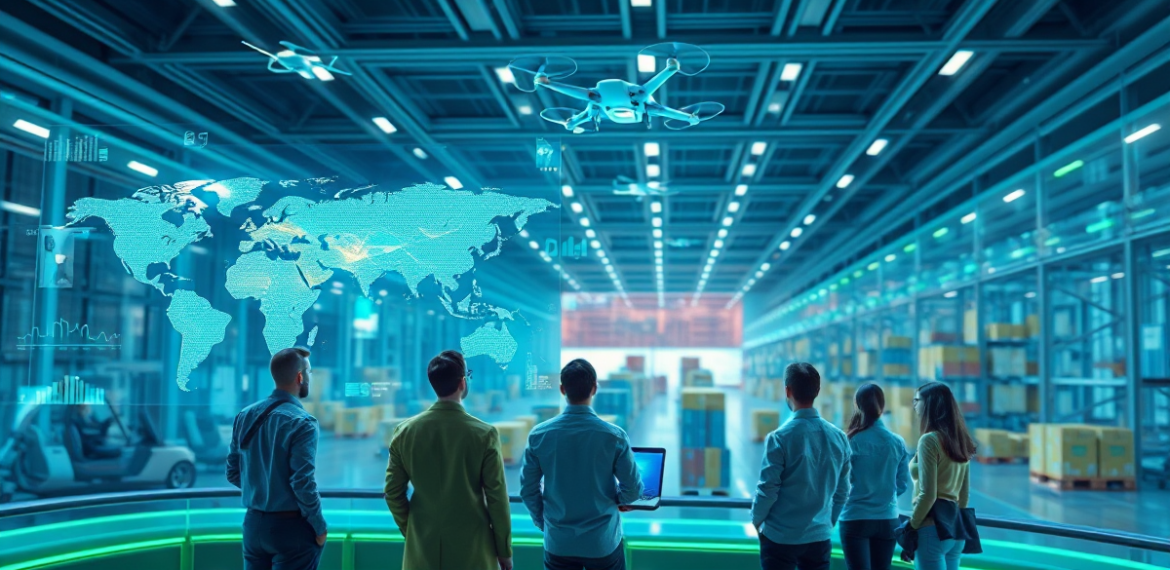
Predictive Analytics with AutoML: Reducing Supply Chain Disruptions by 35%
Predictive Analytics with AutoML: Reducing Supply Chain Disruptions by 35%
With suppliers, manufacturers, distributors, and customers spread across the globe, even minor disruptions can ripple through the entire network, leading to costly delays and operational inefficiencies. From natural disasters and geopolitical tensions to fluctuating consumer demands and raw material shortages, businesses face an increasing number of unpredictable challenges that can impact their bottom line.
The Challenge: Navigating Unpredictable Disruptions
Supply chain disruptions aren’t just inconvenient—they’re expensive. They can lead to delayed deliveries, lost sales, increased operational costs, and, ultimately, dissatisfied customers. Traditional forecasting methods and manual processes often fall short when it comes to predicting and mitigating these disruptions, leaving businesses vulnerable to unforeseen risks.
The Solution: Harnessing Predictive Analytics with AutoML
Predictive analytics, powered by AutoML (Automated Machine Learning), is revolutionizing supply chain management. By analyzing vast amounts of historical and real-time data, businesses can identify patterns, forecast potential disruptions, and make data-driven decisions that enhance efficiency and resilience. AutoML simplifies the process by automating complex machine learning tasks, enabling supply chain teams to gain actionable insights without the need for extensive data science expertise.
The Result? Companies implementing predictive analytics with AutoML have achieved up to a 35% reduction in supply chain disruptions—resulting in smoother operations, lower costs, and improved customer satisfaction.
Understanding Predictive Analytics and AutoML
In the rapidly evolving landscape of supply chain management, data-driven decision-making has become essential for staying competitive. Predictive analytics and AutoML (Automated Machine Learning) are two powerful tools that, when combined, empower businesses to anticipate disruptions, optimize operations, and reduce costs.
What is Predictive Analytics?
Predictive analytics uses historical data, statistical algorithms, and machine learning techniques to forecast future outcomes. In supply chain management, it plays a critical role in helping businesses:
- Anticipate demand fluctuations to improve inventory planning.
- Identify potential supply chain bottlenecks before they occur.
