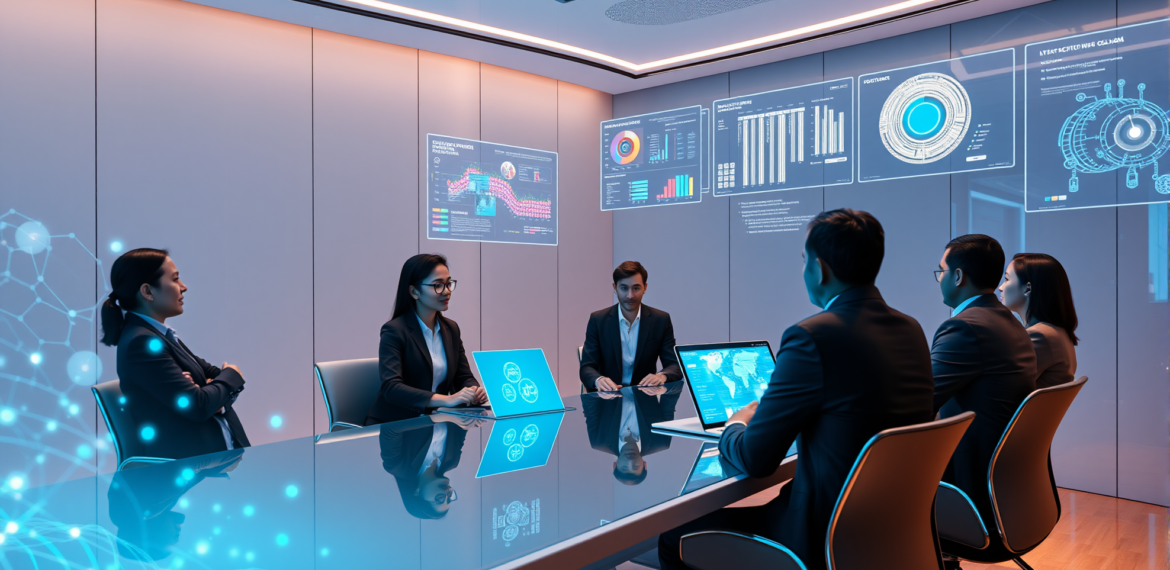
Bringing LLMs to the Boardroom: Creating Visual Dashboards Powered by AI
Bringing LLMs to the Boardroom: Creating Visual Dashboards Powered by AI
Executives and board members rely heavily on data analytics to craft strategies, mitigate risks, and forecast future trends. Yet, despite the abundance of data at their fingertips, many leaders struggle to translate complex datasets into actionable insights.
Traditional dashboards, while useful, often fall short. They present static snapshots, require manual data interpretation, and can overwhelm decision-makers with too much raw information and too little context. This creates a gap between data availability and data usability—especially in the boardroom, where every decision carries significant weight.
Enter Large Language Models (LLMs). These AI-powered systems are not just transforming natural language processing—they’re reshaping how data is analyzed and presented. When combined with visual dashboards, LLMs enable real-time insights, predictive analytics, and natural language querying, making it easier for executives to interact with data, uncover trends, and make strategic decisions faster and more accurately.
The Current State of Boardroom Analytics
In many boardrooms today, data plays a central role in shaping strategic decisions. However, the tools traditionally used to present this data—Business Intelligence (BI) dashboards—often fail to meet the evolving needs of modern executives. While these dashboards have served businesses well for years, they come with inherent limitations that can hinder decision-making at the highest levels.
1. Limitations of Conventional BI Dashboards
- Static Data Representation: Traditional dashboards often display static or historical data, limiting leaders’ ability to react to real-time changes. This time lag can result in missed opportunities or delayed responses to critical issues.
- Manual Updates and Maintenance: Many BI systems require periodic manual updates, leading to inconsistencies and outdated information. For board-level decision-making, where accuracy and timeliness are crucial, this is a significant drawback.
