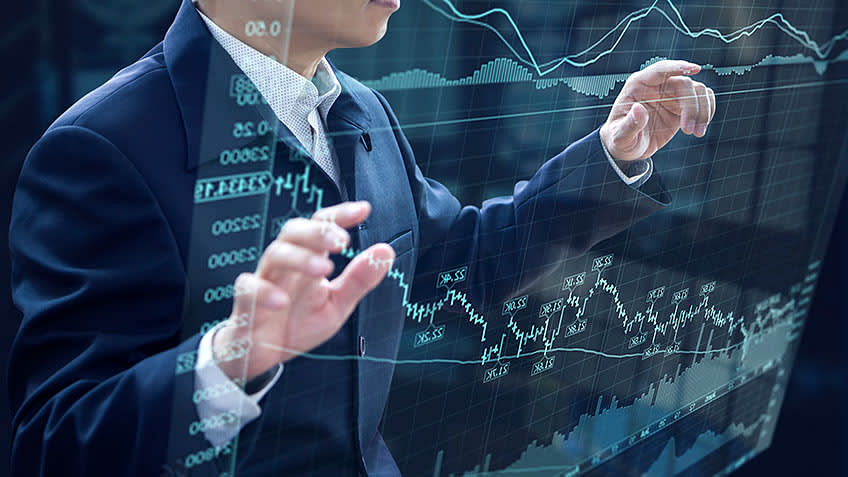
Leading Data Analytics Initiatives
Leading Data Analytics Initiatives
I. Introduction
In the ever-evolving landscape of modern business, data has emerged as the lifeblood that fuels informed decision-making and strategic planning. Within this data-driven paradigm, organizations are increasingly relying on Data Analytics Initiatives to gain actionable insights, optimize processes, and stay ahead of the competition. Understanding the intricacies of these initiatives is pivotal for any business aiming to thrive in the digital age.
Definition of Data Analytics Initiatives
At its core, Data Analytics Initiatives refer to the systematic use of statistical analysis, data mining, predictive modeling, and other analytical techniques to interpret data and extract valuable insights. These initiatives are tailored processes designed to transform raw data into meaningful patterns, enabling businesses to make data-driven decisions.
Importance of Data Analytics in Modern Business
In the contemporary business landscape, data analytics serves as the linchpin for success. Businesses can leverage data analytics to understand customer behavior, forecast trends, streamline operations, and enhance overall efficiency. By harnessing the power of data, organizations can make strategic decisions based on evidence and patterns rather than mere intuition, leading to improved outcomes and heightened competitiveness.
Significance of Aligning Analytics Initiatives with Business Goals
Aligning Analytics Initiatives with Business Goals is akin to calibrating a compass: it ensures that the organization is moving in the right direction. When analytics initiatives are in harmony with business objectives, they become catalysts for growth. Such alignment enables businesses to focus their analytical efforts on areas that directly impact revenue, customer satisfaction, and operational excellence, thereby maximizing the value derived from data analytics investments.
In the subsequent sections, we will dissect the challenges faced in implementing data analytics initiatives, elaborate on the best practices that can pave the way for success, and discuss strategies for aligning these initiatives seamlessly with the overarching business objectives. Join us on this insightful journey into the realm of data analytics, where challenges are met with innovation, and best practices light the path toward transformative business outcomes.
II. Challenges in Leading Data Analytics Initiatives
In the pursuit of leveraging data analytics for strategic advantage, organizations encounter several challenges that can significantly impact the success of their initiatives. Understanding and mitigating these challenges are crucial for the effective implementation of data analytics projects.
A. Lack of Clear Business Objectives
Importance of Defining Clear and Measurable Goals
Clear, well-defined objectives act as the guiding stars for any data analytics initiative. They provide a roadmap, outlining what the organization aims to achieve through its analytical efforts. Clear objectives help in focusing the analytics team’s efforts, ensuring that resources are utilized efficiently toward achieving specific, measurable outcomes.
Impact of Ambiguous Objectives on Analytics Projects
Ambiguity in objectives leads to confusion and inefficiency. When objectives lack clarity, analytics projects can become misguided, leading to inconclusive results. Ambiguous goals make it challenging to measure progress and evaluate the success of the analytics initiative, hindering the organization’s ability to derive actionable insights.
B. Data Quality and Integration Issues
Challenges in Data Collection and Cleaning
Raw data is often riddled with inconsistencies, errors, and missing values. Data cleaning, a crucial step in the analytics process, becomes challenging when dealing with vast and diverse datasets. Inaccurate or incomplete data can skew analysis results, leading to misguided business decisions.
Integrating Data from Multiple Sources
Organizations typically have data stored across various platforms and systems. Integrating data from disparate sources is complex, involving technical challenges and compatibility issues. Inconsistent data formats and structures make it difficult to create a unified view, hampering the ability to gain comprehensive insights.
