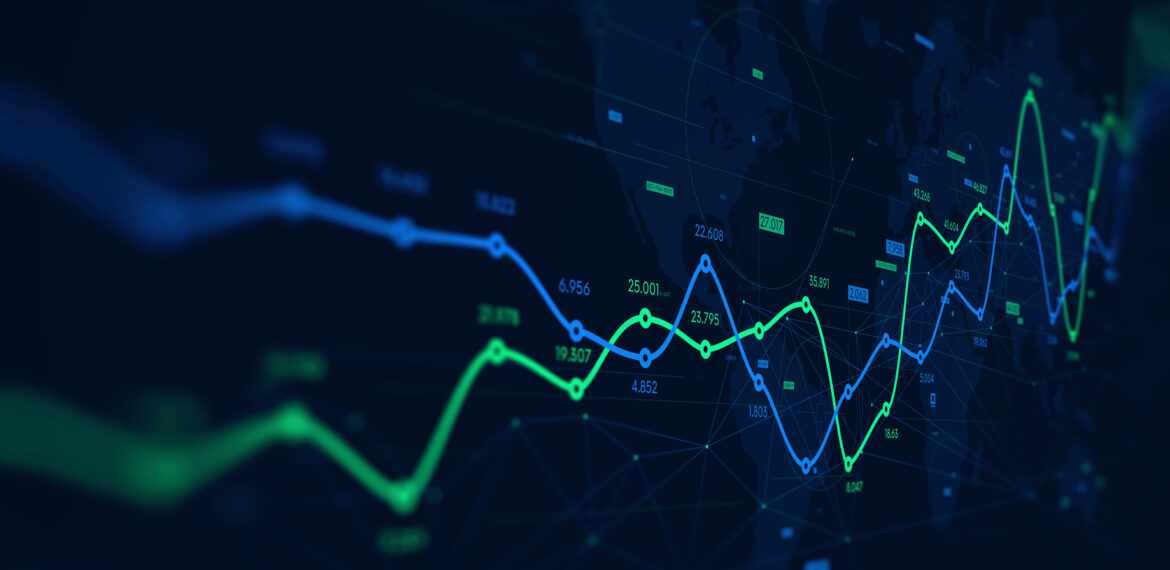
Leveraging AI for Enhanced Data Quality and Management
Leveraging AI for Enhanced Data Quality and Management
In today’s rapidly evolving digital landscape, data quality and management have emerged as critical differentiators for business success. Organizations harness vast amounts of data to drive insights, inform decision-making, and improve operational efficiency. However, managing this massive influx of data—ensuring its quality, accuracy, and reliability—remains a significant challenge.
Artificial Intelligence (AI) has emerged as a powerful solution to this challenge. By automating, streamlining, and enhancing data management tasks, AI technology can substantially improve data quality and provide actionable insights for informed decision-making.
In this detailed guide, we will explore how AI technologies are revolutionizing data quality management, outline key applications, and share insights on effective implementation.
Understanding Data Quality: Why It Matters
High-quality data means data that is accurate, consistent, timely, reliable, and relevant. Poor data quality directly impacts business operations, decision-making, customer experience, and overall organizational efficiency.
Common issues with data quality include:
- Duplicate records
- Incomplete or missing information
- Outdated or inaccurate data
- Inconsistent formatting and standards
- Misclassification or improper tagging
Each of these issues can negatively affect business decisions, customer insights, and strategic initiatives, ultimately leading to reduced profitability and lost opportunities.
The Role of AI in Improving Data Quality
Artificial Intelligence leverages advanced algorithms and machine learning techniques to address common data management challenges efficiently and effectively.
1. Data Cleansing and Preparation
AI systems automatically identify inaccuracies, redundancies, and inconsistencies. For instance:
- Duplicate Detection: Algorithms pinpoint and remove duplicate records swiftly.
- Automated Correction: AI suggests or applies corrections automatically based on historical data patterns and rules.
- Normalization: AI-driven processes standardize data formats, facilitating seamless integration across various data sources.
2. Data Integration and Consolidation
Managing data across multiple systems or platforms becomes simpler and faster with AI. Machine learning algorithms can:
- Integrate Disparate Data Sources: AI models reconcile differences in schemas and formats, automatically mapping similar fields.
- Resolve Conflicts: Intelligent reconciliation systems identify and resolve conflicting records by referencing historical resolution patterns.