- Complex Querying Processes: Extracting specific insights from BI dashboards often demands technical expertise. Non-technical board members may rely on data analysts to run complex queries, creating bottlenecks and slowing down the decision-making process.
- Information Overload: Traditional dashboards can overwhelm users with vast amounts of raw data, making it difficult to identify key trends, risks, or opportunities without deep analytical skills.
2. The Growing Need for Real-Time, Intelligent Data Visualization
Today’s business environment demands agility. Executives need to react to market shifts, operational challenges, and customer trends in real time. Static dashboards can’t keep up with this pace. The need for real-time, dynamic dashboards that automatically update as new data flows in has become essential for data-driven boardroom discussions.
Moreover, beyond just seeing data, leaders need tools that help them understand the implications of that data. They require dashboards that not only visualize information but also provide context, highlight anomalies, and suggest possible courses of action.
3. Increasing Demand for AI-Driven Insights
The complexity of modern business data has outgrown the capabilities of traditional BI tools. As organizations collect more unstructured data from various sources—social media, IoT devices, customer interactions—the challenge isn’t gathering data; it’s making sense of it.
This is where AI-driven insights come into play. Executives are increasingly seeking tools that go beyond simple data visualization to provide predictive analytics, risk assessments, and trend forecasts. AI-enhanced dashboards can surface patterns, detect anomalies, and even recommend strategic actions, empowering board members to make faster, smarter decisions.
As boardrooms demand more intelligent, flexible, and user-friendly analytics tools, integrating Large Language Models (LLMs) with visual dashboards emerges as a transformative solution—ushering in a new era of decision-making that’s not only data-driven but also AI-augmented.
What Are LLMs and Why Do They Matter?
As the volume and complexity of business data continue to grow, traditional analytics tools often struggle to provide the depth of insight executives need. This is where Large Language Models (LLMs) step in, offering a transformative approach to data interaction and decision-making.
1. A Quick Overview of Large Language Models (LLMs)
Large Language Models (LLMs) are advanced AI systems trained on massive datasets to understand, process, and generate human language. Popular examples include GPT (Generative Pre-trained Transformer), Claude, and LLaMA (Large Language Model Meta AI). These models have the capability to interpret natural language, generate coherent responses, and even perform complex reasoning tasks.
While LLMs were initially developed for applications like chatbots, content creation, and virtual assistants, their potential extends far beyond. In the world of data analytics, LLMs can bridge the gap between complex datasets and human decision-makers by enabling more intuitive and conversational interactions with data.
2. LLMs’ Capabilities in Data Comprehension and Insight Generation
LLMs bring a new level of intelligence to data analytics, offering several key capabilities that traditional BI tools lack:
- ✅ Data Comprehension: LLMs can process structured and unstructured data, identify patterns, and contextualize information without needing extensive manual programming.
- ✅ Natural Language Querying: One of the most transformative features is the ability for users to interact with data using natural language. Instead of complex SQL queries or advanced coding, board members can simply ask, “What was our revenue growth last quarter?” and receive an immediate, data-backed response.
- ✅ Contextual Insights: LLMs don’t just present raw data—they interpret it. By understanding the context of a query, LLMs can highlight key trends, identify anomalies, and even explain why certain metrics changed, making the insights more actionable.
- ✅ Predictive and Prescriptive Analytics: Beyond analyzing historical data, LLMs can forecast future trends and suggest potential strategies, empowering leaders to make proactive decisions.
3. The Value of Conversational Analytics for Non-Technical Stakeholders
One of the most significant challenges in boardroom analytics is making complex data accessible to all stakeholders—especially those without technical expertise. This is where conversational analytics, powered by LLMs, becomes a game-changer.
With conversational analytics, board members no longer need to navigate complicated dashboards or rely on data analysts to interpret reports. Instead, they can engage with data directly through natural language conversations. Imagine a boardroom where executives can simply ask:
💬 “Which product line had the highest profit margin last year?”
💬 “How might a 5% price increase impact quarterly revenue?”
…and receive immediate, clear answers—complete with visualizations—without needing to understand the backend data structure.