C. Skilled Workforce Shortage
Demand for Data Professionals
The rising demand for data professionals, including data scientists, analysts, and engineers, surpasses the available talent pool. As businesses increasingly invest in data analytics, the competition for skilled workforce intensifies, making it challenging for organizations to recruit and retain top talent.
Strategies for Overcoming Skill Gaps
To address skill shortages, organizations can invest in training and upskilling programs for existing employees. Collaboration with educational institutions and online learning platforms can provide avenues for continuous learning. Additionally, fostering a culture of knowledge sharing and mentorship within the organization can help bridge skill gaps.
D. Regulatory and Ethical Challenges
Data Privacy Regulations (e.g., GDPR, CCPA)
Stringent data privacy regulations, such as the General Data Protection Regulation (GDPR) and the California Consumer Privacy Act (CCPA), impose legal obligations on organizations regarding data handling and user privacy. Non-compliance can result in severe penalties, necessitating meticulous adherence to these regulations.
Ethical Implications of Data Analytics
Ethical considerations arise concerning the use of data, especially in sensitive areas like profiling and decision-making. Ethical dilemmas may surface when algorithms exhibit biases or when data-driven decisions impact individuals or communities negatively. Addressing these ethical challenges requires transparency, fairness, and a commitment to responsible data practices.
In the face of these challenges, organizations must develop comprehensive strategies and adopt best practices to navigate the complexities of data analytics initiatives successfully. By addressing these hurdles proactively, businesses can unlock the full potential of their data, driving innovation and informed decision-making.
III. Best Practices for Leading Data Analytics Initiatives
Navigating the intricate landscape of data analytics initiatives demands a strategic approach and adherence to best practices. These practices not only mitigate challenges but also lay the foundation for successful, impactful analytics projects.
A. Defining Clear Business Objectives
Involving Stakeholders in Goal Setting:
Engaging key stakeholders from different departments ensures that diverse perspectives are considered when setting objectives. Involving stakeholders fosters a sense of ownership and collective responsibility for achieving the defined goals, aligning the entire organization toward a common purpose.
SMART Criteria for Setting Objectives (Specific, Measurable, Achievable, Relevant, Time-bound):
Adhering to SMART criteria ensures that objectives are well-defined and actionable. Specific objectives clarify what needs to be achieved, measurable criteria provide tangible metrics for success, achievable goals set realistic expectations, relevant objectives align with the organization’s mission, and time-bound goals establish clear deadlines, fostering accountability and focus.
B. Ensuring Data Quality and Integration
Data Cleaning and Preprocessing Techniques:
Implementing robust data cleaning and preprocessing techniques is paramount to ensure the accuracy and reliability of analysis. Techniques like outlier detection, imputation for missing data, and normalization enhance the quality of the dataset, laying a solid foundation for meaningful analysis and interpretation.
Implementing Data Integration Platforms:
Deploying advanced data integration platforms streamlines the process of combining data from diverse sources. These platforms facilitate seamless data flow, transforming raw data into a unified, coherent dataset. Integration platforms automate repetitive tasks, reduce errors, and enhance efficiency, enabling organizations to work with comprehensive, reliable datasets.
C. Building a Skilled Workforce
Training and Upskilling Existing Employees:
Investing in training programs equips existing employees with the necessary skills to handle data analytics tasks. Continuous training keeps the workforce updated with the latest tools and techniques, fostering a culture of learning within the organization. Upskilled employees contribute to improved productivity and innovation in analytics projects.
Collaborating with Educational Institutions and Training Centers:
Collaborations with educational institutions and specialized training centers provide access to a pool of fresh talent. Internship programs, workshops, and partnerships with academic institutions not only serve as talent pipelines but also encourage knowledge exchange between academia and industry, promoting innovation and skill development.
D. Addressing Regulatory and Ethical Concerns
Staying Updated with Data Privacy Laws:
Regulations surrounding data privacy and security are constantly evolving. Staying informed about the latest laws, such as GDPR, CCPA, and others relevant to the organization’s jurisdiction, is imperative. Compliance with these laws safeguards customer data, protects the organization from legal repercussions, and fosters trust with stakeholders.
Implementing Ethical Guidelines and Practices in Analytics Projects:
Establishing ethical guidelines ensures responsible use of data. Ethical considerations should be integrated into every stage of analytics projects, from data collection to algorithm development and result interpretation. By prioritizing fairness, transparency, and accountability, organizations uphold ethical standards, fostering trust and credibility in their analytics initiatives.
By adopting these best practices, organizations can enhance the effectiveness of their data analytics initiatives, transforming challenges into opportunities for growth, innovation, and sustainable success. These practices not only empower organizations to harness the full potential of their data but also enable them to make ethical, informed decisions that positively impact both the business and its stakeholders.
