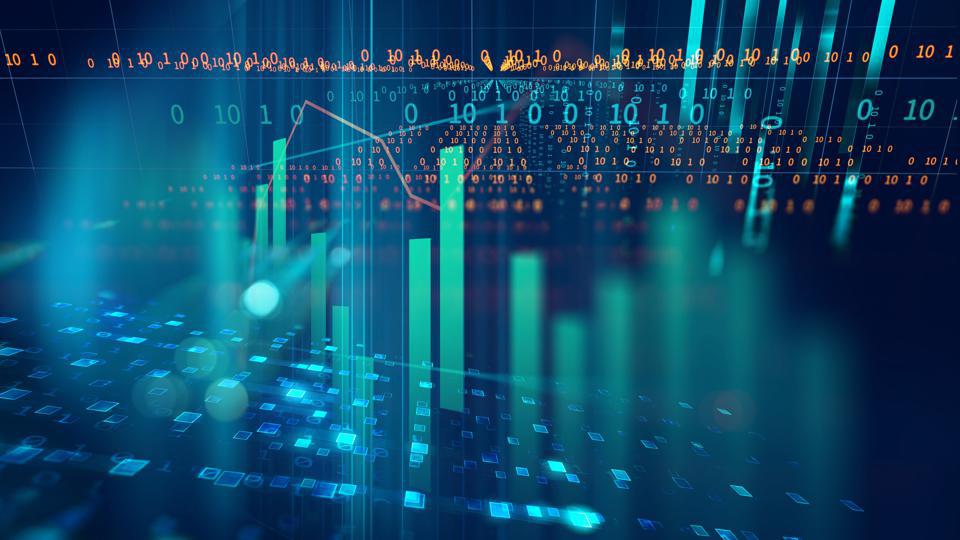
Navigating the Future: The Latest Trends in Data Analytics
Navigating the Future: The Latest Trends in Data Analytics
Consider this: by 2025, it’s estimated that worldwide data will reach a mind-boggling 175 zettabytes. That’s equivalent to stacking DVDs from Earth to the Moon and back—over 23 times! Amidst this data deluge, the importance of effective data analytics cannot be overstated.
From its early days of simple descriptive analytics to today’s sophisticated predictive and prescriptive analytics, the field has evolved at a breakneck pace. Emerging trends in data analytics are now setting the stage for even more revolutionary changes. These include the rise of artificial intelligence (AI) in data processing, the shift towards cloud-based analytics platforms, and the burgeoning importance of data ethics and governance.
1: Increased Adoption of Artificial Intelligence and Machine Learning
The integration of Artificial Intelligence (AI) and Machine Learning (ML) into data analytics is one of the most transformative trends in the industry. AI and ML technologies enhance traditional analytics by automating complex processes and enabling faster and more accurate analysis of big data sets. This shift is empowering companies to predict future trends, optimize operations, and personalize customer experiences like never before.
AI and ML in Action
Several tools and platforms are at the forefront of integrating AI and ML into data analytics. For instance, Google’s BigQuery ML empowers users to build and deploy machine learning models directly in Google BigQuery, using SQL queries. This integration simplifies the process of applying machine learning to large datasets without requiring specialized expertise in data science.
IBM Watson is another powerful tool that leverages AI to provide insights and predictive analytics across various sectors, including health care, finance, and retail. Watson can analyze unstructured data from numerous sources such as emails, social media, and more to deliver nuanced insights that drive strategic business decisions.
Key Benefits of AI and ML in Data Analytics
The adoption of AI and ML in data analytics brings several key benefits:
Improved Accuracy: AI algorithms continuously learn from new data inputs, which enhances their accuracy over time. This learning ability allows for more precise forecasts and smarter decision-making.
Increased Efficiency: Machine learning models can analyze vast amounts of data at speeds no human could achieve. This speed enables businesses to quickly identify trends, anomalies, and opportunities, leading to faster decision-making processes.
Automation of Routine Tasks: AI can automate repetitive and time-consuming tasks such as data cleaning and processing. This automation frees up human analysts to focus on more strategic tasks that require human insight.
Enhanced Personalization: AI-driven analytics can sift through enormous datasets to identify patterns in customer behavior, enabling companies to tailor products, services, and experiences to individual preferences, thereby enhancing customer satisfaction and loyalty.