3. Real-time Data Validation
AI-powered validation systems continuously monitor incoming data, promptly identifying anomalies:
- Pattern Recognition: AI algorithms recognize deviations from established data patterns.
- Anomaly Detection: Systems flag unusual or improbable entries immediately upon data ingestion, minimizing propagation of errors downstream.
4. Data Enrichment
AI enriches datasets by sourcing additional contextual information:
- Contextual Understanding: NLP (Natural Language Processing) technologies can extract relevant details from unstructured sources, enriching structured data sets.
- Predictive Enrichment: Machine learning models predict and automatically populate missing fields based on historical trends and contextual information.
Key AI Technologies in Data Quality Management
Several AI technologies have emerged as vital tools for enhancing data quality and management:
1. Machine Learning (ML)
ML algorithms learn from historical data, automatically refining processes as new data is ingested. They enhance accuracy, consistency, and predictive capabilities within data management.
2. Natural Language Processing (NLP)
NLP techniques enable understanding, interpreting, and organizing unstructured textual data, greatly expanding the scope and usability of the data sources available to an organization.
3. Computer Vision
For visual data, computer vision automates tagging, classification, and validation tasks, significantly accelerating the management of visual assets.
4. Robotic Process Automation (RPA)
Integrating RPA with AI-driven decision-making enhances automation of repetitive and rule-based tasks, greatly reducing manual effort and minimizing errors in data entry.
Benefits of Leveraging AI for Data Quality Management
AI-driven data quality management offers substantial advantages, including:
- Improved Accuracy and Reliability: Enhanced data integrity significantly elevates trustworthiness and decision confidence.
- Enhanced Productivity: Automation of routine tasks frees teams to focus on strategic initiatives.
- Faster Data Processing: Accelerated data cleansing, integration, and validation allow organizations to respond to market trends more rapidly.
- Better Decision-making: Access to accurate, high-quality data results in informed, strategic decisions.
- Cost Savings: Reduction in manual labor, minimized data storage redundancies, and fewer costly mistakes yield significant cost benefits.
Real-World Applications
AI-driven data quality management is transforming industries. Consider the following examples:
Healthcare
AI algorithms cleanse medical records data, removing duplication and resolving inconsistencies, directly improving patient care quality and outcomes.
Financial Services
Machine learning models in banking detect fraudulent transactions by continuously analyzing transaction patterns and anomalies, ensuring transactional data integrity.
Retail and E-commerce
AI enriches consumer data, enabling accurate personalized marketing strategies. It detects data anomalies, ensuring accurate inventory and supply chain management.
Steps for Effective AI Implementation in Data Management
To leverage AI effectively, follow these critical implementation steps:
1. Assess Current Data Environment
- Audit your current data infrastructure.
- Identify data quality issues and their business impacts.
- Understand your requirements clearly.
2. Define Clear Objectives
- Set precise, measurable goals for your data quality improvement initiatives.
- Align your AI implementation strategy to these specific goals.
3. Select Appropriate Technologies and Tools
- Evaluate AI solutions and platforms suitable for your specific needs.
- Ensure compatibility with your existing infrastructure.
4. Ensure High-Quality Training Data
- AI performance directly depends on training data quality.
- Curate and manage data carefully to avoid bias and inaccuracies.
5. Integrate and Test AI Solutions
- Conduct thorough testing of AI models before full-scale deployment.
- Gradually integrate AI solutions into your current workflow to identify potential issues early.
6. Monitor and Refine Continuously
- Implement real-time monitoring systems to ensure ongoing accuracy and performance.
- Continuously fine-tune AI models based on changing requirements and data patterns.
Overcoming Challenges in AI Adoption
Despite numerous benefits, AI adoption can pose challenges such as:
- Bias in AI algorithms: Ensure AI training sets represent diverse data to avoid biased outputs.
- Integration complexity: Invest in expertise and robust infrastructure planning to streamline AI adoption.
- Data privacy concerns: Maintain strict compliance with privacy regulations and robust data governance practices.
Addressing these challenges proactively maximizes your AI implementation’s effectiveness and ROI.
Conclusion: Empowering Your Data Strategy
As the digital transformation accelerates, AI-powered data quality management becomes not just beneficial, but essential. Businesses committed to leveraging AI can expect improved accuracy, efficiency, and strategic outcomes. Embracing AI today positions your organization to unlock the full potential of its data resources, significantly enhancing overall business performance and competitive advantage.
Ready to elevate your data quality and management practices? Explore our expert AI-driven data management solutions and unlock the potential of your organization’s data.