate biases in AI predictions, ensuring fairness and accountability.
- Human-in-the-Loop (HITL) Approach – AI-enhanced data warehouses can incorporate human oversight to validate and refine AI-driven insights, improving decision-making accuracy.
By implementing transparency measures and continuous validation, businesses can trust AI-powered insights and minimize the risk of biased or misleading predictions.
3. Cost & ROI Considerations: Measuring the Success of AI-Driven Data Warehouses
AI-powered data warehousing requires significant investment in cloud infrastructure, AI tools, and operational workflows. To ensure a strong return on investment (ROI), organizations should:
- Optimize Cost Efficiency – AI-driven auto-scaling and intelligent workload management help minimize unnecessary cloud expenses while maintaining performance.
- Define Key Performance Indicators (KPIs) – Businesses should track KPIs such as query performance improvements, data processing time reduction, and cost savings from automation.
- Measure Business Impact – AI-powered warehouses should drive measurable business outcomes, such as faster decision-making, improved customer analytics, and revenue growth.
By establishing clear metrics and optimizing AI resource allocation, businesses can ensure that AI-driven data warehousing delivers a strong financial and operational ROI.
Future Trends: What to Expect Beyond 2025
1. The Convergence of AI, IoT, and Data Warehousing
AI-powered data warehouses will increasingly integrate with IoT devices, enabling real-time ingestion and analysis of sensor data. This will drive smarter decision-making in industries like manufacturing, healthcare, and logistics.
2. Real-Time Adaptive Analytics & Hyperautomation
AI will enable self-optimizing data warehouses, where real-time adaptive analytics and hyperautomation refine query execution, optimize resources, and deliver instant insights without manual intervention.
3. AI-Powered Self-Service Analytics for Non-Technical Users
Business users will gain direct access to AI-driven insights through natural language processing (NLP) and no-code analytics platforms, democratizing data analytics across enterprises.
4. Generative AI for Data Interpretation & Reporting
Generative AI will automate data storytelling, dashboard creation, and report generation, providing personalized insights and context-driven analysis tailored to business needs.
AI-driven data warehousing will continue evolving, making real-time, intelligent, and user-friendly analytics the new standard for data-driven organizations.
Conclusion
AI-powered data warehousing is no longer a futuristic concept—it is a necessity for businesses aiming to stay competitive, agile, and data-driven. By integrating AI with modern data warehouses, organizations can eliminate data silos, accelerate real-time analytics, enhance security, and drive smarter decision-making. As we move beyond 2025, AI will continue to revolutionize data management through hyperautomation, self-service analytics, and AI-powered reporting, making it imperative for businesses to adopt these innovations.
Unlock the Power of AI-Driven Data Warehousing with Datahub Analytics
At Datahub Analytics, we help businesses build, optimize, and scale AI-powered data warehouses that deliver real-time insights, predictive analytics, and cost efficiency. Whether you are transitioning to the cloud, modernizing your data architecture, or looking to integrate AI-driven automation, our team has the expertise to guide you.
Let’s discuss how AI-powered data warehousing can accelerate your business success. Contact Datahub Analytics now and take the next step toward an intelligent, future-ready data infrastructure!