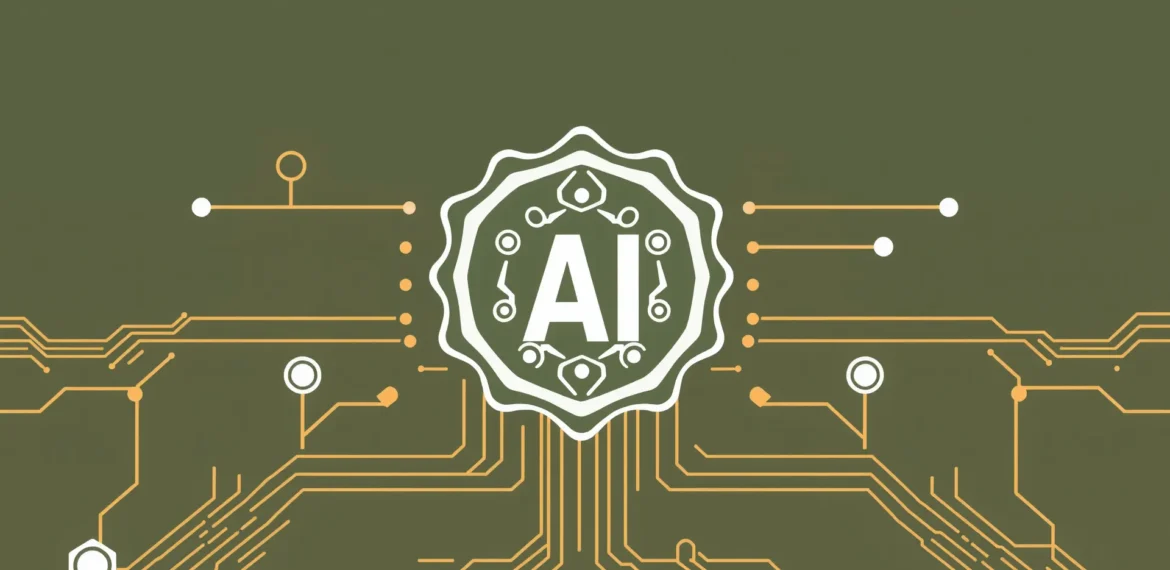
Intelligent Resource Allocation: AI Strategies in Infrastructure Automationย
Intelligent Resource Allocation: AI Strategies in Infrastructure Automationย
Modern IT and cloud infrastructure have become increasingly complex, requiring businesses to manage vast networks, storage, and computing resources efficiently. As enterprises scale, manual resource allocation struggles to keep pace with dynamic demands, often leading to inefficiencies, increased costs, and operational errors.
Traditional approaches rely on human decision-making, static provisioning, and reactive problem-solving. These methods frequently result in over-provisioned resources that inflate expenses or underutilized assets that hinder performance. Additionally, unpredictable workloads, system failures, and the need for real-time optimization make manual management unsustainable.
AI-driven automation is transforming infrastructure management by enabling intelligent resource allocation. Machine learning models analyze usage patterns, predict demand, and optimize workloads in real time. Automated systems ensure efficient distribution, self-healing capabilities, and proactive scaling, reducing costs while enhancing performance and reliability. Businesses leveraging AI-driven infrastructure automation can achieve greater agility, resilience, and operational efficiency.
The Need for AI in Infrastructure Automation
As businesses expand and digital transformation accelerates, IT infrastructure must support increasing demands for scalability, agility, and resilience. Organizations require real-time adaptability to fluctuating workloads, seamless scaling of resources, and the ability to recover from failures without disruptions. Manual infrastructure management often falls short, leading to inefficiencies and performance bottlenecks.
Rising infrastructure costs and operational inefficiencies pose another challenge. Overprovisioning wastes resources, while underutilization results in unnecessary expenses. Traditional capacity planning lacks real-time adaptability, leading to higher operational costs and wasted computing power. IT teams struggle to balance cost-efficiency with performance optimization, especially in cloud and hybrid environments.
Challenges in traditional resource allocation methods stem from static provisioning, reactive problem resolution, and human error. Manual workflows slow down response times and limit the ability to optimize workloads dynamically. Without intelligent automation, organizations face delays, inconsistent performance, and a higher risk of system failures.
AI-driven infrastructure automation addresses these challenges by optimizing IT infrastructure performance through predictive analytics, real-time workload management, and autonomous decision-making. AI models assess patterns, anticipate future demands, and allocate resources dynamically. By integrating AI into infrastructure operations, businesses can achieve cost savings, maximize efficiency, and ensure seamless performance at scale.
Key AI-Driven Strategies for Intelligent Resource Allocation
3.1 Predictive Resource Management
AI-powered predictive analytics leverages historical data to forecast future resource demand accurately. By analyzing usage patterns, AI models anticipate workload spikes, ensuring that resources are scaled dynamically to prevent overprovisioning or underutilization. This proactive approach reduces operational costs and optimizes performance.