- Optimize logistics and distribution strategies for cost-efficiency.
- Forecast supplier performance and mitigate risks related to delays or shortages.
By shifting from reactive to proactive strategies, predictive analytics enables businesses to reduce operational risks and enhance overall supply chain efficiency.
What is AutoML?
AutoML, or Automated Machine Learning, simplifies the process of developing machine learning models, making advanced analytics accessible to non-technical users. Traditionally, building predictive models required specialized knowledge in data science and coding. AutoML automates many of these complex steps—such as data preprocessing, feature selection, model training, and hyperparameter tuning—allowing supply chain professionals to generate accurate insights without deep technical expertise.
Key Benefits of AutoML:
- Speed: Rapidly builds and deploys machine learning models, reducing time-to-insight.
- Scalability: Handles large volumes of supply chain data and adapts to dynamic market conditions.
- Accuracy: Continuously refines models for higher prediction accuracy as new data becomes available.
Why Combine Them?
The true power of predictive analytics is unlocked when combined with AutoML. Together, they create a seamless framework for:
- Real-Time Decision-Making: Businesses can respond proactively to emerging risks and market shifts.
- End-to-End Supply Chain Visibility: Gain comprehensive insights across procurement, production, logistics, and distribution.
- Risk Mitigation: Predict potential disruptions and implement preventive measures.
- Cost Optimization: Improve demand planning, reduce waste, and minimize stockouts or overstocking.
By merging the predictive capabilities of advanced analytics with the accessibility of AutoML, businesses can transform supply chain operations, ensuring greater resilience, agility, and efficiency.
Common Supply Chain Disruptions and Their Impact
Supply chains are intricate networks that rely on seamless coordination between suppliers, manufacturers, logistics providers, and retailers. However, even minor disruptions at any point in the chain can cause significant operational challenges. Understanding the most common sources of these disruptions and their business impact is crucial for developing effective mitigation strategies.
Top Disruption Sources:
- Demand Fluctuations:
Rapid changes in consumer demand, seasonal variations, or market trends can lead to inaccurate forecasting. This often results in stockouts or overstocking, directly affecting revenue and customer satisfaction. - Supplier Delays:
Supplier issues—such as production halts, raw material shortages, or geopolitical factors—can delay the flow of essential components, causing downstream disruptions in manufacturing and delivery schedules. - Inventory Stockouts:
Poor inventory management and forecasting inaccuracies can lead to stockouts, resulting in lost sales and damaged customer relationships. On the other hand, overstocking increases warehousing costs and ties up capital. - Transportation Bottlenecks:
Delays in logistics—caused by traffic congestion, customs hold-ups, or extreme weather—can disrupt delivery timelines. Inefficient routing and last-mile delivery challenges can further exacerbate these delays.
Business Impact:
- Increased Operational Costs:
Disruptions often necessitate emergency shipping, expedited labor, or sourcing from alternative suppliers—driving up operational expenses. - Missed Delivery Deadlines:
Delays in production or transportation lead to late deliveries, which can damage customer trust and brand reputation. - Decreased Customer Satisfaction:
Inconsistent product availability and delayed shipments frustrate customers, leading to potential revenue loss and decreased brand loyalty. - Revenue Losses and Competitive Disadvantage:
Companies unable to meet demand or fulfill orders on time risk losing market share to competitors with more resilient supply chains.
Traditional supply chain management approaches struggle to predict and mitigate these disruptions effectively. This is where Predictive Analytics with AutoML becomes a game-changer—enabling businesses to proactively identify risks and optimize supply chain operations for greater resilience.
In the next section, we’ll explore how AutoML helps reduce supply chain disruptions and drives measurable improvements in efficiency and cost savings.
How AutoML Reduces Supply Chain Disruptions
Automated Machine Learning (AutoML) is transforming the way supply chains operate by enabling businesses to harness complex data and turn it into actionable insights—without the need for extensive data science expertise. By integrating AutoML into supply chain operations, organizations can predict disruptions before they happen, optimize decision-making, and improve overall efficiency. Here’s how AutoML helps reduce supply chain disruptions by up to 35%:
1. Data Integration: Building a Unified View
AutoML excels at processing and analyzing vast amounts of data from multiple sources. In supply chain management, this means integrating data from:
- Sales records for demand forecasting
- Inventory levels to prevent stockouts or overstocking
- Supplier schedules to anticipate potential delays
- Logistics and transportation data for real-time tracking and delivery optimization
By consolidating these datasets into a single view, AutoML creates a comprehensive picture of the supply chain, enabling better coordination and more informed decision-making.
