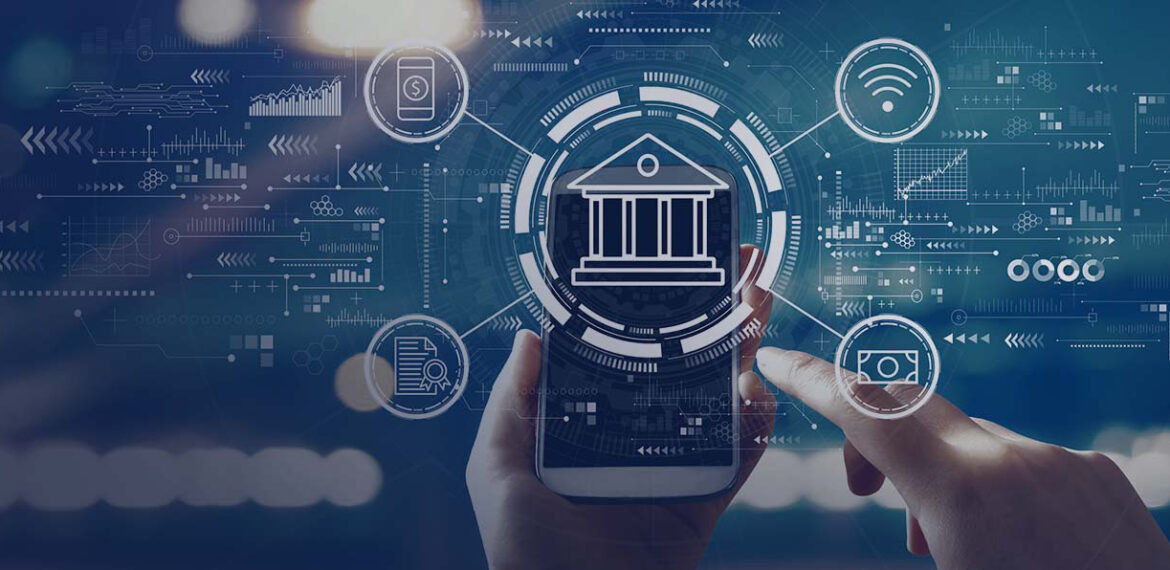
Use Cases Series – 1 – Unveiling the Power of Data Analytics in the Banking Industry
Use Cases Series – 1 – Unveiling the Power of Data Analytics in the Banking Industry
In the fast-paced world of finance, the banking industry is undergoing a transformative journey fueled by technological innovation. At the forefront of this evolution is the integration of data analytics, a powerful tool that not only navigates the complex landscape of financial data but also reshapes the very fabric of decision-making within the sector. In this comprehensive exploration, we unveil the profound impact of data analytics through key use cases, shedding light on how it is revolutionizing banking practices and ushering in a new era of efficiency, customer-centricity, and risk mitigation.
As the financial industry continues to grapple with unprecedented volumes of data, the importance of leveraging this information for strategic advantage becomes increasingly apparent. Data analytics emerges as the cornerstone, offering a lens through which banks can decipher patterns, uncover insights, and drive decisions that resonate with the dynamic needs of the market.
Informed decision-making has always been a critical aspect of the banking sector. However, as the industry becomes more complex and interconnected, the traditional methods of decision-making are proving insufficient. Data analytics becomes the beacon, illuminating the path toward more precise, informed, and agile decision-making.
The Data-Driven Paradigm Use Cases in Banking
1. Customer Segmentation for Targeted Marketing:
Customer segmentation stands as a cornerstone in the realm of data analytics for banking. By leveraging analytics to categorize customers based on behavior, preferences, and transaction history, banks gain invaluable insights into their diverse customer base. This segmentation lays the foundation for targeted marketing strategies, allowing financial institutions to tailor their offerings to specific customer segments. The result is not only heightened customer engagement but also increased satisfaction as banks deliver personalized services that resonate with individual needs.
2. Credit Risk Assessment and Management:
One of the critical challenges in banking is managing credit risks effectively. Data analytics, particularly through predictive modeling, transforms the traditional approach to credit risk assessment. By analyzing historical data and utilizing machine learning algorithms, banks can make more accurate and timely decisions, ensuring a proactive stance in mitigating credit risks. This use case not only enhances risk management strategies but also contributes to the overall financial health and stability of the institution.