Risk Management: Advanced predictive capabilities of AI models help organizations anticipate potential problems and mitigate risks. In financial sectors, for instance, AI can predict market changes and help companies adapt their strategies accordingly.
As AI and ML continue to advance, their integration into data analytics will only deepen, bringing more sophisticated tools and technologies that enhance the ability of organizations to harness the power of their data. This not only democratizes data analytics but also opens up new horizons for innovation across all sectors.
2: Shift Towards Cloud-Based Analytics Solutions
The landscape of data analytics has undergone a significant transformation with the shift from traditional on-premise data centers to cloud-based analytics solutions. This transition has been driven by the need for greater scalability, flexibility, and cost-effectiveness in managing the ever-increasing volumes of data.
The Move to the Cloud
Traditionally, businesses stored and analyzed data on-premise, requiring substantial investment in physical hardware, IT infrastructure, and maintenance. However, as data volumes have exploded and the pace of business has accelerated, on-premise solutions have struggled to keep up. Cloud-based analytics platforms offer a compelling alternative, allowing data to be stored, processed, and analyzed remotely on servers maintained by cloud service providers.
Benefits of Cloud-Based Analytics
Scalability: One of the most significant advantages of cloud-based analytics is scalability. Companies can easily scale their data storage and computing power up or down based on their needs without the upfront cost of purchasing and maintaining physical servers. This flexibility is particularly beneficial for businesses with fluctuating data processing needs.
Cost-Effectiveness: Cloud analytics can be more cost-effective than on-premise solutions. With the cloud, companies pay only for the storage and services they use, eliminating the need for large capital expenditures on hardware. Additionally, the maintenance and updates of the infrastructure are handled by the service provider, reducing the burden on internal IT teams.
Enhanced Collaboration: Cloud-based solutions facilitate better collaboration across teams and geographical locations. Data and insights can be accessed and shared in real time, enabling teams to work together more efficiently and make decisions quickly, regardless of their physical location.
Addressing Data Security Concerns
Despite the benefits, the shift to cloud-based solutions has raised concerns about data security and privacy. Storing sensitive information on external servers and transmitting data over the internet inherently increases vulnerability to cyber attacks. However, cloud service providers are continually enhancing their security measures to mitigate these risks.
Advanced Security Measures: Many cloud providers implement robust security protocols such as encryption, multi-factor authentication, and secure access controls to protect data. Encryption ensures that data is unreadable to unauthorized users, while multi-factor authentication adds an extra layer of security before access is granted.
Regulatory Compliance: Reputable cloud providers are often compliant with major regulatory standards such as GDPR, HIPAA, and PCI-DSS, which dictate how data should be securely handled and protected.
Continuous Monitoring and Updates: Cloud providers also offer the advantage of continuous monitoring and regular updates to their security infrastructure to protect against the latest threats and vulnerabilities.
While data security remains a crucial consideration, the benefits of cloud-based analytics—scalability, cost-effectiveness, and enhanced collaboration—make it an increasingly attractive option for businesses looking to leverage advanced data analytics capabilities. As technology and security practices evolve, the adoption of cloud solutions is likely to accelerate, further enhancing the way organizations manage and analyze data.
3: The Rise of Edge Analytics
As the Internet of Things (IoT) continues to expand, so does the need for more efficient ways to process the vast amounts of data generated by connected devices. This need has given rise to edge analytics, a key development in data processing that is set to transform how data is analyzed across various industries.
Understanding Edge Analytics
Edge analytics refers to the process of analyzing data at the point of generation, rather than transmitting it to a centralized data center or cloud. This approach utilizes powerful analytics algorithms directly on IoT devices or local edge servers, allowing for real-time data processing and decision-making. Edge analytics is crucial in the IoT ecosystem where the immediacy of data processing can be critical—for example, in autonomous vehicles or emergency response systems.
Benefits of Edge Analytics
Reduced Latency: By processing data locally, edge analytics significantly cuts down the time taken to analyze and act upon data, essentially enabling real-time decision-making. This is vital in scenarios where even milliseconds matter, such as in manufacturing processes or during emergency services.
Bandwidth Conservation: Transmitting large volumes of data can be costly and bandwidth-intensive. Edge analytics minimizes the need to send data back to a central server or cloud, thereby reducing bandwidth requirements and associated costs.
Enhanced Privacy and Security: Processing data locally means sensitive information does not have to travel over the network, reducing the risk of interception. This is particularly important in industries where data security is paramount, such as healthcare or finance.