This democratizes data access, empowers more informed discussions, and accelerates decision-making. LLMs make data analytics accessible, intuitive, and actionable for everyone in the boardroom, not just the data experts.
How LLMs Enhance Visual Dashboards
In traditional boardroom settings, data dashboards often require technical know-how to extract meaningful insights. Board members may find themselves dependent on data analysts to run specific queries or interpret complex reports, slowing down the decision-making process. Large Language Models (LLMs) change this dynamic by making dashboards smarter, more interactive, and accessible to all stakeholders—regardless of their technical background.
Here’s how LLMs transform visual dashboards into powerful decision-making tools:
✅ 1. Natural Language Queries: Simplifying Data Access
One of the most impactful features of LLMs is their ability to process natural language queries. Instead of navigating complex data filters or writing SQL queries, board members can simply type or ask questions in plain English (or any other supported language) and receive instant, data-driven answers.
Example:
- “What was the YoY revenue growth in the KSA region?”
- “Show me the top-performing products last quarter.”
LLMs interpret the context of these questions and pull the relevant data, often accompanied by visualizations like charts or graphs, making data exploration intuitive and efficient.
✅ 2. Automated Insights Generation: Going Beyond Raw Data
While traditional dashboards display data, LLM-powered dashboards explain it. LLMs can automatically scan through vast datasets, detect anomalies, surface trends, and highlight key takeaways—without requiring users to dig deep into the numbers.
Key Benefits:
- Trend Detection: Identify patterns in sales, customer behavior, or operational performance.
- Anomaly Alerts: Flag sudden changes or outliers, such as a sudden dip in revenue or unexpected cost spikes.
- Narrative Summaries: Convert complex data points into concise, human-readable insights (e.g., “Revenue increased by 12% in Q4, primarily driven by new market expansion”).
This automation eliminates the need for manual data analysis, saving time and reducing the risk of oversight.
✅ 3. Predictive Analytics: Anticipating Future Trends
Boardroom decisions often rely not just on historical data but also on forecasts. LLMs, when combined with machine learning algorithms, enable predictive analytics—helping leaders anticipate future outcomes based on past trends and current market dynamics.
Use Cases:
- Sales Forecasting: Predict next quarter’s revenue based on historical sales and market conditions.
- Customer Churn Analysis: Identify customers at risk of leaving and recommend retention strategies.
- Operational Optimization: Forecast inventory needs, supply chain disruptions, or resource allocation.
By integrating predictive models into dashboards, board members can make proactive decisions rather than reactive ones.
✅ 4. Scenario Planning: Exploring “What-If” Possibilities
LLMs empower decision-makers to run dynamic scenario planning directly within their dashboards. By altering variables and parameters, board members can see how different strategies might impact outcomes—without waiting for data analysts to build complex models.
Example “What-If” Scenarios:
- “What if we reduce operating expenses by 10%?”
- “How would increasing the marketing budget by 15% impact customer acquisition?”
- “What are the financial implications of expanding into a new market?”
LLMs process these hypothetical scenarios in real time, providing instant visual projections and helping leaders weigh different strategies before taking action.
Designing AI-Powered Dashboards for the Boardroom
An effective boardroom dashboard is more than just a collection of charts—it’s a strategic tool that empowers executives to make informed decisions quickly. By integrating Large Language Models (LLMs) into dashboard design, organizations can create intelligent, user-friendly interfaces that provide real-time insights and foster data-driven decision-making.
To maximize impact, AI-powered dashboards should balance sophisticated analytics with intuitive user experiences, ensuring board members can focus on insights rather than data manipulation.
✅ Key Features of AI-Powered Dashboards
- Natural Language Interface
One of the most transformative features of LLM-powered dashboards is the natural language interface. Executives can bypass complex filters and technical jargon by simply typing or speaking queries in plain language.
- Example Queries:
- “What were our top three revenue-generating regions last year?”
- “Show me the profit margin trends over the past five quarters.”