3. Fraud Detection and Prevention:
The menace of fraud poses a constant threat to the banking sector, requiring robust defense mechanisms. Data analytics, armed with advanced algorithms and machine learning capabilities, is at the forefront of fraud detection and prevention. By analyzing transaction patterns, detecting anomalies, and identifying suspicious activities in real-time, banks can thwart fraudulent attempts before they inflict financial damage. This use case not only safeguards the interests of both the institution and its customers but also reinforces trust in the banking system.
4. Predictive Analytics for Financial Forecasting:
Accurate financial forecasting is imperative for strategic planning in the banking industry. Predictive analytics, driven by historical data and sophisticated algorithms, empowers banks to make precise predictions about market trends, customer behavior, and economic shifts. By anticipating future scenarios, financial institutions can make informed decisions, allocate resources effectively, and navigate the complexities of the financial landscape with confidence.
5. Operational Efficiency and Cost Optimization:
Operational efficiency lies at the core of a successful banking enterprise. Data analytics plays a pivotal role in streamlining operations, identifying inefficiencies, and optimizing costs. By analyzing internal processes, banks can automate routine tasks, allocate resources judiciously, and enhance overall operational agility. This use case not only improves efficiency but also contributes to long-term cost optimization, ensuring sustainable growth in a competitive environment.
6. Personalized Banking Services:
Data analytics enables banks to offer personalized services that go beyond traditional one-size-fits-all approaches. By understanding individual customer preferences, transaction histories, and financial goals, banks can tailor their services to meet specific needs. This use case not only enhances customer satisfaction but also fosters stronger customer loyalty, positioning the bank as a trusted financial partner in the eyes of its clients.
7. Cross-Selling and Upselling Opportunities:
Through data analytics, banks can identify cross-selling and upselling opportunities by analyzing customer behavior and transaction patterns. By understanding the products and services that align with individual customer needs, banks can create targeted campaigns and offers, maximizing revenue potential. This use case not only boosts the institution’s bottom line but also enhances the overall customer experience by providing relevant and valuable offerings.
8. Compliance and Regulatory Reporting:
Staying compliant with ever-evolving regulations is a constant challenge for banks. Data analytics simplifies the process of compliance and regulatory reporting by automating the collection and analysis of data required for reporting. This use case ensures that banks adhere to regulatory requirements, mitigating the risk of penalties and legal complications. Moreover, it enhances transparency and accountability in the banking operations.
9. Customer Churn Prediction:
Retaining existing customers is as crucial as acquiring new ones in the competitive banking landscape. Data analytics aids in predicting customer churn by analyzing patterns and identifying factors that contribute to customer attrition. Armed with these insights, banks can implement targeted retention strategies, such as personalized offers or improved customer service, to mitigate churn and strengthen customer relationships.
10. Sentiment Analysis for Customer Feedback:
Understanding customer sentiment is vital for enhancing customer experience and brand perception. Data analytics, particularly sentiment analysis, allows banks to analyze customer feedback from various channels, such as social media and surveys. By gauging the sentiment of customers, banks can identify areas for improvement, address concerns promptly, and proactively enhance their services based on real-time feedback.
Future Trends in Data Analytics for Banking
As the banking industry continues to traverse the digital landscape, the future of data analytics holds promises of unprecedented advancements and transformative trends.
Emerging Technologies Shaping the Future
The integration of emerging technologies is set to redefine the trajectory of data analytics in banking. Artificial Intelligence (AI) is expected to play a pivotal role, enhancing predictive analytics, automating processes, and providing deeper insights into customer behavior. Machine Learning (ML), coupled with AI, will further amplify the capabilities of data analytics, enabling banks to adapt and evolve in real-time based on evolving market conditions.
The Role of AI and Machine Learning
Artificial Intelligence and Machine Learning will not merely be tools but integral components driving innovation in data analytics for banking. AI-powered chatbots and virtual assistants will enhance customer interactions, providing real-time assistance and personalized experiences. Machine Learning algorithms will become more sophisticated, enabling banks to detect intricate patterns, optimize decision-making processes, and continually improve the accuracy of predictive analytics models.