2. Pattern Recognition: Spotting Disruptions Before They Happen
One of AutoML’s most valuable capabilities is its ability to identify patterns and anomalies within large datasets. By analyzing historical data and real-time inputs, AutoML can:
- Detect trends that precede disruptions, such as recurring delays from a specific supplier or seasonal spikes in demand
- Recognize inefficiencies in transportation routes and delivery schedules
- Identify correlations between external factors (like weather patterns or geopolitical events) and supply chain delays
This proactive approach allows businesses to predict potential issues and act before disruptions occur.
3. Demand Forecasting: Achieving Greater Accuracy
Accurate demand forecasting is crucial for maintaining optimal inventory levels and ensuring timely fulfillment. AutoML enhances traditional forecasting methods by:
- Using historical sales data, market trends, and customer behavior to create precise demand predictions
- Continuously updating forecasts based on real-time data, ensuring adaptability to sudden market changes
- Reducing the risks of stockouts or overstocking, directly impacting operational costs and customer satisfaction
With more accurate forecasts, businesses can optimize inventory management, improve production planning, and better align supply with demand.
4. Risk Mitigation Strategies: Data-Driven Solutions
AutoML not only predicts potential disruptions but also suggests actionable strategies to mitigate them. Based on predictive insights, businesses can:
- Reallocate inventory to high-demand regions before shortages occur
- Adjust procurement schedules to account for potential supplier delays
- Optimize transportation routes to avoid bottlenecks and reduce delivery times
- Implement contingency plans for high-risk scenarios, such as extreme weather events or supply chain strikes
This proactive risk management approach minimizes downtime, reduces operational costs, and ensures smoother supply chain operations.
5. Real-World Example: Reducing Stockouts by 35%
Imagine a global electronics manufacturer struggling with frequent stockouts during peak sales seasons. By implementing AutoML-driven predictive analytics, the company integrated data from sales, inventory, and supplier systems. The AutoML model identified recurring patterns of demand surges and pinpointed specific suppliers prone to delays. With these insights, the company adjusted its inventory strategy and optimized supplier schedules—resulting in a 35% reduction in stockouts and a significant boost in customer satisfaction.
AutoML enables businesses to move from reactive problem-solving to proactive risk management, transforming supply chain operations and delivering measurable improvements.
In the next section, we’ll dive into the key benefits businesses can expect when integrating AutoML into their supply chain analytics.
Key Benefits of AutoML in Supply Chain Analytics
Integrating AutoML (Automated Machine Learning) into supply chain analytics offers a transformative approach to managing complexity, reducing risks, and optimizing operations. By automating data-driven decision-making, businesses gain a competitive edge in an increasingly volatile market. Here are the key benefits of leveraging AutoML in supply chain management:
1. Improved Forecast Accuracy
AutoML refines demand forecasting by analyzing vast amounts of historical data, market trends, and real-time inputs. This results in:
- Precise demand predictions that reduce stockouts and overstocking
- Better production planning aligned with customer needs
- Optimized inventory levels to improve order fulfillment rates
With improved forecast accuracy, businesses can proactively manage resources, minimize waste, and enhance customer satisfaction.
2. Faster Decision-Making with Real-Time Insights
AutoML continuously processes and analyzes real-time data, enabling supply chain teams to:
- Identify and respond to disruptions as they happen
- Optimize logistics routes based on current traffic, weather, and shipping data
- Make agile adjustments to inventory, procurement, and delivery plans
This real-time visibility reduces delays and enhances the responsiveness of supply chain operations.
3. Reduced Operational Costs
By predicting disruptions and inefficiencies before they occur, AutoML helps businesses minimize unnecessary expenses:
- Lower inventory carrying costs through accurate demand forecasting
- Reduced emergency shipping fees by planning ahead for potential delays
- Optimized workforce allocation by aligning labor needs with actual demand
The result is a leaner, more cost-effective supply chain that maximizes profitability.