๐น Dynamic Scaling: AI adjusts computing power, storage, and network bandwidth based on predicted demand, preventing resource wastage.
๐น Cloud Cost Optimization: AI-driven predictions help allocate cloud instances efficiently, reducing unnecessary expenses.
๐ Case Study: A global enterprise implemented AI-powered predictive resource management for its cloud infrastructure. By analyzing traffic trends and application workloads, AI automated scaling, reducing cloud expenses by 30% while improving response times during peak demand.
3.2 Autonomous Workload Distribution
AI-driven workload orchestration ensures efficient distribution of computing tasks across cloud, on-prem, and hybrid environments. Intelligent scheduling algorithms analyze performance metrics and workload priorities to allocate resources dynamically.
๐น Workload Balancing: AI monitors CPU, memory, and storage usage in real time to distribute tasks across available infrastructure efficiently.
๐น Latency Reduction: Adaptive scheduling minimizes processing delays by optimizing resource allocation.
๐ Example: A DevOps team used AI-driven workload orchestration to manage containerized applications across multiple cloud providers. AI ensured high availability, reduced latency, and automated resource adjustments, eliminating manual intervention.
3.3 Self-Healing Infrastructure
AI enables infrastructure to detect anomalies, resolve failures, and maintain optimal performance without human intervention. Self-healing capabilities allow systems to recover from unexpected disruptions automatically.
๐น Anomaly Detection: AI continuously monitors system logs and network activity to detect potential failures before they occur.
๐น Automated Recovery: AI triggers failover mechanisms, rerouting workloads to healthy resources, ensuring uninterrupted operations.
๐ Case Study: A financial institution integrated AI-driven self-healing mechanisms into its IT infrastructure. AI proactively identified security threats, mitigated system crashes, and reduced downtime by 40%, improving business continuity.
3.4 AI in Network Optimization
AI-driven network management optimizes bandwidth usage, traffic routing, and predictive maintenance, ensuring seamless connectivity. Machine learning models analyze traffic patterns to adjust network configurations dynamically.
๐น Traffic Optimization: AI intelligently routes data packets to reduce congestion and improve network efficiency.
๐น Predictive Maintenance: AI detects signs of hardware degradation, allowing preemptive repairs before failures occur.
๐ Example: A telecom provider deployed AI-driven network monitoring to enhance service reliability. AI-based predictions prevented outages, reduced latency, and improved user experience, leading to a 25% increase in network uptime.
3.5 Intelligent Energy Optimization
AI-driven energy management solutions enhance sustainability by optimizing power consumption in data centers and cloud environments. By leveraging AI insights, businesses can reduce carbon footprints while lowering operational costs.
๐น Energy Efficiency: AI minimizes redundant power usage by dynamically adjusting resource utilization.
๐น Smart Cooling Systems: AI monitors temperature and optimizes cooling processes, reducing energy waste.
๐ Case Study: A hyperscale data center used AI-driven energy optimization to manage its power distribution. AI-controlled cooling systems and workload shifts led to a 20% reduction in energy consumption while maintaining peak performance.
Benefits of AI-Powered Infrastructure Automation
1. Cost Efficiency
AI-driven automation optimizes resource utilization, ensuring that infrastructure operates at peak efficiency without unnecessary expenditure. By dynamically scaling resources based on real-time demand, AI eliminates overprovisioning and reduces cloud and data center costs.
๐น Reduced Waste: AI minimizes idle resources, cutting down operational expenses.
๐น Smart Budget Allocation: AI-driven insights help optimize infrastructure investments based on actual usage patterns.
๐ Example: A multinational enterprise used AI-powered infrastructure management to optimize cloud spending, reducing operational costs by 30% while maintaining system reliability.
2. Performance Enhancement
AI continuously monitors infrastructure health and dynamically adjusts configurations to maximize performance. By predicting system failures and proactively addressing issues, AI-driven automation ensures higher uptime and responsiveness.
๐น Intelligent Load Balancing: AI distributes workloads efficiently to prevent bottlenecks.
๐น Predictive Maintenance: AI identifies hardware/software issues before they impact operations.
๐ Example: A SaaS company integrated AI for real-time workload distribution, improving application response times by 40% and reducing server crashes.
3. Scalability & Flexibility
AI enables infrastructure to scale dynamically based on real-time workload demands, ensuring businesses can expand or contract resources without manual intervention. This adaptability is crucial for handling traffic spikes, seasonal fluctuations, and business growth.
๐น Elastic Resource Scaling: AI provisions additional capacity during peak usage and scales down during low demand.
๐น Seamless Integration: AI-driven automation ensures smooth performance across multi-cloud and hybrid environments.
๐ Example: An e-commerce platform leveraged AI-based infrastructure scaling during high-traffic sales events, preventing slowdowns and ensuring seamless customer experiences.
4. Reduced Human Intervention
AI automation minimizes reliance on manual processes, reducing human errors and operational inefficiencies. By handling repetitive tasks, AI allows IT teams to focus on strategic initiatives rather than routine maintenance.
