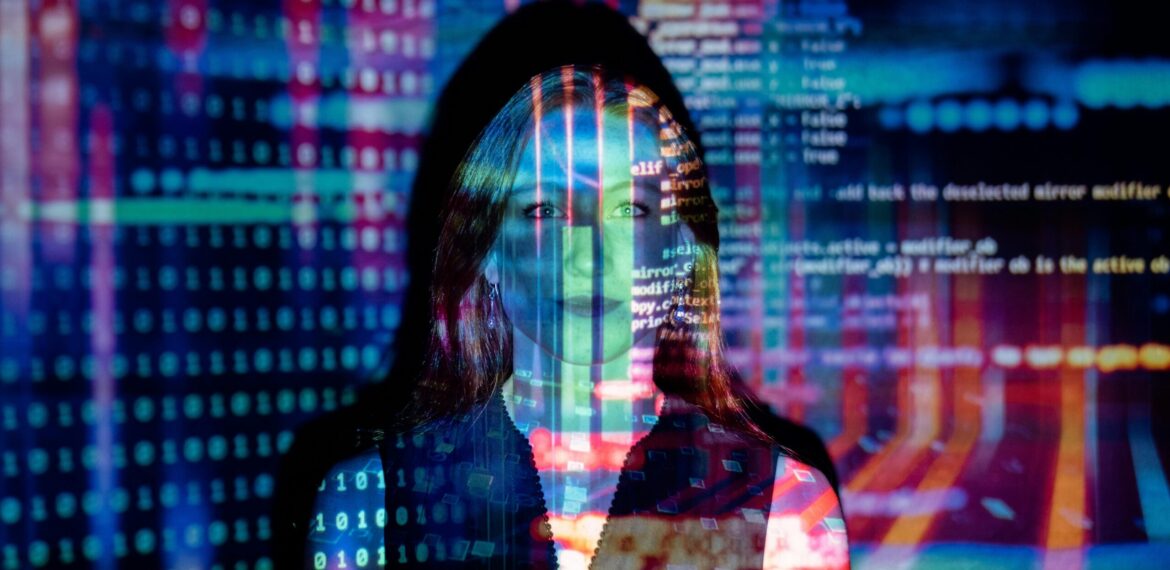
Types of Simulation Models in Data Analytics
Types of Simulation Models in Data Analytics
Simulation models are becoming a popular way for businesses to understand trends and their impact. Simulations mean replicating the conditions of the real world in an isolated setting to understand how certain variables react when changed. Simulation models tend to do the same work, becoming an important part of data analytics.
Simulation models help one understand how new rules and conditions may impact situations in the real world. Businesses are investing in these models to understand how certain situations can impact the economy and how specific trends can help them gain more profitability. There are four types of simulation models that are used in data analytics. In this blog, we will be diving deep into each of them to understand how they can benefit your business.
4 Types of Simulation Models in Data Analytics
Simulation models can be very helpful in predicting how a business will fare in different situations. When combined with data analytics, it becomes a great tool that all organisations must leverage to get insights that are similar to those of the real world. Let’s examine the four types of simulation models and how they work. Scroll down to read more about them!
Monte Carlo Method
This method is also known as the risk assessment model. This model is based highly on mathematical principles and is based on the roulette game that became popular in Monte Carlo resorts. Businesses use this type of simulation method before implementing any major changes in their policies or business operations. In a roulette game, once you spin, you can get any random value between 0 and 36. While there can be many variables that may result in wrong calculations when you spin the wheel, suppose, say, 1000 times, the actual method of simulation is used for risk assessment using empirical data.
Through this method, you can gain knowledge about potential threats and issues on your bottom line when implementing a change that is considered risky. This type of model is also used by political analysts to take into consideration the many variables that can create potential challenges or risks.
Agent-based Modeling
Agent-based models are a type of simulation that depends heavily on agents. These agents can be anything from the immediate environment to people. However, what is important to note about these agents is that they also produce a pattern. The pattern made by them is created keeping in mind certain rules, which are often discrete and not explicitly dictated. It is the knowledge one has already acquired by one means or another.
Agent-based systems similarly simulate agents and make sure they follow the rules that are already in place. Through this model, one can check how a certain agent acts when there are anomalies. This model is one of the best when it comes to assessing risks. Through this model, one can understand how agents will act out in different situations and plan for them accordingly.
Discrete Event Simulation
Your business can benefit greatly from discrete event simulation as it can help you get rid of bottlenecks in your work processes. This type of model can improve the efficiency of your processes and improve operational efficiency. This model helps in understanding how certain processes work and how changes in the process can impact it.
This method breaks down time into unique steps so it is no longer continuous. It uses explicit programming to create stable or quasi-stable components. Each step of the process can be broken down to understand how it is related to the next and previous steps. This type of model is used in a number of industries to understand how certain processes work and can be optimised.
System Dynamic Modeling
This type of simulation model is abstract and does not take into account any specific agent or process. This type of model is used more for long-term strategy and planning of business operations and processes. System dynamic modelling is designed specifically to study and understand situations that are chaotic in nature. When there are too many agents that are high in density, this model can create simulations of the system to offer what kind of structures can be created when the original ones are disrupted.
Businesses can create alternative courses of action in case of adversaries by using such methods. It can offer insights into aggregate levels to create a system in response to any variables.
To Sum Up
Simulation models used in data analytics have the potential to move your business towards profitability and growth. Using data with simulations allows you to create plans for unforeseen scenarios. Simulation models allow you to make the most of the data you have by providing you with real-life scenarios and how certain patterns produce certain results. So, ensure that you combine simulation models with data analytics to make the most of the technologies available to you for your growth and expansion.