4. Enhanced Supply Chain Agility
In today’s dynamic markets, agility is essential. AutoML equips businesses with the flexibility to adapt quickly to changing conditions:
- Rapid scenario modeling allows supply chain managers to test responses to potential disruptions
- Dynamic routing and scheduling enable faster adjustments to logistics and distribution plans
- Improved supplier management through risk assessments and performance tracking
This agility ensures that supply chains remain resilient in the face of market volatility and unexpected disruptions.
5. Scalability Across Global Operations
AutoML’s ability to process massive datasets and adapt to varying market conditions makes it ideal for global supply chains:
- Unified data analysis across regions, suppliers, and distribution networks
- Standardized processes that streamline operations across multiple locations
- Localized insights that optimize supply chain strategies for specific markets
As businesses expand their operations globally, AutoML ensures that supply chain strategies remain efficient, cohesive, and adaptable.
Steps to Implement AutoML in Your Supply Chain
Adopting AutoML (Automated Machine Learning) in your supply chain can significantly improve efficiency, reduce disruptions, and optimize operations. However, successful implementation requires a structured approach to ensure that predictive models align with your business goals and supply chain processes. Here’s a step-by-step guide to help you integrate AutoML into your supply chain operations effectively:
✅ Step 1: Identify Critical Data Sources
The success of any AutoML-driven system depends on the quality and diversity of data it analyzes. Start by identifying and consolidating key data sources across your supply chain:
- Sales Data: Historical sales records, seasonal trends, and customer behavior.
- Inventory Data: Stock levels, turnover rates, and warehouse operations.
- Supplier Data: Delivery timelines, reliability, and historical performance.
- Logistics and Transportation Data: Shipping routes, delivery schedules, and real-time tracking.
- External Factors: Market trends, weather forecasts, geopolitical risks, and economic indicators.
Tip: Ensure data consistency, accuracy, and proper formatting before feeding it into AutoML models.
✅ Step 2: Choose the Right AutoML Platform
Not all AutoML platforms are created equal. Select a platform that aligns with your technical capabilities, budget, and business needs. Consider the following when making your choice:
- User-Friendliness: Intuitive interfaces that don’t require deep data science expertise.
- Integration Capabilities: Seamless connectivity with existing ERP, CRM, and supply chain management systems.
- Scalability: Ability to handle large data volumes and complex supply chain networks.
- Customization: Flexibility to tailor models based on unique business requirements.
Popular AutoML Platforms: Google Cloud AutoML, Amazon SageMaker Autopilot, Microsoft Azure AutoML, and H2O.ai.
✅ Step 3: Train Models Using Historical Data
Once your data is integrated and your platform is set up, it’s time to train your AutoML models. The goal is to help the system identify patterns and correlations that can be used to predict future outcomes.
- Load Historical Data: Supply the model with data from previous sales cycles, past disruptions, inventory movements, and supplier performance.
- Define Objectives: Determine what you want the model to predict—such as demand surges, delivery delays, or stockouts.
- Allow AutoML to Optimize: The system will automatically select algorithms, fine-tune parameters, and evaluate model accuracy.
Tip: Use cross-validation techniques to ensure the model’s reliability and reduce overfitting.
✅ Step 4: Integrate Predictions into Supply Chain Workflows
For AutoML to drive real impact, its insights need to be embedded into your day-to-day supply chain operations.
- Forecast Demand: Use predictive insights for accurate production planning and inventory management.
- Optimize Logistics: Improve route planning, shipping schedules, and last-mile delivery efficiency.
- Mitigate Risks: Identify potential disruptions early and develop contingency plans.
- Enhance Supplier Collaboration: Share data-driven forecasts with suppliers to ensure better coordination and reduce lead times.
Pro Tip: Implement dashboards and reporting tools that make it easy for supply chain teams to act on predictive insights.
✅ Step 5: Continuously Monitor and Refine Models
Supply chains are dynamic, and predictive models need regular updates to remain effective. Continuously monitor your AutoML system’s performance and refine models based on new data.
- Track Key Performance Indicators (KPIs): Monitor forecast accuracy, stockout rates, on-time deliveries, and cost reductions.
- Retrain Models with New Data: Regularly update the system with real-time and historical data to improve accuracy.
- Incorporate Feedback Loops: Encourage teams to provide feedback on predictions and integrate their insights into model improvements.
Remember: The more data the model processes, the smarter and more accurate it becomes over time.