- Why It Matters: It democratizes data access, enabling non-technical stakeholders to interact with data effortlessly.
- Real-Time Data Updates
In high-stakes decision-making, outdated data can lead to missed opportunities or costly mistakes. AI-powered dashboards ensure real-time data synchronization by integrating directly with live data sources, enabling:
- Instant performance tracking
- Real-time alerts for anomalies or key events
- Continuous monitoring of KPIs during critical meetings
Executives gain a single source of truth, ensuring decisions are based on the most current data available.
- Customizable KPIs
Every boardroom has unique strategic priorities. AI-powered dashboards should allow users to customize KPIs to align with evolving business goals.
- Choose metrics relevant to the organization (e.g., ROI, customer acquisition cost, market share)
- Create role-specific views for different stakeholders (e.g., CFO sees financial KPIs, CMO focuses on customer engagement)
- Adapt KPIs in real time to match changing business objectives
This flexibility ensures that every board meeting focuses on what matters most.
- Interactive Charts and Predictive Visualizations
Static charts are a thing of the past. AI-powered dashboards enable interactive data exploration, allowing board members to:
- Drill down into data points (e.g., click on a revenue spike to see the contributing factors)
- Apply dynamic filters without leaving the dashboard
- Leverage predictive visualizations that use AI to forecast trends, identify risks, and simulate future scenarios
These features foster deeper engagement and more insightful discussions during board meetings.
⚡ Best Practices for Designing Effective Boardroom Dashboards
- Prioritize Clarity and Simplicity in Visualizations
Board members often juggle multiple priorities and need clear, concise insights. Avoid overwhelming them with complex charts or data-heavy screens.
- Use clean layouts with logical data groupings
- Highlight key figures and trends using color coding and callouts
- Present insights with executive summaries for quick comprehension
Pro Tip: Less is more—focus on high-level trends and allow drill-down options for deeper dives.
- Use AI-Driven Alerts for Anomalies
Board members shouldn’t have to sift through data to identify issues. Set up AI-driven alerts that automatically flag:
- Revenue drops
- Unexpected cost spikes
- Operational inefficiencies
- Market anomalies
These alerts can trigger push notifications or emails, ensuring leadership is always informed of critical changes—even outside board meetings.
- Integrate with Existing BI Tools (e.g., Power BI, Tableau)
Rather than starting from scratch, AI-powered dashboards should seamlessly integrate with widely-used BI platforms like Power BI, Tableau, or Looker.
- Leverage existing data infrastructure
- Enhance BI tools with LLM capabilities (e.g., natural language querying, predictive analytics)
- Facilitate cross-platform data sharing for a unified view of organizational performance
This approach reduces learning curves, encourages adoption, and extends the capabilities of existing systems.
Real-World Applications and Use Cases of AI-Powered Dashboards
Integrating Large Language Models (LLMs) into boardroom dashboards doesn’t just enhance data visualization—it transforms how executives approach decision-making across key business functions. By leveraging AI-driven insights, companies can make faster, smarter, and more strategic decisions that directly impact performance and growth.
Here are some real-world applications and use cases demonstrating how LLM-powered dashboards create tangible value:
💰 1. Financial Planning: AI-Driven Forecasting and Risk Assessment
Financial health is at the core of every strategic boardroom discussion. AI-powered dashboards take financial planning to the next level by providing real-time insights, predictive analytics, and risk assessments.
How LLMs Add Value:
- Revenue Forecasting: Predict future revenue streams based on historical data, seasonal trends, and market fluctuations.
- Expense Optimization: Identify cost centers and suggest areas for budget reallocation.
- Risk Analysis: Evaluate the potential impact of market volatility, currency fluctuations, and interest rate changes.
- Cash Flow Monitoring: Use real-time data to manage liquidity and ensure healthy cash flow.
Example Scenario:
A CFO uses an AI-powered dashboard to run “what-if” scenarios, evaluating the financial impact of increasing marketing spend by 15% or entering a new market. The dashboard provides real-time forecasts, helping the board make data-backed investment decisions.