Anticipated Advancements: A Glimpse into Tomorrow
Looking ahead, the banking industry can anticipate advancements that extend beyond traditional data analytics. The integration of blockchain technology is poised to revolutionize data security and transparency, ensuring the integrity of financial transactions. Furthermore, the adoption of sentiment analysis tools will enable banks to gauge customer emotions and preferences more accurately, fostering a deeper understanding of customer sentiments and enhancing customer-centric strategies.
Challenges and Considerations
While the integration of data analytics holds immense potential for revolutionizing the banking sector, it is not without its set of challenges and considerations. Navigating these hurdles is crucial for banks to harness the full benefits of data analytics and ensure a seamless transition to a data-driven future.
Common Challenges in Implementing Data Analytics
Implementing data analytics solutions within a banking framework presents inherent challenges. One primary obstacle is the vast and diverse datasets that banks handle, often stored in disparate systems. The integration of these datasets for comprehensive analysis poses a significant technical challenge. Additionally, ensuring data quality, accuracy, and consistency across various sources is a perpetual concern, as errors or inconsistencies can compromise the integrity of analytical outcomes.
Strategies for Overcoming Barriers
Overcoming these challenges requires a strategic approach. Banks must invest in robust data governance frameworks, ensuring data quality and integrity from collection to analysis. Establishing cross-functional teams that include data scientists, analysts, and domain experts can facilitate a holistic approach to data analytics implementation. Moreover, fostering a culture of collaboration and open communication within the organization is essential to break down silos and facilitate the seamless flow of information.
Data Security and Privacy Compliance
As banks navigate the expansive landscape of data analytics, ensuring the security and privacy of sensitive information is paramount. Data breaches can have severe consequences not only for customers but also for the reputation and trustworthiness of the financial institution. Adhering to strict data security protocols, implementing encryption measures, and regularly auditing systems for vulnerabilities are imperative steps. Moreover, compliance with stringent data protection regulations, such as GDPR and HIPAA, is essential to avoid legal repercussions and maintain customer trust.
Organizational Resistance to Change
Resistance to change within the organizational culture can pose a formidable challenge. Employees may be hesitant to adopt new technologies, processes, or ways of thinking, fearing job displacement or increased workloads. To address this, banks must invest in comprehensive training programs to upskill their workforce, fostering a culture that embraces innovation and continuous learning. Communication strategies that highlight the positive impacts of data analytics on both individual roles and the organization as a whole can also mitigate resistance.
Scalability and Integration Issues
As data analytics solutions evolve, scalability and seamless integration with existing systems become critical considerations. Banks must choose platforms and technologies that not only meet their current analytical needs but are also scalable to accommodate future growth. Integration with legacy systems can be complex, requiring careful planning and execution to avoid disruptions in day-to-day operations. Collaboration with experienced technology partners and vendors can aid in overcoming these integration challenges.
Ethical Considerations and Bias in Analytics
The ethical use of data analytics is a growing concern. Biases within datasets can lead to discriminatory outcomes, affecting decisions related to lending, promotions, or customer interactions. Banks must prioritize ethical considerations, regularly audit their analytics models for biases, and implement measures to address and rectify any identified issues. Transparent communication about how data is used and the ethical principles guiding analytics practices is crucial for maintaining customer trust.
Conclusion
The fusion of data analytics emerges as a strategic imperative, redefining industry norms. Explored through various use cases, from targeted marketing to predictive analytics, data analytics proves pivotal in enhancing efficiency, mitigating risks, and delivering personalized experiences. The future promises even greater innovation, with emerging technologies like artificial intelligence and blockchain poised to revolutionize the industry.
However, challenges in implementation, ethical considerations, and organizational resistance must be navigated. Strategic investments in technology, a culture of collaboration, and a commitment to transparency are essential. As the symbiotic relationship between data analytics and banking evolves, it invites financial institutions to embrace the digital era, charting a path toward a future where data becomes the guiding force, ensuring resilience, innovation, and a banking experience finely tuned to the diverse needs of customers. This integration is not just a trend; it is a strategic imperative shaping the future success of the banking industry.
Join us on this transformative journey where data becomes the compass guiding banking into a future marked by resilience, innovation, and unparalleled customer value.