IV. Strategies for Aligning Analytics Initiatives with Business Goals
In the dynamic realm of data analytics, aligning initiatives with overarching business objectives is essential for driving meaningful outcomes and sustainable growth. Implementing effective strategies ensures that analytics efforts directly contribute to the organization’s success and strategic vision.
A. Collaboration Between IT and Business Departments
Bridging the Gap Between IT and Business Language:
Establishing a shared vocabulary between IT professionals and business stakeholders is fundamental. Clear communication bridges the divide, enabling IT teams to understand business needs and translate them into actionable technical requirements. By speaking a common language, collaboration becomes seamless, ensuring that analytical solutions directly address business challenges.
Establishing Cross-Functional Teams:
Creating cross-functional teams that comprise members from IT, business, data analytics, and other relevant departments promotes diverse perspectives. These teams foster collaboration and innovation by integrating insights from different domains. Collaborative problem-solving enhances the quality of analytics initiatives, aligning them more closely with multifaceted business goals.
B. Continuous Communication with Stakeholders
Regular Updates on Analytics Projects:
Transparent communication through regular updates keeps stakeholders informed about project progress, challenges, and achievements. By sharing milestones and insights, stakeholders gain a deeper understanding of the analytics initiatives. Timely updates foster trust and enable stakeholders to provide valuable input, ensuring that the projects remain on course.
Gathering Feedback and Making Necessary Adjustments:
Actively soliciting feedback from stakeholders, both internal and external, is vital. Feedback loops create opportunities for course correction and improvement. Listening to concerns and adapting strategies based on feedback ensures that analytics projects remain relevant and effective. Continuous refinement in response to stakeholder input strengthens the alignment between analytics initiatives and business objectives.
C. Implementing Agile and Iterative Approaches
Agile Methodologies in Data Analytics:
Agile methodologies, adapted from software development, are invaluable in the world of data analytics. Agile emphasizes iterative, incremental progress and flexibility in response to changing requirements. By breaking down analytics projects into manageable sprints, teams can quickly adapt to evolving business needs, ensuring that the initiatives remain aligned with the dynamic nature of the organization.
Importance of Iterative Prototyping and Testing:
Iterative prototyping and testing allow for the rapid development of analytical models and solutions. By continuously testing prototypes, teams can identify flaws early, enabling swift adjustments. Iterative development ensures that the end product meets business requirements effectively. Regular testing and refinement guarantee that analytics initiatives are finely tuned to support business objectives.
D. Measuring and Demonstrating ROI
Key Performance Indicators (KPIs) for Analytics Projects:
Defining and monitoring Key Performance Indicators (KPIs) specific to analytics projects is essential. KPIs should be aligned with business objectives, measuring factors such as cost savings, revenue growth, customer satisfaction, or operational efficiency. Clear, measurable KPIs provide a tangible way to assess the impact of analytics initiatives on the organization’s bottom line.
Methods for Demonstrating the Impact of Analytics Initiatives on Business Outcomes:
Implementing methods to quantify the impact of analytics initiatives is crucial for demonstrating return on investment (ROI). Comparative analysis, A/B testing, and predictive modeling can provide valuable insights into the direct influence of analytics efforts on business outcomes. Tangible evidence of positive impacts strengthens the alignment between analytics initiatives and business goals, justifying ongoing investment and fostering continuous improvement.
By incorporating these strategies, organizations can ensure that their data analytics initiatives are not just isolated technical projects but integral components of the broader business strategy. Alignment with business goals becomes a continuous, iterative process, enhancing the organization’s agility and competitiveness in the data-driven marketplace.
Conclusion
In response to these challenges, a set of robust best practices emerges as a guiding light for organizations. Defining clear, measurable objectives, ensuring data quality, building a skilled workforce, and addressing ethical and regulatory concerns form the bedrock of successful data analytics initiatives. Collaboration between IT and business, continuous communication with stakeholders, embracing agile methodologies, and measuring ROI are pivotal strategies that organizations can employ to align their analytics efforts seamlessly with overarching business goals.
The alignment of analytics initiatives with business goals is not merely a technical nicety; it’s a strategic imperative. When analytics efforts are harmonized with the broader organizational vision, they become powerful tools for growth, innovation, and enhanced decision-making. Aligning analytics with business objectives fosters a data-driven culture where insights are transformed into actionable strategies, enabling businesses to respond swiftly to market changes, customer needs, and emerging opportunities.
In the face of a data-rich future, organizations equipped with strategic data analytics initiatives are not just surviving; they are thriving, leading, and shaping the industries of tomorrow. So, let this be a call to action – invest in data analytics, align your initiatives strategically, and embark on a transformative journey that will redefine the way your organization perceives, processes, and utilizes data, paving the way for unparalleled success and innovation in the digital era.