Use Cases in Industries
Manufacturing: In the manufacturing sector, edge analytics is used to monitor equipment performance in real time. Sensors can detect anomalies in equipment operation and immediately trigger corrective measures without the need for cloud processing. This not only prevents equipment failure but also optimizes the manufacturing process for efficiency and reduced downtime.
Retail: Retailers are using edge analytics to enhance customer experiences. For example, smart shelves equipped with weight sensors and cameras can analyze consumer behavior and inventory levels directly at the point of sale. This data can be used to trigger restocking, adjust pricing dynamically, or even tailor promotions to shopper preferences in real time.
Smart Cities: Edge analytics plays a crucial role in smart city initiatives. Traffic management systems can process data from cameras and sensors to adjust traffic signals based on real-time road conditions, easing congestion and reducing accident rates. Similarly, public safety applications can use local data processing to detect and respond to emergencies faster and more effectively.
The rise of edge analytics marks a significant shift in data processing, particularly in the IoT-driven landscape. By enabling immediate insights and actions directly at the data source, edge analytics not only enhances efficiency and speed but also opens new avenues for innovation across various sectors, paving the way for smarter, more responsive technologies.
4: Growing Importance of Data Governance and Ethics
In an era where data breaches regularly make headlines and personal data has become a new currency, the importance of data governance and ethics in analytics cannot be overstated. As organizations increasingly rely on data-driven decision-making, establishing robust data governance frameworks and adhering to ethical guidelines have become imperative.
The Need for Robust Data Governance Frameworks
Data governance involves the overall management of the availability, usability, integrity, and security of the data employed in an enterprise. A robust data governance framework ensures that data across the organization is accurate, consistent, and used appropriately. It sets the policies and procedures that govern data collection, storage, and access, ensuring compliance with relevant laws and ethical standards.
Ethical Considerations in Data Usage
The ethical use of data refers to using data in ways that are morally sound and respect individual rights. Key ethical considerations include:
Consent and Privacy: Ensuring that data is collected and used with the informed consent of individuals. This involves being transparent about how data is used and giving individuals control over their own information.
Bias and Fairness: Addressing biases in data collection and analysis to prevent discriminatory outcomes. This is particularly crucial in algorithms that make decisions affecting individuals, such as those used in hiring, lending, and law enforcement.
Accountability: Establishing clear accountability for data-driven decisions. Organizations must be able to explain and justify how data analytics conclusions are reached, particularly when these decisions have significant impacts on individuals.
Legislation Impacting Analytics Practices
Several pieces of legislation have been enacted globally to regulate how data is collected, stored, and processed. One of the most comprehensive is the General Data Protection Regulation (GDPR) in the European Union.
Impact of GDPR:
Enhanced Data Protection: GDPR requires businesses to implement suitable safeguards that protect personal data. This includes mandates on data encryption, anonymization, and the ethical handling of data.
Increased Accountability: Organizations must not only comply with the GDPR but also demonstrate compliance through appropriate documentation and regular audits.
Rights of Individuals: GDPR strengthens the rights of individuals in relation to their data. Individuals have the right to access their data, correct inaccuracies, and even request deletion in certain circumstances.
The impact of GDPR has been profound, influencing not only European businesses but any global entity that handles the data of EU citizens. This legislation has prompted organizations worldwide to reassess their data handling and analytics practices, leading to more rigorous data governance structures.
As the digital landscape evolves, the emphasis on data governance and ethics becomes more pronounced. Organizations must not only focus on leveraging data for business gains but also ensure they are doing so in a manner that is ethical and compliant with increasing regulatory requirements. By prioritizing ethical considerations and robust governance, companies can mitigate risks and enhance their reputations, ultimately building trust with consumers and stakeholders.
5: Enhanced Visualization Tools for Data Interpretation
In the realm of data analytics, the ability to clearly and effectively visualize complex datasets is crucial for deriving actionable insights. Advancements in data visualization tools and techniques have significantly improved how businesses interpret vast amounts of data, making it easier to identify trends, patterns, and outliers. These tools transform raw data into visual formats that are intuitive and accessible, enabling stakeholders at all levels to make informed decisions.
Advancements in Data Visualization
Recent years have seen major innovations in data visualization tools, incorporating everything from real-time data streams to interactive and 3D visualizations. Enhanced capabilities such as automated insights, integration with AI for predictive analytics, and customizable dashboards have set new standards in how data is presented and interpreted.