Measuring Success: KPIs to Track
Implementing AutoML (Automated Machine Learning) in your supply chain is a strategic move, but its true value lies in measurable outcomes. To evaluate the effectiveness of your AutoML initiatives and ensure they deliver tangible business results, it’s crucial to track key performance indicators (KPIs). These metrics provide insights into operational efficiency, cost savings, and overall ROI.
Here are the essential KPIs to monitor when measuring the success of AutoML in your supply chain:
📉 1. Reduction in Supply Chain Disruptions
Why it matters: The primary goal of predictive analytics is to minimize supply chain disruptions, which directly affect costs, efficiency, and customer satisfaction.
How to measure it:
- Track the frequency and severity of disruptions before and after AutoML implementation.
- Monitor unplanned delays, production halts, and order fulfillment failures.
Target Outcome:
A noticeable decrease in supply chain interruptions, leading to smoother operations and reduced crisis management costs.
📈 2. Forecast Accuracy Improvement
Why it matters: Accurate demand forecasting ensures optimal inventory levels, efficient production planning, and timely order fulfillment.
How to measure it:
- Compare historical forecast accuracy with post-AutoML accuracy rates.
- Use metrics like Mean Absolute Percentage Error (MAPE) and Root Mean Square Error (RMSE) to evaluate prediction precision.
Target Outcome:
Improved demand forecasts that lead to fewer stockouts, overstocking incidents, and production inefficiencies.
💰 3. Decrease in Inventory Carrying Costs
Why it matters: Poor inventory management leads to high storage costs, product obsolescence, and cash flow constraints.
How to measure it:
- Calculate inventory carrying costs as a percentage of total inventory value.
- Track warehouse space utilization, stock turnover rates, and average holding periods.
Target Outcome:
Optimized inventory levels that reduce storage expenses and free up working capital.
🚚 4. Increase in On-Time Deliveries
Why it matters: Timely delivery is a critical factor in customer satisfaction and brand reputation.
How to measure it:
- Measure the On-Time Delivery Rate (OTD)—the percentage of orders delivered on or before the promised date.
- Track shipping delays, last-mile delivery issues, and customer feedback related to delivery times.
Target Outcome:
Higher on-time delivery rates, leading to improved customer satisfaction and increased repeat business.
💵 5. ROI from AutoML Investments
Why it matters: To justify the investment in AutoML, it’s essential to evaluate the financial return it generates.
How to measure it:
- Calculate the Return on Investment (ROI) by comparing cost savings and revenue increases against the initial implementation and ongoing operational costs of AutoML.
- Factor in cost reductions from fewer disruptions, lower inventory carrying costs, and improved operational efficiency.
Target Outcome:
A positive ROI within a reasonable time frame, demonstrating the long-term value of AutoML in optimizing supply chain operations.
Bringing It All Together
Tracking these KPIs ensures that your AutoML-driven supply chain strategies are aligned with business goals and continuously optimized for better performance. Regularly reviewing these metrics helps identify improvement areas, fine-tune predictive models, and maximize the overall impact of AutoML on your supply chain.
In the final section, we’ll wrap up with key takeaways and how your business can start its journey toward a more resilient, data-driven supply chain.
Conclusion
In today’s fast-paced global market, supply chain disruptions can lead to significant financial losses, operational inefficiencies, and diminished customer satisfaction. Traditional management methods often fall short in navigating these complexities. Predictive Analytics powered by AutoML offers a forward-thinking solution—enabling businesses to transition from reactive problem-solving to proactive risk management.
By leveraging AutoML, businesses gain the ability to:
- Reduce supply chain disruptions by up to 35%
- Enhance forecast accuracy for better inventory management
- Decrease operational costs through optimized logistics and planning
- Boost supply chain agility, enabling faster responses to market changes
- Increase on-time deliveries and improve overall customer satisfaction
With the power of predictive analytics and AutoML, supply chains become smarter, more resilient, and better equipped to handle uncertainty—driving operational excellence and competitive advantage.
At Datahub Analytics, we specialize in helping businesses unlock the full potential of their data to create smarter, more resilient supply chains. Our Predictive Analytics and AutoML solutions empower you to anticipate disruptions, optimize operations, and achieve measurable results.
🎯 Reduce disruptions. Optimize costs. Improve efficiency.
Let us help you build a supply chain that’s agile, data-driven, and future-proof.