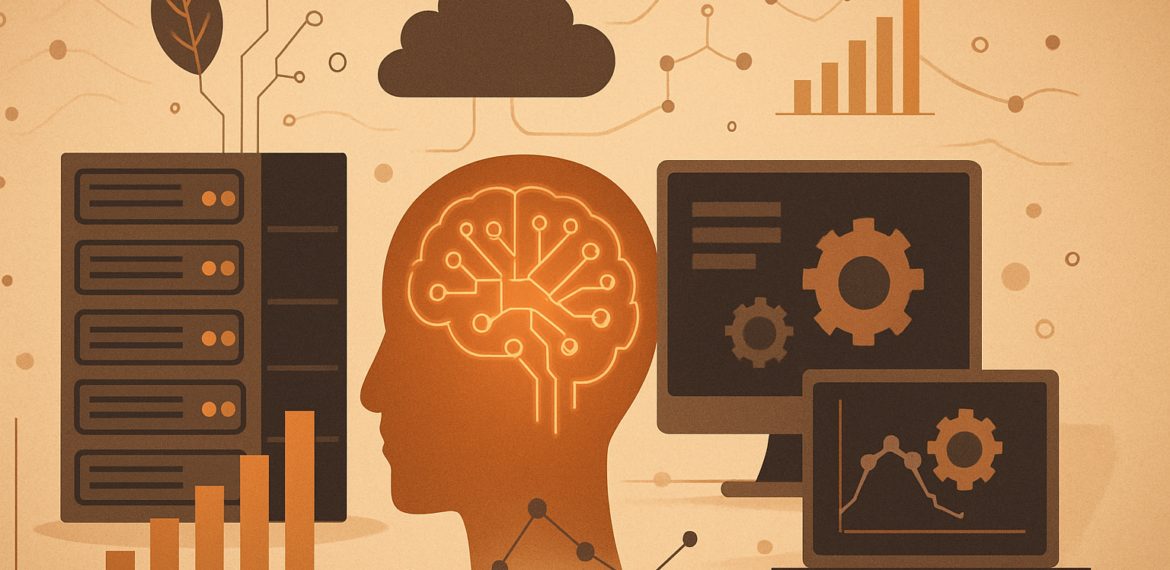
Scaling Your AI Initiatives with a Strong Data Foundation
Scaling Your AI Initiatives with a Strong Data Foundation
In today’s rapidly evolving digital landscape, artificial intelligence (AI) is no longer a futuristic concept. It’s here, and it’s revolutionizing the way businesses operate—from automating mundane processes to enabling predictive analytics and hyper-personalized customer experiences. However, as companies in Jordan, the wider MENA region, and globally embrace AI, one truth remains constant: AI is only as powerful as the data that fuels it.
To truly scale AI initiatives and generate meaningful ROI, organizations must first establish a robust data foundation. This blog explores why a strong data backbone is critical, what it entails, and how your organization can build it strategically.
The AI Hype vs. AI Reality
AI promises transformative outcomes—optimized operations, smarter decision-making, and new revenue streams. However, many enterprises encounter a stark reality:
- Models that don’t perform as expected
- Disconnected data systems
- Inconsistent or poor-quality data
- A lack of skilled data talent
- Difficulty operationalizing AI at scale
In most of these cases, the culprit isn’t the AI algorithm itself—it’s the underlying data architecture.
Why AI Needs a Strong Data Foundation
Here’s how a modern data foundation supports and scales AI:
1. Data Availability: Centralizing Your Data Assets
AI requires access to a variety of structured and unstructured data—historical records, customer interactions, sensor data, third-party feeds, and more.
- Without a unified data lake or modern data warehouse, AI projects are limited by data silos.
- Centralizing data in platforms like Snowflake, Google BigQuery, or Azure Synapse improves accessibility and collaboration.
2. Data Quality and Consistency
AI models are only as accurate as the data they’re trained on.
- Inconsistent, incomplete, or duplicate data can skew model outcomes and reduce trust.
- Implementing data cleansing, deduplication, and enrichment pipelines ensures high-quality, reliable inputs for AI/ML models.
3. Metadata, Lineage, and Governance
Understanding where data comes from and how it has been transformed builds transparency and trust.
- Data lineage helps debug models and maintain compliance.
- Governance ensures only authorized personnel access sensitive information, supporting ethical AI.
4. Real-Time and Batch Processing
Some AI use cases—fraud detection, dynamic pricing, customer support—demand real-time data ingestion and analysis.