๐น Automated Incident Response: AI detects and resolves infrastructure issues autonomously.
๐น Efficient IT Operations: AI-driven workflows streamline administration, reducing workload on IT teams.
๐ Example: A financial institution used AI for automated security patching and system monitoring, reducing IT workload by 50% while improving compliance.
5. Sustainability
AI-driven infrastructure automation supports energy-efficient IT operations, reducing environmental impact while optimizing costs. AI ensures minimal power usage and enhances sustainability in cloud computing and data centers.
๐น Energy-Efficient Resource Allocation: AI optimizes power consumption by intelligently managing workloads.
๐น Smart Cooling & Green IT: AI-controlled cooling systems reduce energy waste in data centers.
๐ Example: A cloud provider implemented AI-driven energy management, cutting power consumption by 20% and improving its sustainability footprint.
Challenges and Considerations
1. Data Privacy & Security Risks
AI models rely on vast amounts of infrastructure data for optimization, raising concerns about data privacy, security, and potential vulnerabilities. Unauthorized access or misconfigurations in AI-driven automation can lead to breaches, exposing sensitive enterprise data.
๐น Data Protection Measures: Encryption, access controls, and AI-driven security monitoring help mitigate risks.
๐น AI Bias & Security Threats: AI models must be regularly audited to prevent bias, adversarial attacks, or unauthorized modifications.
๐ Example: A financial institution implementing AI-driven infrastructure monitoring had to adopt strict encryption and compliance policies to prevent data leaks and maintain regulatory adherence.
2. Integration Complexity
Organizations with legacy IT infrastructure often face challenges when integrating AI-driven automation. Many legacy systems lack APIs or compatibility with AI-driven tools, making seamless adoption difficult.
๐น Legacy System Compatibility: AI solutions must support hybrid IT environments, integrating cloud, on-premise, and legacy components.
๐น Incremental AI Adoption: A phased approach, starting with AI-enhanced monitoring and automation in non-critical systems, helps reduce disruption.
๐ Example: A telecom provider introduced AI-driven network optimization by first deploying AI on cloud-based systems before extending automation to legacy infrastructure, ensuring smooth transition.
3. Skill Gap
The adoption of AI-powered infrastructure automation requires expertise in AI, data analytics, and IT operations. However, many organizations lack skilled personnel who can effectively implement and manage AI-driven systems.
๐น Upskilling & Training: Businesses must invest in AI and automation training for IT teams.
๐น Managed AI Services: Leveraging external AI experts or managed services can bridge the skill gap.
๐ Example: A global enterprise partnered with an AI consulting firm to accelerate AI-based infrastructure automation while training internal teams to manage and scale operations.
4. Compliance & Governance
Regulatory frameworks governing data privacy, cybersecurity, and IT governance require organizations to ensure AI-driven infrastructure automation aligns with compliance mandates. AI systems must be transparent, auditable, and aligned with industry regulations.
๐น Regulatory Adherence: Organizations must comply with GDPR, ISO 27001, NIST, and other security standards when using AI for infrastructure automation.
๐น Explainable AI (XAI): AI models should be transparent and provide insights into how decisions are made to avoid compliance risks.
๐ Example: A healthcare provider integrating AI-driven automation ensured compliance with HIPAA by deploying AI models with built-in audit trails and real-time monitoring.
Partner with Datahub Analytics for AI-Driven Infrastructure Automation
Unlock the full potential of AI-powered infrastructure automation with Datahub Analytics. Our expertise in AI, cloud automation, and data-driven insights helps businesses streamline operations, enhance scalability, and optimize resource allocation.
Why Choose Datahub Analytics?
โ
AI-Driven Optimization: Leverage cutting-edge AI models for predictive resource management, workload automation, and self-healing infrastructure.
โ
Seamless Integration: Implement AI automation across cloud, on-premise, and hybrid environments with minimal disruption.
โ
Cost Savings & Efficiency: Reduce operational costs while maximizing performance and uptime.
โ
Security & Compliance: Ensure AI-driven infrastructure aligns with industry regulations and cybersecurity best practices.
โ
Expert AI Consultants: Bridge the skill gap with our AI specialists, enabling smooth adoption and training for your IT teams.
Conclusion
AI-driven infrastructure automation is revolutionizing IT operations by enabling intelligent resource allocation, predictive scaling, autonomous workload management, and self-healing capabilities. Businesses that embrace AI-powered automation can achieve cost efficiency, enhanced performance, scalability, reduced manual intervention, and sustainability.
However, challenges such as data privacy, integration complexity, skill gaps, and regulatory compliance must be carefully managed to ensure a seamless transition. Organizations that adopt AI strategically can future-proof their IT infrastructure, reduce downtime, and optimize resource utilization in real time.
Partnering with Datahub Analytics ensures a smooth and secure adoption of AI-driven automation, helping businesses stay ahead in an increasingly digital and competitive landscape. Now is the time to leverage AI for infrastructure efficiency and scalabilityโget in touch with us today to explore customized AI solutions for your enterprise.