Benefits of Modern Visualization Tools
Intuitive Data Interpretation: Modern visualization tools convert complex data sets into more understandable and engaging graphical formats, helping users to comprehend the information quickly and fully. This intuitive presentation allows non-experts to recognize patterns and correlations without needing to understand the underlying statistical techniques.
Real-Time Data Interaction: Many advanced tools now offer capabilities for interacting with data in real-time. Users can adjust parameters, drill down into specific metrics, or explore different scenarios directly within the visualization platform. This interactivity enhances the analytical process, making it dynamic and user-driven.
Enhanced Decision Making: By providing clear and immediate visualizations, these tools help businesses to quickly identify areas that require attention, track changes over time, and make informed decisions based on up-to-date data.
Popular Visualization Tools and Their Features
Tableau: One of the leaders in the field, Tableau is renowned for its ability to create a wide range of interactive and sophisticated visualizations. Key features include drag-and-drop capabilities, integration with a variety of data sources, and powerful options for creating dashboards and reports.
Power BI by Microsoft: Known for its deep integration with other Microsoft products, Power BI is a robust business analytics service that offers comprehensive modeling, real-time analytics, and custom development options. Its ability to integrate with Azure makes it particularly powerful for handling large datasets and incorporating machine learning models.
Qlik Sense: Qlik offers an intuitive platform for data exploration and discovery, with a strong emphasis on self-service capabilities. It provides associative analytics that highlight relationships between data points, smart search features, and responsive design that adapts to any device.
D3.js: For organizations looking for a more customizable approach, D3.js is a JavaScript library that allows developers to bind arbitrary data to a Document Object Model (DOM), and then apply data-driven transformations to the document. D3’s flexibility enables the creation of complex graphs and interactive visualizations that are tailored precisely to an organization’s needs.
As data continues to grow in volume and complexity, the role of advanced visualization tools becomes increasingly important in business analytics. These tools not only simplify data analysis but also enhance the overall decision-making process, enabling businesses to respond more quickly and effectively to changing market dynamics and internal performance metrics.
6: Predictive Analytics in Decision Making
Predictive analytics harnesses statistical algorithms and machine learning techniques to forecast future outcomes based on historical and current data. It plays a crucial role in decision-making across various sectors by providing insights not just into what has happened, but what is likely to happen in the future. This foresight enables organizations to proactively plan and strategize rather than reactively respond, transforming data into a valuable strategic asset.
The Role of Predictive Analytics in Forecasting Trends
Predictive analytics helps organizations identify trends, predict events, and generate actionable insights, thereby enhancing operational efficiency and minimizing risks. By analyzing patterns in historical data, predictive models can anticipate trends and behaviors days, months, or even years into the future. This predictive power is particularly valuable in dynamic environments where early anticipation of changes can provide a competitive edge.
Industries Leveraging Predictive Analytics
Finance: In the financial industry, predictive analytics is used extensively to assess credit risks, manage financial risks, and automate trading decisions. For example, banks use predictive models to determine the likelihood of a borrower defaulting on a loan, enabling them to make informed lending decisions. Similarly, investment firms use predictive algorithms to analyze market conditions and optimize investment strategies.
Healthcare: Predictive analytics is revolutionizing healthcare by improving patient care and managing costs. Hospitals utilize predictive models to forecast patient admissions, which helps in resource allocation and operational planning. Additionally, predictive tools can identify patients at high risk of chronic diseases, allowing for earlier interventions.
Marketing: In marketing, predictive analytics is used to understand customer behavior and enhance customer engagement. By analyzing past purchasing patterns and customer interactions, businesses can predict future buying behaviors and tailor their marketing strategies to increase customer retention and optimize marketing spend.
Challenges and Solutions in Adopting Predictive Analytics
While predictive analytics offers significant benefits, its adoption comes with challenges:
Data Quality and Availability: The accuracy of predictive models heavily depends on the quality and completeness of the input data. Poor data quality can lead to inaccurate predictions.
Solution: Implementing robust data governance practices to ensure data accuracy, completeness, and consistency is crucial. Organizations need to invest in technology and processes that clean and validate data regularly.
Skill Gap: Predictive analytics requires a certain level of expertise in data science and related fields, which can be a barrier for many organizations.