- A robust foundation includes streaming capabilities (e.g., Apache Kafka, Spark Streaming).
- Others like churn prediction or demand forecasting may rely on batch processing—which must be optimized for scale.
5. Scalable Infrastructure
Training and deploying AI models requires significant computing resources.
- Cloud-native and containerized environments (Kubernetes, Docker) allow for elastic scaling.
- High-performance infrastructure ensures that AI workloads don’t slow down or fail.
Key Components of a Strong Data Foundation
To scale your AI ambitions, here’s what your data foundation should include:
A. Modern Data Architecture
- Data Lakes & Lakehouses: Combine raw and structured data for flexibility.
- Data Warehouses: Optimized for analytics and reporting.
- Data Mesh: Promotes domain ownership and decentralized data governance.
- ETL/ELT Pipelines: Tools like Airbyte, Fivetran, or Apache NiFi to move and transform data.
B. Master Data Management (MDM)
- Ensures consistency in core business entities (e.g., customers, products).
- Crucial for use cases like personalized marketing or demand forecasting.
C. Metadata Management
- Track schemas, definitions, classifications, and quality metrics.
- Leverage tools like Alation, Collibra, or open-source alternatives.
D. Data Governance Framework
- Define roles, responsibilities, access controls, and compliance protocols (GDPR, HIPAA, local regulations in Jordan or KSA).
- Use DAMA (Data Management Body of Knowledge) as a guiding framework.
E. DataOps and MLOps Integration
- Automate data testing, model retraining, deployment, and monitoring.
- Use tools like MLflow, Kubeflow, or AWS SageMaker for streamlined AI pipelines.
Common Pitfalls in Scaling AI Without Data Readiness
- Initiating AI before establishing data maturity
- Building models without considering data drift or model governance
- Neglecting data privacy and security policies
- Underestimating the need for cross-functional collaboration (data engineers, scientists, domain experts)
A Strategic Roadmap to Build a Scalable Data Foundation for AI
Phase 1: Data Strategy & Assessment
- Conduct a data maturity assessment
- Identify gaps in availability, quality, architecture, and governance
- Align AI goals with business outcomes
Phase 2: Infrastructure Modernization
- Migrate from legacy on-prem systems to hybrid or cloud-native architecture
- Implement DevOps/Infrastructure as Code (IaC) for agility and consistency
Phase 3: Data Integration & Quality
- Break data silos using API integrations, data lake ingestion, or data virtualization
- Standardize and validate data using automation frameworks
Phase 4: Governance & Compliance
- Define data ownership and stewardship roles
- Apply data masking, encryption, and access policies
Phase 5: AI Readiness & Enablement
- Build shared datasets for training, testing, and validation
- Promote self-service AI/ML tools for business users
- Train staff in AI ethics, bias mitigation, and explainability
Use Case Spotlight: AI at Scale with a Strong Data Backbone
Client: A leading logistics provider in Saudi Arabia
Challenge: Inefficient demand forecasting and inventory management
Solution:
- Implemented a modern data lake on Azure Data Lake
- Built real-time ingestion pipelines using Apache Kafka
- Established master data governance for SKUs and regions
- Deployed ML models on Databricks for demand forecasting
Outcome: - 22% improvement in inventory turnover
- 3x faster insights for decision-making
- Fully scalable AI system for future expansion
The Role of Strategic Partners
Scaling AI initiatives isn’t just about tools and technology. It’s about strategy, orchestration, and execution. That’s where partners like Datahub Analytics come in:
We Offer:
- Data Strategy & Governance Consulting (based on DAMA principles)
- Modern Data Infrastructure Design (hybrid cloud, containerized setups)
- AI/ML Engineering & Model Deployment Services
- Data Quality Management & Metadata Frameworks
- Outsourced Talent for Scaling Analytics Teams
Final Thoughts: Think Data First, Then AI
AI has immense transformative potential—but without data, it’s like a car without fuel. Organizations across Jordan and the GCC that invest in a strong, scalable, and secure data foundation today will be the ones leading tomorrow’s AI revolution.
Before you train your next model, ask yourself:
- Is your data accessible, trusted, and governed?
- Can your infrastructure scale with your AI ambitions?
- Are your teams ready—technically and culturally—for AI at scale?
If the answer is no or not yet, start by reinforcing your data foundation.
Let Datahub Analytics help you:
- Design future-ready data infrastructure
- Accelerate AI deployment
- Govern your data for compliance and ethics
📩 Contact us today to schedule a data readiness assessment.