⚙️ 2. Operational Efficiency: Identifying Bottlenecks and Optimizing Resources
Operational inefficiencies can lead to significant cost overruns and reduced productivity. AI-powered dashboards equipped with LLMs help leadership teams pinpoint inefficiencies and optimize workflows.
How LLMs Add Value:
- Supply Chain Optimization: Detect disruptions, predict stock shortages, and streamline logistics.
- Resource Allocation: Identify underutilized resources and suggest optimal allocation across departments.
- Process Bottleneck Detection: Analyze workflows to uncover delays or inefficiencies in manufacturing, logistics, or service delivery.
- Performance Benchmarking: Compare operational metrics across departments or geographies to identify best practices.
Example Scenario:
An operations manager identifies a recurring supply chain delay impacting product delivery timelines. Using the dashboard, the team pinpoints the bottleneck and optimizes logistics, reducing delivery times by 20%.
📊 3. Customer Insights: Analyzing Behavioral Patterns for Strategic Decisions
Understanding customer behavior is crucial for creating personalized experiences and driving growth. LLM-powered dashboards analyze massive amounts of customer data to uncover insights that shape product development, marketing strategies, and customer engagement.
How LLMs Add Value:
- Customer Segmentation: Automatically categorize customers based on demographics, behavior, and preferences.
- Churn Prediction: Identify at-risk customers using historical behavior and engagement data.
- Sentiment Analysis: Analyze customer feedback from social media, reviews, and surveys to gauge brand sentiment.
- Sales Funnel Optimization: Visualize customer journeys and identify drop-off points to improve conversion rates.
Example Scenario:
A CMO uses the dashboard to track customer engagement across various digital channels. The AI highlights that a new product line is resonating with a previously untapped demographic, leading to a targeted marketing campaign that increases sales by 25%.
💼 4. M&A Scenarios: Rapid Data Integration and Due Diligence Analysis
Mergers and acquisitions involve vast amounts of data that require rapid integration and careful analysis. LLM-powered dashboards streamline the due diligence process, enabling leadership to make informed decisions quickly.
How LLMs Add Value:
- Data Integration: Automatically consolidate financial, operational, and market data from both organizations.
- Risk Assessment: Identify potential legal, financial, and operational risks in the target company.
- Cultural Alignment Analysis: Use sentiment analysis and employee data to assess potential cultural integration challenges.
- Synergy Modeling: Run “what-if” scenarios to predict cost savings, revenue growth, and post-merger efficiencies.
Example Scenario:
During an acquisition, the board uses an AI-powered dashboard to evaluate the target company’s financials, customer data, and operational KPIs in real-time. The AI highlights cost synergies and potential integration challenges, helping the board finalize the deal with confidence.
Future of Boardroom Analytics: Where AI is Taking Executive Decision-Making
The integration of Large Language Models (LLMs) into boardroom analytics is only the beginning of a broader transformation. As AI technologies continue to evolve, boardrooms will shift from static, human-dependent decision-making frameworks to dynamic, intelligent platforms that offer proactive, data-driven strategies with minimal manual input.
Here’s a look at how boardroom analytics is evolving and what the future holds:
🚀 1. The Rise of Decision Intelligence Platforms
Decision Intelligence (DI) is emerging as the next frontier in data analytics. These platforms go beyond traditional dashboards by combining data science, artificial intelligence, and domain expertise to streamline and optimize decision-making processes.
How DI Platforms Will Shape Boardrooms:
- Contextualized Insights: DI platforms don’t just present data—they explain the why behind trends and suggest next steps.
- Predictive & Prescriptive Analytics: Boards will move from historical reporting to future-focused strategies, using AI to predict outcomes and recommend optimal actions.
- Decision Pathways: DI platforms can map out various strategic options, evaluate their potential impact, and recommend the most profitable or risk-averse path.