Solution: Investing in training for current employees, hiring skilled data professionals, and using automated predictive analytics tools that simplify the model-building process can help bridge this gap.
Integration with Existing Systems: Integrating predictive analytics tools with existing IT infrastructure can be complex and time-consuming.
Solution: Choosing predictive analytics solutions that can be seamlessly integrated with existing systems or opting for cloud-based solutions that offer flexibility and ease of integration.
Ethical and Privacy Concerns: Using predictive analytics involves handling a lot of personal data, which raises privacy and ethical concerns.
Solution: Organizations must adhere to regulatory requirements like GDPR and develop clear policies regarding data use that respect customer privacy and ensure transparency.
Predictive analytics is a powerful tool that can significantly enhance decision-making and operational efficiency across various industries. Despite the challenges, with the right strategies and solutions, organizations can effectively implement predictive analytics to harness its full potential.
7: Integration of Big Data with Data Analytics
In the modern era, the integration of big data with traditional analytics practices is reshaping how businesses operate, innovate, and compete. Big data refers to the enormous volumes of data generated every second from various sources like social media, sensors, digital images, and more. This integration involves using advanced analytics techniques to extract valuable insights from this vast amount of data, which can then be used to inform decision-making and strategic planning.
How Big Data is Being Integrated into Traditional Analytics\
The integration process often involves sophisticated data processing technologies and methodologies that can handle the scale, variety, and complexity of big data. This integration has prompted the evolution of traditional analytics into more dynamic, predictive analytics and machine learning, which can process and analyze data in real-time. For example, big data technologies like Hadoop and Spark have been crucial for storing and processing large datasets that traditional data management tools could not handle efficiently.
Challenges and Technological Advancements
Challenges:
Volume and Variety: The sheer volume and diversity of big data can overwhelm traditional data processing tools and infrastructure.
Speed: The velocity at which data flows into organizations can be challenging to manage effectively with conventional analytics tools.
Data Quality and Security: Managing the quality of massive datasets and ensuring their security are significant concerns.
Technological Advancements:
Cloud Computing: Cloud services offer scalable resources to store and analyze large datasets, alleviating the burden on local infrastructure.
Machine Learning and AI: These technologies are crucial in automating the analysis of big data, making it possible to quickly derive insights from large datasets.
Data Lakes: Unlike traditional data warehouses, data lakes allow the storage of unstructured data, supporting the analytics needs of big data environments.
Case Studies Where Big Data Has Transformed Business Strategies
Amazon: Utilizing big data, Amazon has revolutionized its supply chain and customer service. The company uses predictive analytics to anticipate customer demand, optimize delivery routes, and manage inventory effectively. This capability allows Amazon to enhance its operational efficiency and customer satisfaction significantly.
Netflix: Netflix uses big data to power its recommendation engine, which analyzes billions of records to suggest movies and TV shows to users based on their viewing history and preferences. This personalization has been critical in improving user engagement and retention.
Healthcare Sector: Big data has transformed healthcare strategies through predictive analytics and patient data analysis. For example, hospitals use big data to predict patient admission rates, which helps in resource allocation and operational planning. In research, big data enables the analysis of vast amounts of genetic data, contributing to breakthroughs in personalized medicine.
The integration of big data with traditional analytics practices is not just enhancing the capacity to handle data but also revolutionizing decision-making processes across various industries. Despite the challenges, continued technological advancements are making it increasingly feasible to derive meaningful and actionable insights from big data, thereby transforming business strategies and outcomes.
Conclusion
The landscape of data analytics is continually evolving, and the advancements discussed in this blog—from the integration of AI and machine learning to the transformative power of big data—are just the tip of the iceberg. As these technologies become more sophisticated and accessible, they hold the promise to revolutionize industries, enhance operational efficiencies, and provide deeper insights into customer behaviors and market dynamics. For businesses, staying abreast of these trends isn’t just about keeping up with the competition; it’s about setting the pace, predicting future needs, and delivering solutions that resonate both today and tomorrow.
Are you ready to harness the full potential of these cutting-edge data analytics trends in your organization?
At Datahub Analytics, we specialize in helping businesses like yours navigate the complex landscape of modern data analytics. Whether you’re looking to optimize your operations with AI-enhanced tools, shift to cloud-based solutions, or unlock new insights with big data, our expert team is here to guide you every step of the way.