Example Scenario:
Rather than simply reporting quarterly financials, a DI platform could suggest adjustments to pricing strategies based on market fluctuations or customer behavior trends, enabling the board to make more nuanced, data-backed decisions.
🤖 2. Integration of LLMs with Emerging Technologies
The future of boardroom analytics lies in the convergence of multiple advanced technologies. LLMs will increasingly integrate with tools like Generative AI, IoT (Internet of Things), and real-time data streams to create more holistic and responsive analytics ecosystems.
Key Integration Opportunities:
- Generative AI: Beyond summarizing data, generative AI can simulate business scenarios, create financial forecasts, or even draft strategic reports for board review.
- IoT Data Streams: Real-time data from supply chains, manufacturing processes, and customer interactions can feed into dashboards, giving executives a live pulse on operations.
- Augmented & Virtual Reality (AR/VR): Board members could explore complex data models in immersive environments, enabling more intuitive analysis and scenario planning.
- Blockchain Integration: For industries requiring high levels of transparency and traceability, blockchain can be incorporated into analytics for secure data validation.
Example Scenario:
A logistics company’s board uses an AI-powered dashboard that integrates IoT data from delivery trucks. The LLM identifies inefficiencies in delivery routes and, using generative AI, proposes optimized routes, predicting a 15% cost reduction.
🤯 3. Autonomous Decision-Making: From Insight to Action
As AI systems become more sophisticated, the future points toward autonomous decision-making, where certain decisions can be executed without human intervention—especially for routine or low-risk processes.
How This Shift Will Unfold:
- Automated Risk Assessments: AI will detect financial or operational risks and initiate preventative actions without waiting for board approval.
- Self-Optimizing Systems: Supply chains, marketing campaigns, and even financial portfolios could self-adjust in real-time based on AI-driven insights.
- Human-AI Collaboration: While AI will handle data-heavy, repetitive tasks, human leaders will focus on high-level strategy, ethical considerations, and creative problem-solving.
The Long-Term Vision:
We may see the rise of “AI Board Advisors”—intelligent systems that sit alongside human board members, continuously feeding them real-time insights, flagging risks, and even proposing data-backed strategies during live discussions.
📊 What Does This Mean for the Modern Boardroom?
- Faster Decision Cycles: AI reduces the time between data analysis and action, enabling quicker strategic pivots.
- Data-Driven Agility: With real-time insights and predictive capabilities, boards can respond to market changes with unprecedented agility.
- Reduced Human Error: AI-driven analytics reduce the risk of oversight or bias, ensuring more objective decision-making.
- Ethical Considerations: As AI takes on a greater role in decision-making, organizations must establish guidelines for transparency, accountability, and fairness.
Conclusion
As boardrooms evolve to meet the demands of an increasingly data-driven world, relying solely on traditional dashboards and manual analysis is no longer enough. Executives need real-time insights, contextual data interpretation, and predictive foresight—all of which Large Language Models (LLMs) can provide. By integrating LLMs into visual dashboards, businesses can transform complex datasets into accessible, actionable insights, empowering leaders to make smarter, faster, and more strategic decisions.
AI-powered dashboards are not just about simplifying data visualization—they are about unlocking the full potential of enterprise data. From financial planning and operational efficiency to customer engagement and M&A strategies, LLM-driven dashboards offer a new level of intelligence, agility, and foresight in the boardroom.
As the future of boardroom analytics shifts toward Decision Intelligence platforms and autonomous decision-making, organizations that adopt AI-driven tools today will position themselves as industry leaders tomorrow.
✅ Ready to Bring AI to Your Boardroom?
At Datahub Analytics, we specialize in designing and implementing AI-powered dashboards that leverage the capabilities of Large Language Models to drive smarter decision-making at the executive level. Whether you need real-time financial forecasting, customer insights, or predictive analytics, our tailored solutions empower your leadership team to make data-backed decisions with confidence.
💡 Let’s transform your boardroom into a data-driven decision hub.
📞 Contact Datahub Analytics Today to schedule a consultation and discover how AI can unlock your organization’s full potential.